Figures & data
Figure 1. (a): Location of study area with land use and land cover classification. (b): Distribution pattern of DBF (c): Proportion of landscape. DBF: deciduous broadleaf forest, DNF: deciduous needleleaf forest, EBF: evergreen broadleaf forest, ENF: evergreen needleleaf forest. (d): SRTM DEM. (e): PALSAR-2 L-band SAR image displayed as RGB = HV, HH, VH in db. (f): Sentinel-1 C-band SAR image displayed as RGB = VV, VH, VV in db. (g): Sentinel-2 MSI image in true color composite (RGB = Red, Green, Blue).
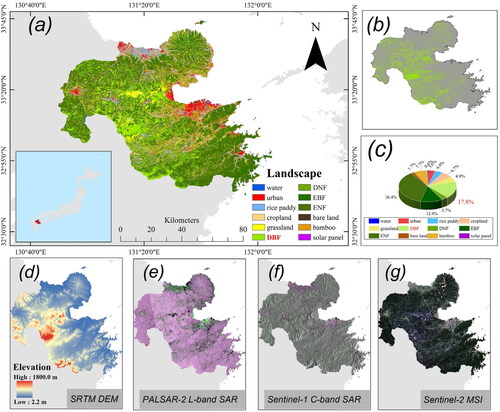
Figure 3. Airborne LiDAR Data. (a) example of modelling data, (b) close-up of airborne LiDAR plots, (c) Oita prefecture.
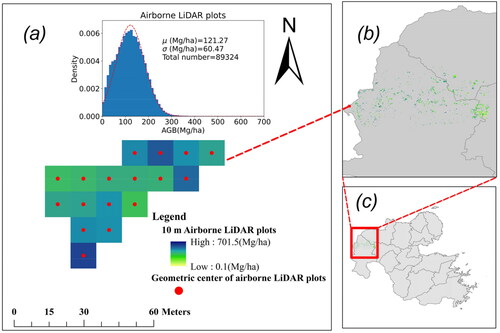
Table 1. Summarize of satellite derived variables.
Table 2. Summarize of regression models.
Figure 4. Result of PCA, the selected bands are pointed in red. (a): Sentinel-2 Multispectral Bands. (b): PALSAR-2 Polarized Channels.
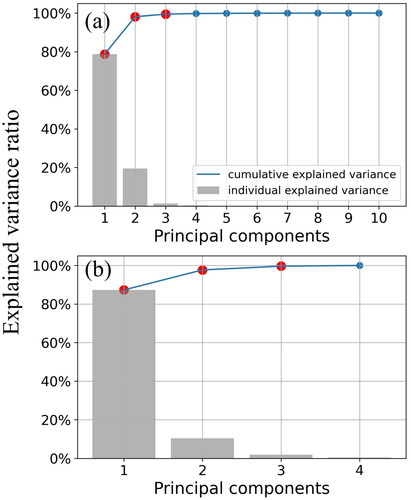
Figure 7. Observed and predicted AGBs for testing samples: (a) PCA-model, (b) VI-model, (c) Band-model, (d) All data; The 1:1 line is marked by the dashed black lines.
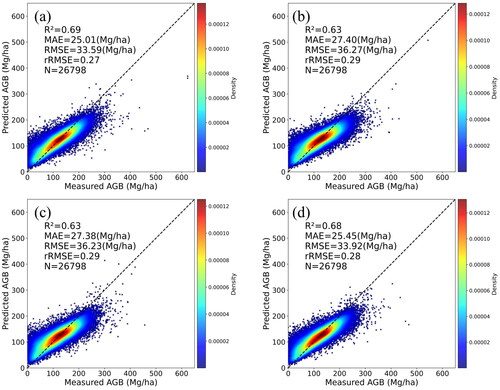
Figure 8. Variables listed in order of importance based on Gini importance: (a) PCA-model, (b): VI-model, (c): Band-model, (d): All data; abbreviation: S2: Sentinel-2, S1: Sentinel-1, P: PALSAR-2, others in .
