Figures & data
Figure 1. The workflow of the multiscale attention HybridSN integrated with UNet for HSI classification. The model combines the advantages of the multiscale attention HybridSN and the UNet to achieve improved performance in HSI classification.
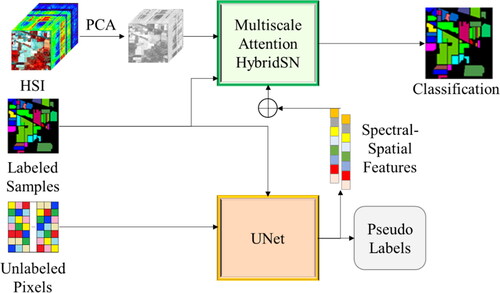
Figure 2. The workflow of the multiscale attention HybridSN model for HSI classification. The model is composed of three sets of 3D convolutional layers, each processing image patches of different scales (5x5, 7x7, and 11x11), and utilizing spectral attention before the 3D convolutional layers to weigh the importance of different spectral bands. The features learned from these 3D convolutional layers are then concatenated and passed through 2D convolutional layers, which employ spatial attention before the 2D convolutional layers to weigh the importance of different spatial locations. Finally, the output is obtained from a fully connected layer with the activation function of softmax.
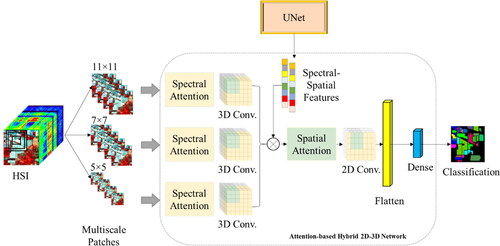
Figure 3. The UNet model architecture for HSI classification. This model consists of a series of 2D convolutional layers and up-sampling layers, with max pooling used to reduce the spatial dimensions. The architecture is symmetrical with a contracting path and an expanding path, which helps to preserve spatial information throughout the model. The final layer includes a dense layer and a softmax activation function to generate class probabilities.
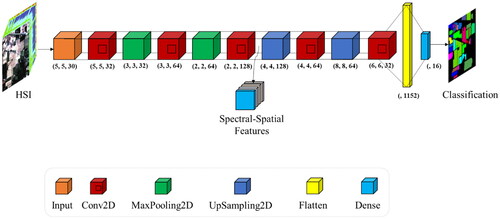
Figure 4. The architecture of spatial attention is used in this research. This component uses a combination of convolutional layers and pooling to learn and highlight the most informative spatial features in the input image.
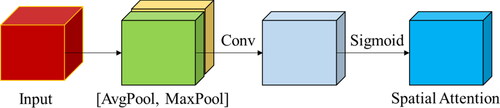
Figure 5. The spectral attention module in the multiscale attention HybridSN model for HSI classification. The module is designed to emphasize the important features in the spectral dimension and weigh them accordingly before the 3D convolutional layers process the input data.
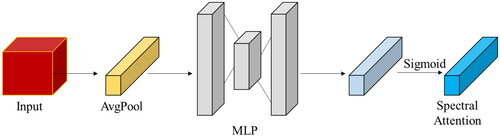
Table 1. The number of training, validation, and testing samples for the Indian Pines dataset.
Table 2. The number of training, validation, and testing samples for the University of Pavia dataset.
Table 3. The number of training, validation, and testing samples for the Houston University dataset.
Figure 7. Classification maps of different models on the IP dataset. (a) Ground-truth map. (b) SVM. (c) 2D CNN. (d) 3D CNN. (e) HybridSN. (f) M3D-DCNN. (g) DBDA. (h) ACA-HybridSN. (i) MSA-HybridSN-U.
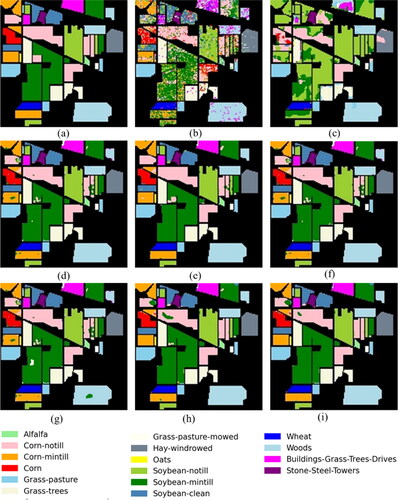
Figure 8. Classification maps of different models on the PU dataset. (a) Ground-truth map. (b) SVM. (c) 2D CNN. (d) 3D CNN. (e) HybridSN. (f) M3D-DCNN. (g) DBDA. (h) ACA-HybridSN. (i) MSA-HybridSN-U.
