Figures & data
Figure 1. The forest types and forest AGB data across California; the dot points correspond to forest AGB at a 500 m spatial resolution obtained by aggregating the lidar-derived biomass at a 30 m spatial resolution.
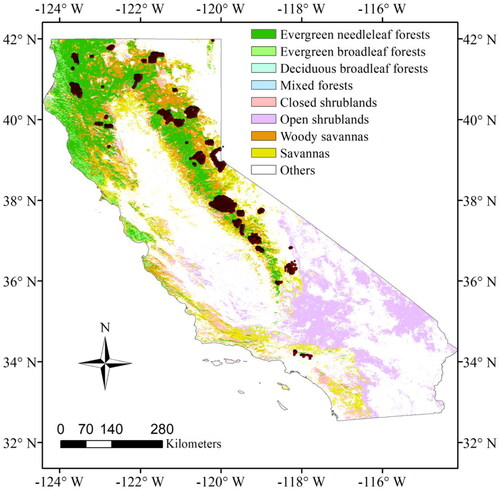
Table 1. The data sets of the MODIS data products used for AGB estimation.
Table 2. The temporal features used for modeling AGB under 18 scenarios.
Figure 2. The accuracy of forest AGB estimated using the annual time-series data under the 18 scenarios; (a), (b), and (c) respectively represent the average R2, RMSE, and bias values achieved by the AGB models developed under the 18 scenarios listed in .
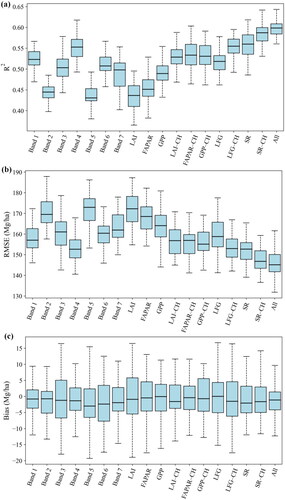
Figure 3. The accuracy of forest AGB estimated using the seasonal time-series data under the 10 scenarios; (a), (b), and (c) respectively represent the seasonal R2, RMSE, and bias values achieved by the AGB models developed under the 18 scenarios; the error bars show the 95% confidence intervals.
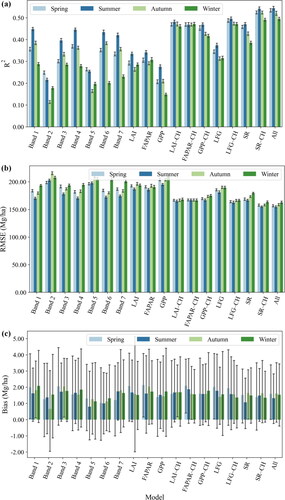
Figure 4. The accuracy of the forest AGB estimated using the temporal features selected by the AAF, SCC, PCP, and SAP methods; (a), (b), and (c) respectively represent R2, RMSE, and bias values achieved under the 18 scenarios; the error bars show the 95% confidence intervals.
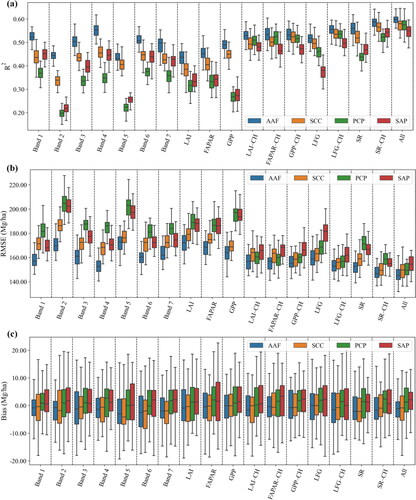
Data availability
The data that support the findings of this study are available on request from the corresponding author.