Figures & data
Figure 1. Scale estimates of the family income data. The SDO scale is measured symmetrically about the median, whereas the DO scales are not and reflect skewness.
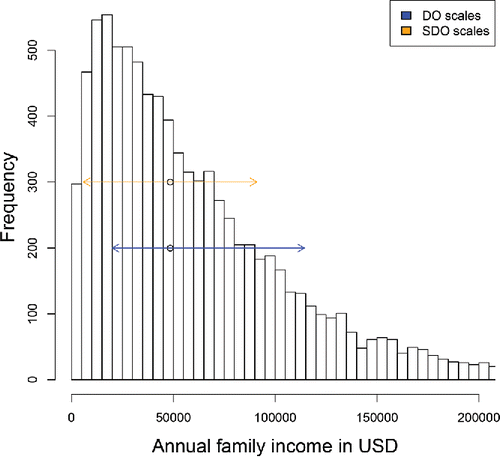
Figure 4. Influence function of DO(x) for F = Φ. Left: 3D, right: 2D seen from above. For a fixed point x it is bounded over all possible positions z of contamination.
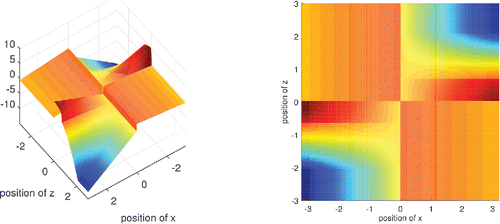
Figure 5. Bloodfat data with (a) SDO contours, and (b) DO contours. The DO contours better reflect the skewness in the data.
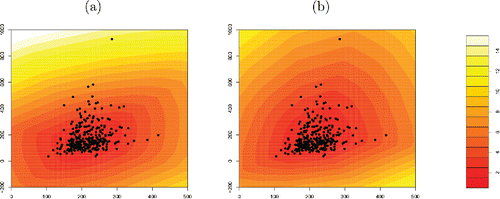
Figure 7. Functional DO (fDO) values of the 180 glass spectra. Higher fDO values correspond to curves that are more outlying on average.
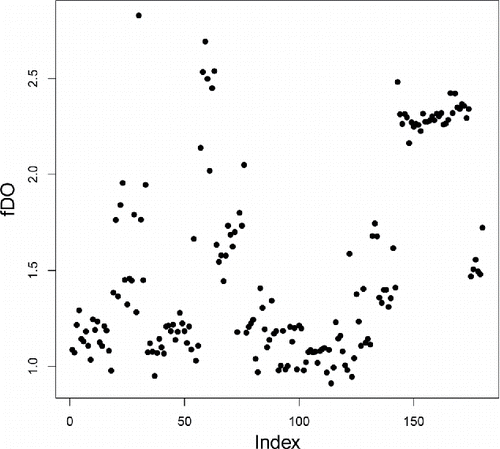
Figure 9. Outlier cutoffs for the family income data. The DO-based cutoff takes the data skewness into account.
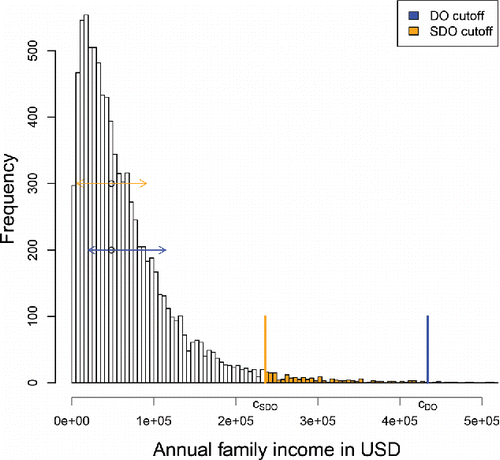
Figure 10. Outlier detection on bloodfat data. The DO-based cutoff adapts to the data skewness and flags fewer points as outlying.
