Figures & data
Figure 2. The study areas Lixin County and Yongqiao District in Anhui Province, China: (a) shows topography of Anhui Province by digital elevation model (DEM), (b) and (c) show spatial distributions of poplar species with sample plots in Yongqiao and Lixin, respectively.
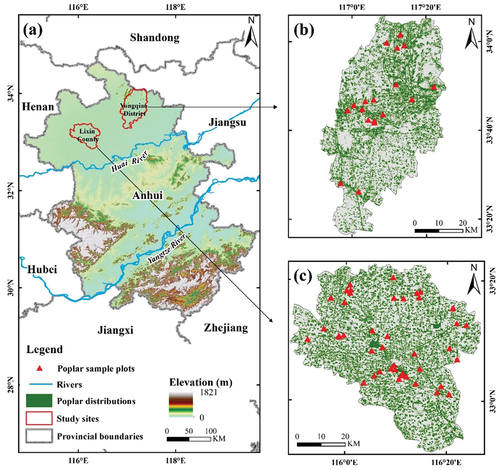
Figure 3. Framework for developing poplar forest growing stock volume (GSV) estimation models based on airborne LIDAR and Gaofen-6 data.
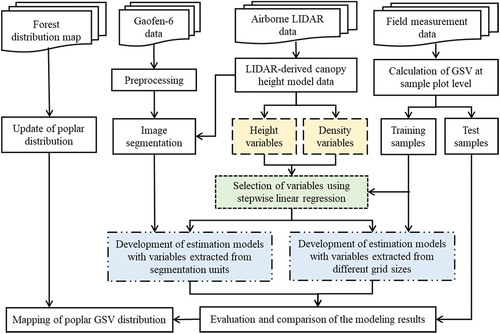
Table 1. The datasets used in this study.
Table 2. The DBH and GSV statistics of poplar sample plots.
Table 3. Poplar GSV estimation models using LIDAR CHM-derived variables at a plot size of 26 m × 26 m.
Figure 4. Relationships between the predicted poplar growing stock volume using developed models and observed values of sample plots, including the scatter plots in Lixin which the models were developed using canopy height variables (a1) and a combination of canopy height variables and density variables (a2); the scatter plot in Yongqiao which the model was developed using canopy height variables only (b).
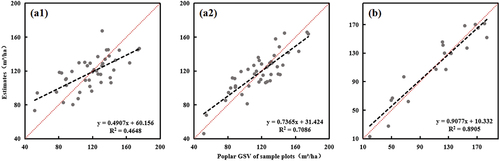
Table 4. Summary of GSV estimation accuracy assessment results based on different scenarios.
Figure 5. Relationships between observed poplar GSV of sample plots and estimated GSV based on different scenarios: (a1) to (a4) represent the statistical units at which the variables were extracted 10 m × 10 m, 15 m × 15 m, 20 m × 20 m, and segment, respectively in Lixin, (b1) to (b4) represent the same statistical units in Yongqiao.
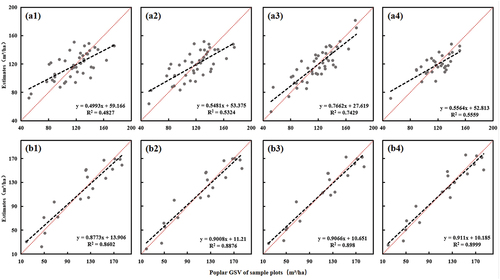
Figure 6. The growing stock volume estimates at segments (a) and (c) and grid sizes of 20 m × 20 m (b) and (d) in Lixin and Yongqiao ((a) and (b) for Lixin; (c) and (d) for Yongqiao).
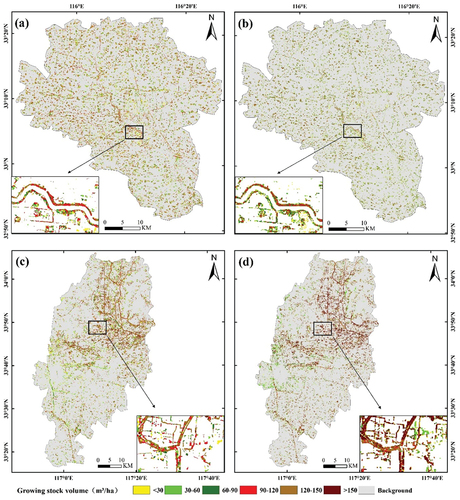
Figure 7. Poplar growing stock volume estimates of typical poplar strip forests from grid-based and object-based approaches: (a1) and (a2) represent grid-based estimates based on grid size of 20 m × 20 m and object-based estimates in Lixin, (b1) and (b2) represent grid-based object-based estimates in Yongqiao.
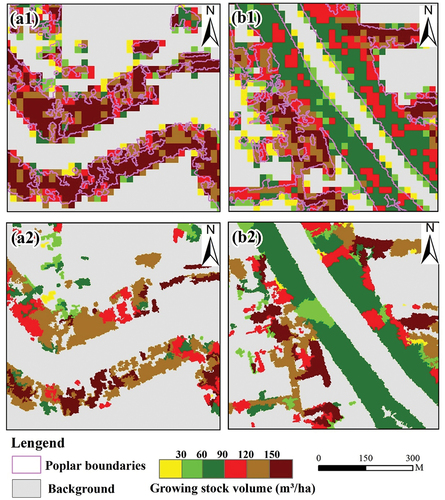
Table 5. A comparison of estimated total GSV amounts in both study areas.
Figure 8. A comparison of scatter plots based on the GSV estimates from grid-based (20 m × 20 m) and object-based modeling approaches in Lixin ((a), (b) are based on the combination of CHM metrics and density variables; (c), (d) are based on CHM metrics only; (a), (c) include fully covered poplar plots while b, d include partially covered poplar plots) and Yongqiao ((e) means fully covered poplar plots, (f) means partially covered poplar plots).
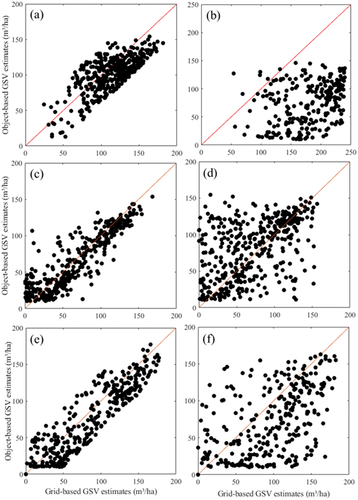
Data availability statement
Due to confidentiality agreements, supporting data can only be made available to bona fide researchers subject to a nondisclosure agreement. Details of the data and how to request access are available from Professor Dengsheng Lu at Fujian Normal University.