Figures & data
Table 1. BEMs and top performance models that have been used in GWPM.
Table 2. Data sources and indicators.
Figure 6. Topographic parameters. (a) ELE; (b) SG; (c) ASP; (d) PLC; (e) PRC; (f) TRI; (g) TPI; (h) SL.
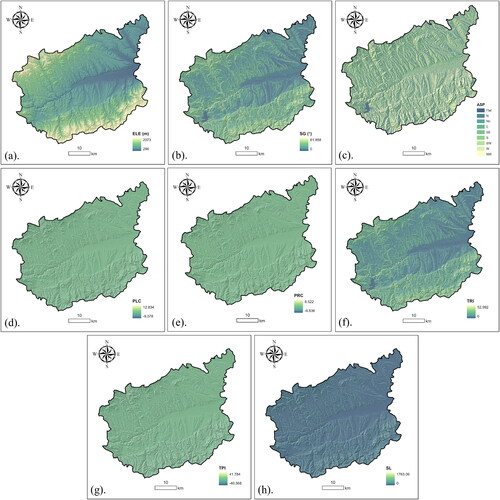
Table 3. Hyperparameter optimization results of different models.
Table 4. Areas of GWP levels in different models.
Table 5. Field validation results.
Figure 9. Model validation results. (a) RF; (b) AdaBoost; (c) GBDT; (d) CatBoost; (e) XGBoost; (f) LightGBM.
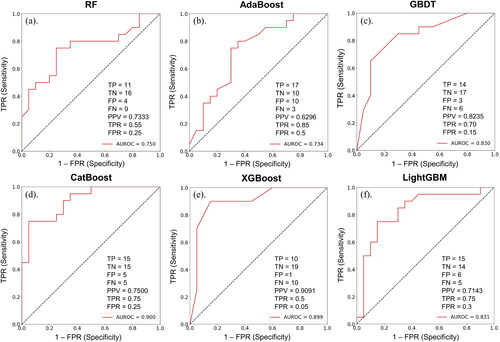
Figure 10. Importance analysis of indicators. (a) RF; (b) AdaBoost; (c) GBDT; (d) CatBoost; (e) XGBoost; (f) LightGBM.
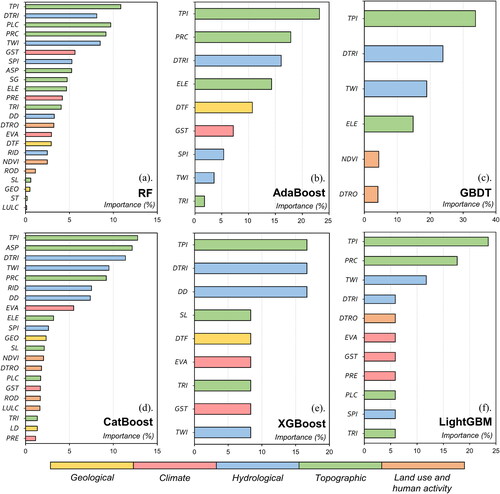
Figure 11. Correlation analysis of important indicators. (a) Random points; (b) Pearson correlation coefficient result; (c) Spearman correlation coefficient result; (d) Kendall correlation coefficient result.
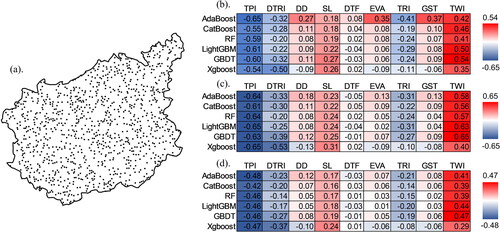
Figure 12. The determination of groundwater management priority levels. (a) Population distribution; (b) Areas for high and very high GWP; (c) The matrix for the combination of population and GWP map; (d) Groundwater management priority levels in Luoning County.
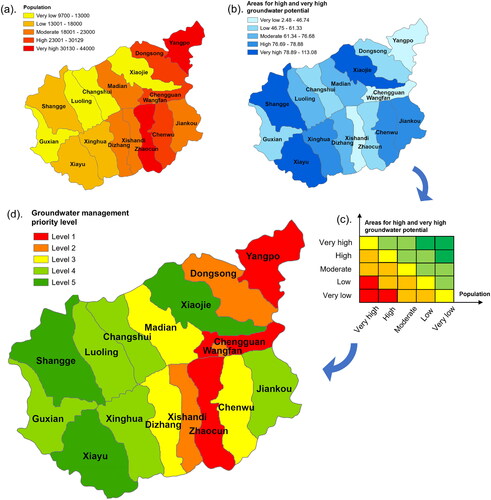
Supplemental Material
Download MS Word (688.2 KB)Data availability
The authors do not have permission to share data.