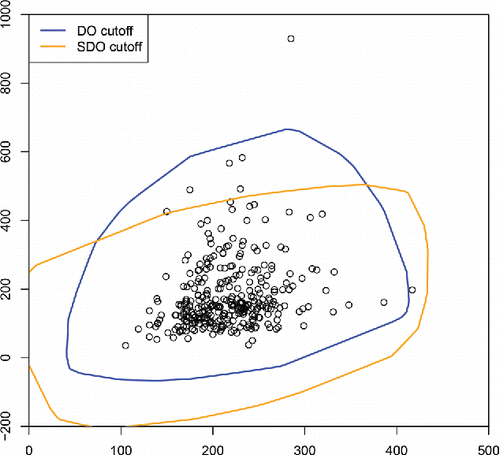
Figure 12. Original MRI image of subject 387, and its derivatives in the horizontal and vertical direction.
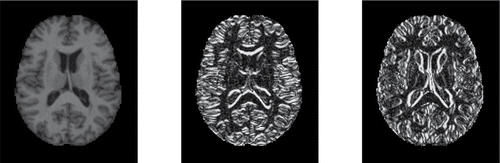
Figure 14. MRI image (left) and DO heatmap (right) of subjects 387 (top), 92 (middle), and 126 (bottom).
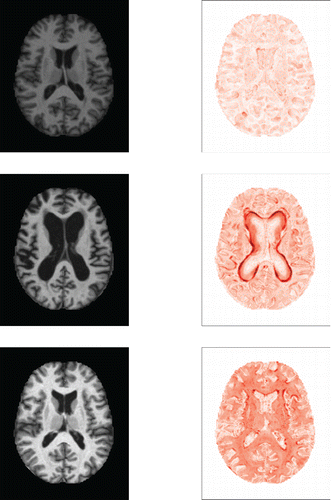
Figure 17. Left: Frames 100, 487, 491, and 500 from the video. Middle: DO heatmaps of these frames. Right: FOM with blue marker at the position of the frame.
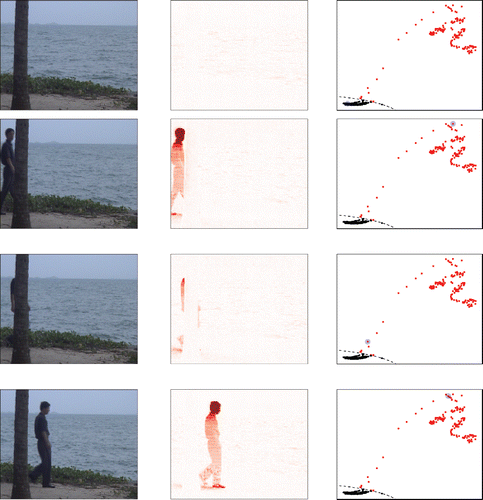
Figure 18. Percentage of outliers found in univariate lognormal samples of size n = 200 (left), n = 500 (middle), and n = 1000 (right), with 10% (top) and 15% (bottom) of outliers in x.
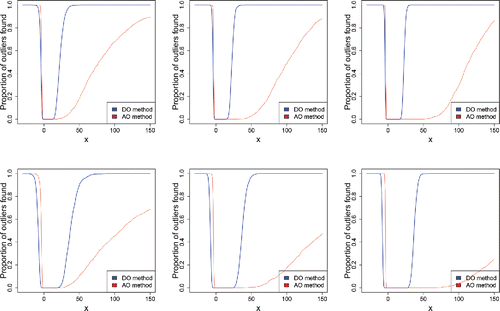
Figure 19. Percentage of outliers found in multivariate skew normal samples of size n = 200 (left), n = 500 (middle), and n = 1000 (right), with 10% of outliers around , in dimensions d = 2 (top), d = 5 (middle), and d = 10 (bottom).
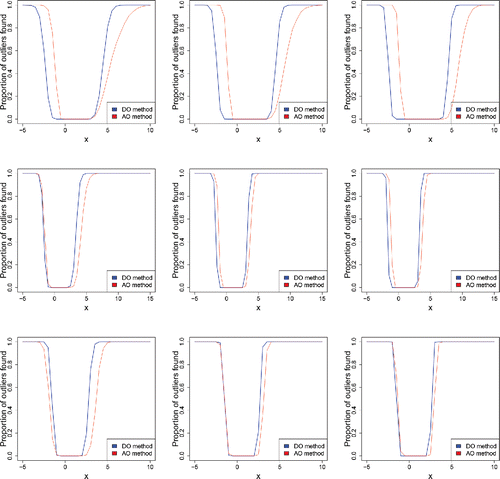