Figures & data
Table 1. Details of the five road datasets.
Figure 4. The flowchart of the proposed pseudo-label guided global-scale road extraction framework, which is composed of two parts: (a) GRSet generation, and (b) pseudo-label guided global-scale road extraction network.
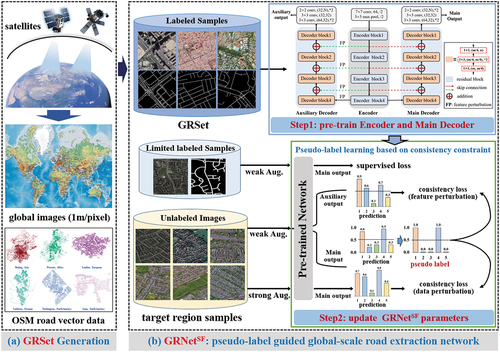
Table
Figure 6. Geographical locations of the validation sets. The validation set covers the four continents of Europe, Africa, Asia, and North America.
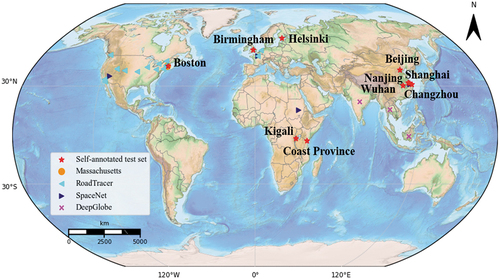
Table 2. The details of the validation set of global road networks.
Table 3. Initialization details of the comparison methods.
Figure 9. The visual outputs for the Birmingham image produced by LinkNet50_IN with different training sets.
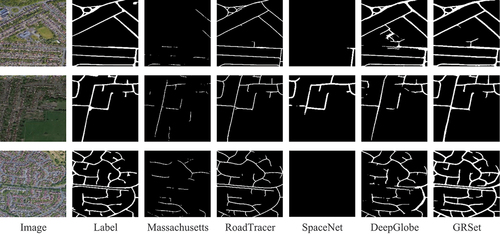
Figure 10. The histogram illustrates the quantitative results obtained on the SpaceNet and DeepGlobe dataset.
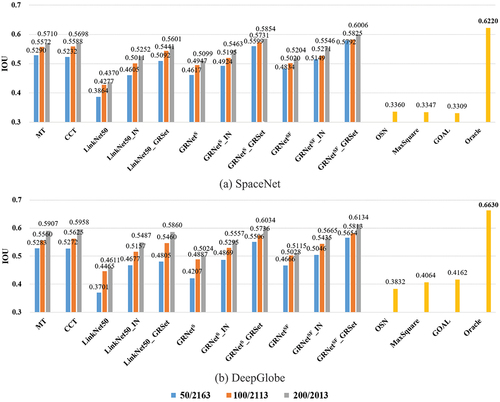
Figure 11. The visualization results of different road datasets, where the first and second rows respectively represent the results obtained on the SpaceNet and DeepGlobe datasets.
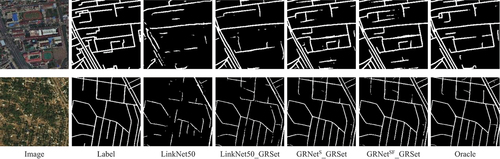
Data availability statement
The data that support the findings of this study are available at https://github.com/xiaoyan07/GRNet_GRSet from the author [X. Lu] and the corresponding author [Y. Zhong] ([email protected]), upon reasonable request.