Figures & data
Table 1. Example of a hypothetical CPT.
Table 2. Example of a hypothetical causal CPT.
Table 3. Some popular Bayesian network (BN) inference algorithms.
Figure 5. Conditional probabilities of AM with (right) and without (left) occupational exposure, for men (top) and women (bottom).
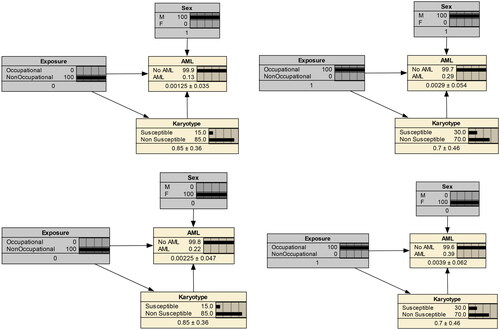
Table 4. Example of qualitative exposure levels defined by the qualitatively different responses elicited.
Table 5. Examples of multipliers and multiplier ranges.
Table 6. Examples of sources of inter-individual variability in epidemiological studies.
Figure 7. Example of a sensitivity analysis showing how the unconditional probability of causation, mean(AS) (which is the same as mean(CAS) if M0 and R are the only direct causes of risk) depends on the assumed probability that exposure is too low to affect the number of malignant cells formed. Source: Code at https://chat.openai.com/share/04aa37d4-ee74-4c8a-ae64-c32e70d7fbeb.
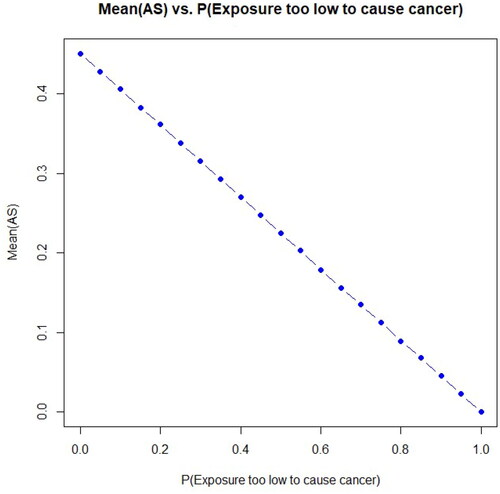
Figure 8. Decomposition of a causal exposure-response function, exposure, x → risk (bottom) into two components: exposure, x → internal dose, y (upper left); and internal dose, y → risk (upper right).
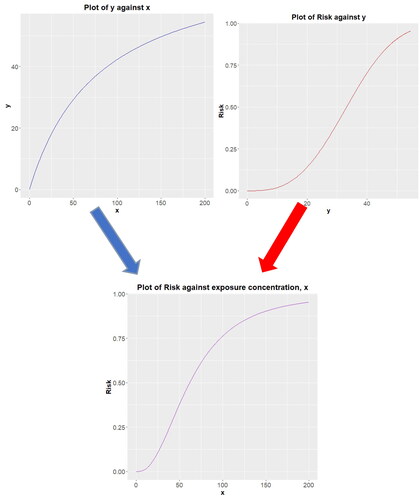
Table 7. Selected causal discovery algorithms for identifying plausible causal models from data.
Figure 9. A “no side effects” conceptualization of proposed causal pathways linking benzene exposure to AML risk. Source: McHale et al. (Citation2012)
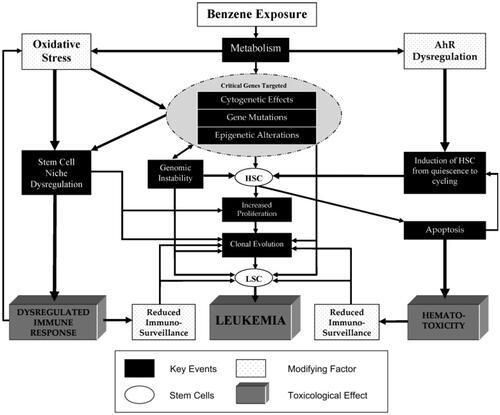
Figure 11. Plausible upper-bound estimates of IPoC as a function of benzene concentration in air. # Source: R code for left side of . PPH = exceedance probability for PH; Y0PH = 69.5; YmaxPH = 5293; X50PH = 107.1; Y0HQ = 6.36; YmaxHQ = 376; X50HQ = 50.9; X <- (0:1000)/20; YHQ <- Y0HQ + YmaxHQ*X/(X50HQ + X); YPH <- Y0PH + YmaxPH*X/(X50PH + X); PHQ <- 1-pnorm(log(68.1), log(YHQ), log(2.24)/1.96); PPH <- 1-pnorm(log(521.5), log(YPH), log(2.24)/1.96); plot(X, PPH*1e-7, ylab = "IPoC", xlab = "benzene ppm"); # plot(X, PPH*0.54, ylab = "CAS", xlab = "benzene ppm");
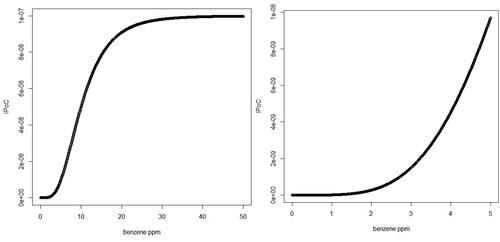
Figure 12. Plausible upper-bound estimates of causal assigned share (CAS) for AML as a function of benzene concentration in air.
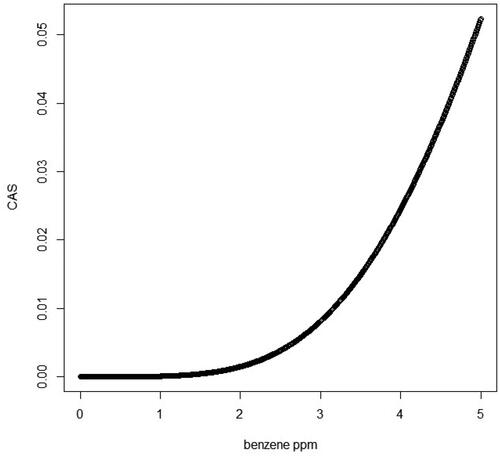