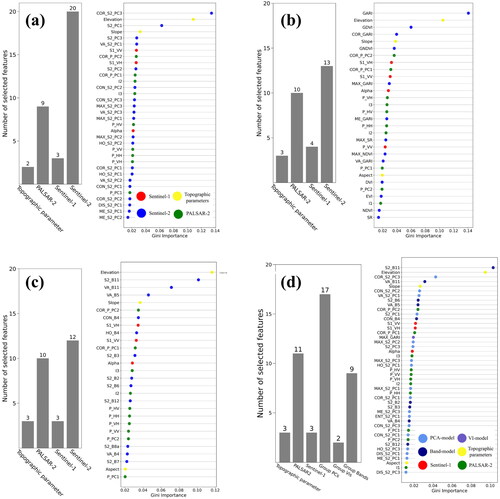
Figure 9. (a) Estimated wall-to-wall AGB of DBF in Oita prefecture, Japan. (b) Comparison of total DBF aboveground biomass (AGB) between statistical data in the Japanese forest register and the satellite-based model in city level. (c) First group. (d) Second group. Each data point represents the cumulative AGB at each of 18 cities (villages, towns, and cities) in Oita prefecture. is masked in red dotted line. 95% Confidence Interval is drawn in blue area.
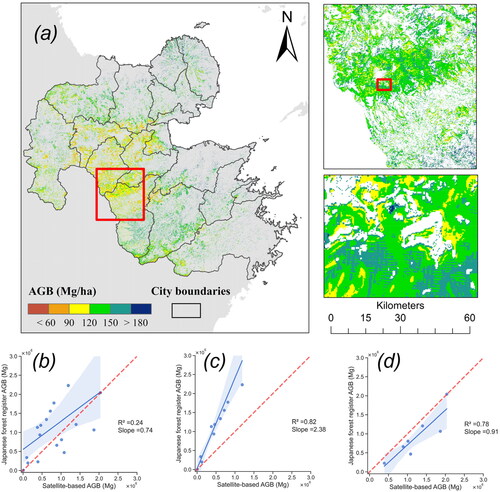
Figure 10. Correlation coefficient matrix between selected features: (a) PCA-model (b) VI-model, (c) Band-model, and (d) All data.
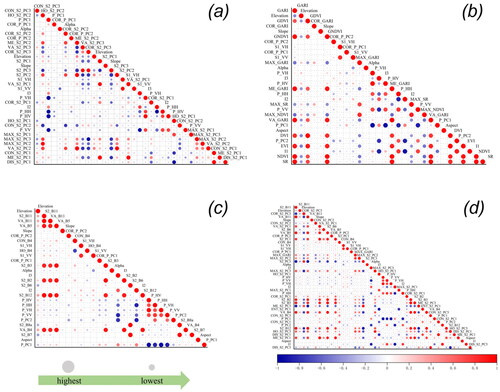
Table 3. Variance inflation factor in each group.
Figure 11. Correlations between individual input bands and output component, (a) PC1 78.2% of dataset variance; (b) PC2 19.4% of dataset variance; (c) PC3, 1.33% of dataset variance; (d) Summarize of PCA proportion.
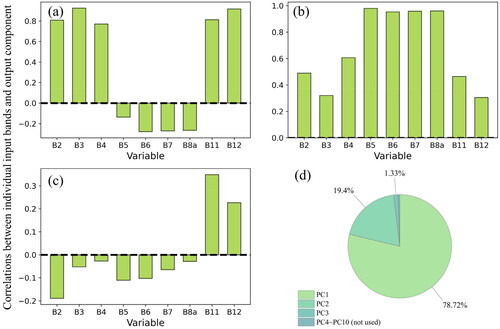
Table Appendix 1. Vegetation indices calculated from Sentinel-2 and PALSAR-2 used in this study.