Figures & data
Figure 1. Graphical Representation of the Model to Examine Emotional Synchrony.
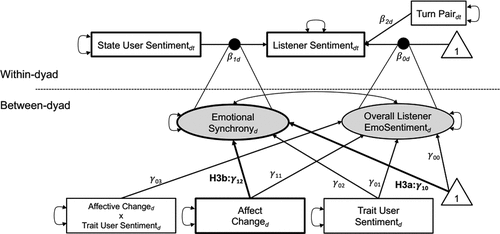
Figure 2. Change in Users’ Affective State from Pre- to Post Conversation.
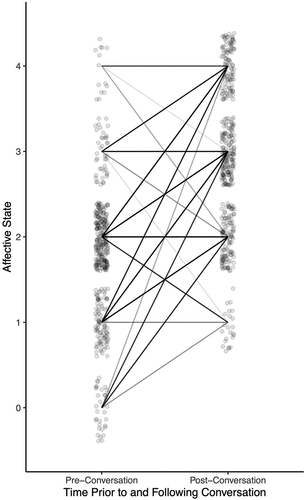
Table 1. Results from regression examining change in users’ affective state.
Figure 3. Results from Regression Model Predicting Affective State Change from Language Style Matching.
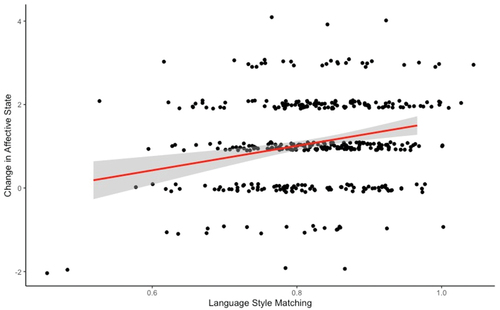
Table 2. Results from regression examining the association between lsm and change in users’ affective state.
Table 3. Results from multilevel model examining the association between users’ and listeners’ emotional sentiment and change in users’ affective state.