Figures & data
Table 1. Sample plots distribution and the numbers of gerbil holes.
Table 2. Image object information on brightness, spectral and geometric features.
Figure 3. ESP tool outputs for the UAV imagery Graphs depict changes in local variance (LV) (solid black) and rate of change (ROC) (solid blue) with increasing scale parameter.
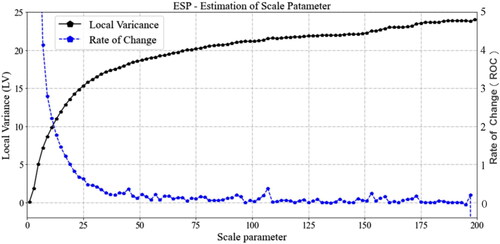
Table 3. Correlation-based forward search process.
Figure 8. Classification results of UAV imagery based on spectral features, texture features and geometric features.
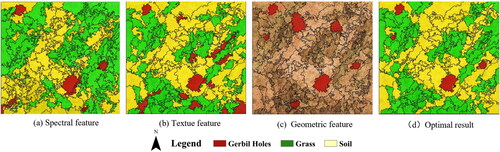
Table 4. Recommended parameters for identifying effective gerbil holes in OBIA.
Figure 10. UAV imagery and partly enlarged detail of classification results. (a) UAV imagery of an entire sample plot, (b) Subset of UAV imagery, (c) OBIA result of subset, (d) ML classification result of subset, (e) OBIA with post classification and (f) MLC with post classification
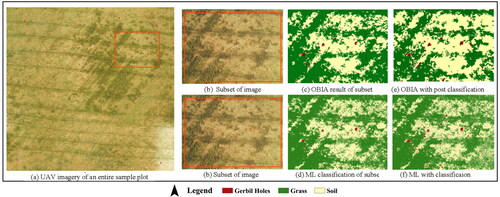
Table 5. Accuracy assessments of two methods.
Data availability statement
The data that support this study was shared on ScienceDB (scidb.cn).