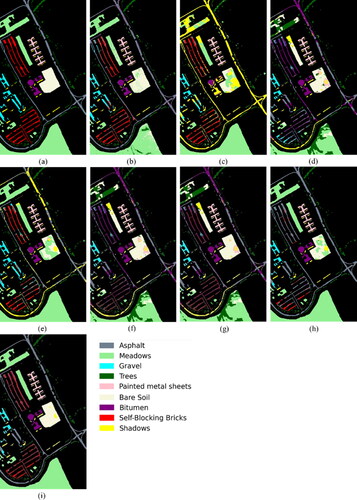
Figure 9. Classification maps of different models on the HU dataset. (a) Ground-truth map. (b) SVM. (c) 2D CNN. (d) 3D CNN. (e) HybridSN. (f) M3D-DCNN. (g) DBDA. (h) ACA-HybridSN. (i) MSA-HybridSN-U.
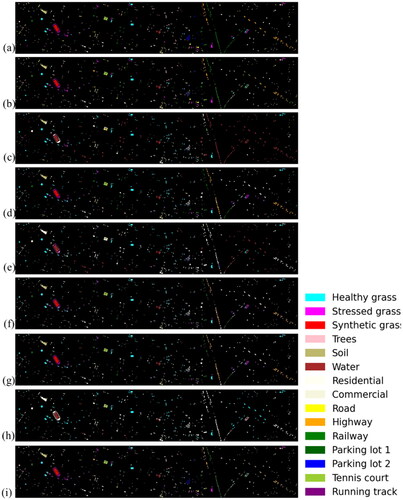
Table 4. Classification results of 5% samples of IP dataset.
Table 5. Classification results of 5% samples of PU dataset.
Table 6. Classification results of 5% samples of the HU dataset.
Table 7. Per class accuracies of 5% samples of IP dataset.
Table 8. Per class accuracies of 5% samples of PU dataset.
Table 9. Per class accuracies of 5% samples of HU dataset.
Figure 11. OA (%) of the proposed model with and without using multiscale features in the three datasets.
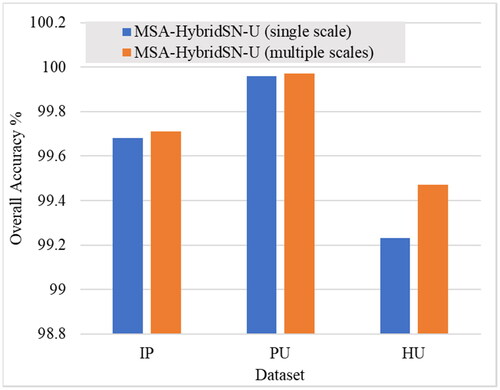
Figure 13. OA (%) of the proposed model with and without unsampled pixel utilization in the three datasets.
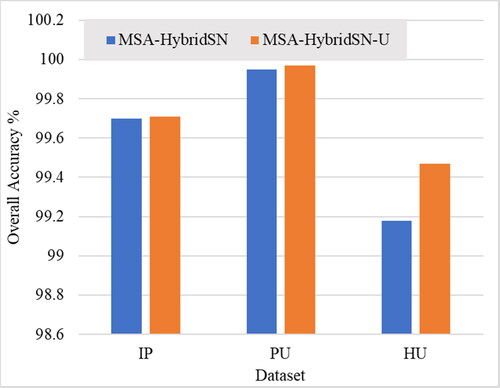
Table 10. Effect of training sample size on the proposed model for hyperspectral image classification.
Table 11. Computational complexity (training time and test time) of the proposed and benchmark models for hyperspectral image classification based on the IP dataset.
Data availability statement
The data that support the findings of this study are available from the corresponding author, Helmi Z. M. Shafri, upon reasonable request.