Figures & data
Table 1 (Example 1) Results for : effective sample size (ESS) (the larger the better), mean squared error (MSE) of
(the smaller the better).
Fig. 1 (Example 1) Illustration of one AIMM increment for the target π1. Top: States of the AIMM chain, proposed new state
activating the increment process (i.e., satisfying
), and neighborhood of
,
. Bottom: Target π1, defensive kernel Q0, first increment
, and updated kernel Q1 plotted on a logarithmic scale.
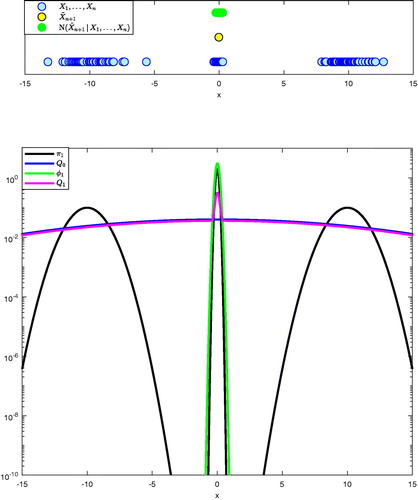
Fig. 2 (Example 1) Illustration of AIMM sampling from the target π1 for iterations sequence of proposals from Q0 to Qn produced by AIMM.
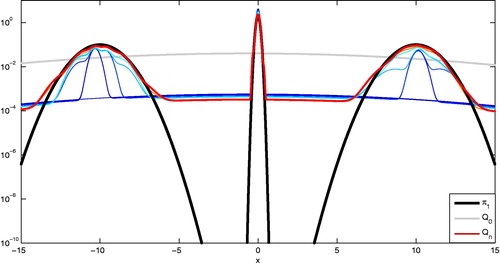
Fig. 3 (Example 2: π2 target, d = 2) Incremental mixture created by AIMM for three different initial proposals. Top row: Q0 is a Gaussian density (the region inside the black dashed ellipses contains 75% of the Gaussian mass) centred on a high density region (left) and a low density region (right). Bottom row: Q0 is Uniform (with support corresponding to the black dashed rectangle). The components of the incremental mixture obtained after
MCMC iterations are represented through (the region inside the ellipses contains 75% of each Gaussian mass). The color of each ellipse illustrates the corresponding component’s relative weight
(from dark blue for lower weights to red).
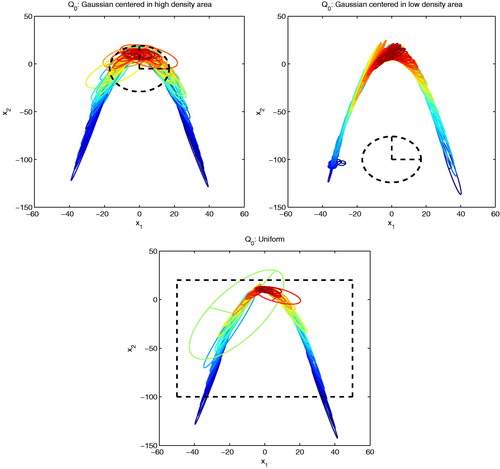
Table 2 (Example 2: π2 target, d = 2) Influence of the initial proposal Q0 on AIMM outcome after MCMC iterations (replicated 20 times).
Fig. 4 (Example 2: π2 target, d = 2) AIMM’s incremental mixture design after MCMC iterations. (a) Evolution of the number of kernels Mn created by AIMM for different thresholds. (b) Evolution of the KL divergence between
and the incremental proposal Qn, plotted in lin-log scale.
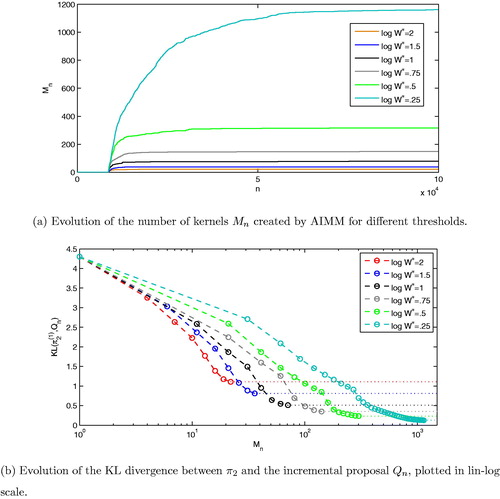
Table 3 (Example 2: π2 target, d = 2) Influence of the threshold on AIMM and f-AIMM outcomes after
MCMC iterations (replicated 20 times).
Fig. 5 (Example 5) Information regarding the acceptance rate of 100 AIMM runs are reported on the left panel and the evolution of the number of components for about 10 runs throughout the sampling is shown on the right panel.
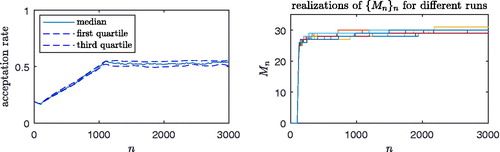
Table 4 (Example 3: π3 target, d = 6) Comparison of f-AIMM with the four other samplers after iterations (replicated 10 times).
Table 5 (Example 4: π4 target, ) Comparison of f-AIMM with RWMH, AMH, AGM, and IM after
iterations (replicated 100 times).
Table 6 (Example 4: π4 target, ) Mean square error (MSE) of the mixture parameter λ, for the different algorithms (replicated 100 times).
Fig. 6 (Example 5) Marginal posterior distribution of three parameters of the model estimated after 70,000 iterations of AIMM.
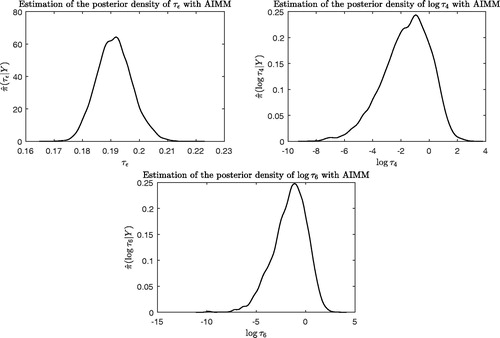
Fig. 7 (Example 6) Marginal posterior of four parameters estimated by AIMM and ARMS after a long run of 100,000 iterations of both algorithm (full swipe update Metropolis-within-Gibbs for ARMS).
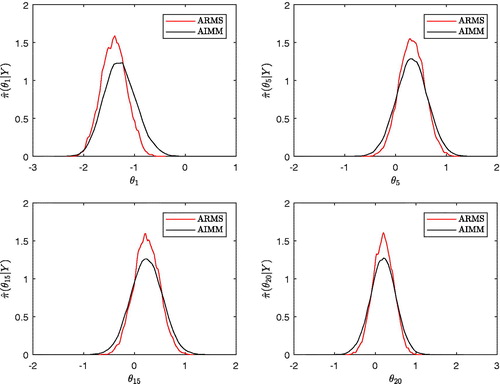
Table 7 (Example 6) Comparison of the performance of three adaptive MCMC samplers: acceptance rate, integrated autocorrelation time and average squared jumping distance.