Figures & data
Fig. 1 Empirical cumulative distribution functions (CDFs) associated with MCMC output for the standard Gaussian -marginal under the “funnel”-model
,
. For visual clarity, only the left half of the distributions are presented. Further details on this experiment can be found in Section 5.1. Each case is based on 5000 samples from each of 10 independent replica. The black solid lines are the empirical CDFs, whereas the red dashed lines are the true CDFs. The shaded gray region would cover 90% of empirical CDFs based on 50,000 iid N(0, 1) samples point-wise. The four left-most panels are based on Stan output with different values of the accept rate target δ. In practice, higher values of δ corresponds to smaller integrator step sizes and more integrator steps per produced sample. The right-most panel shows output for the proposed methodology using constant event rates. For both Stan and the proposed methodology, an identity mass matrix was employed.
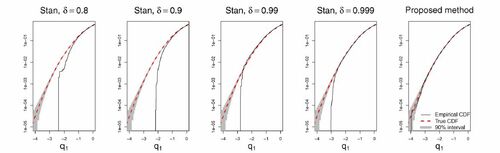
Table 1 The event specifications applied in the reminder of this text.
Fig. 2 Examples of q-coordinate of continuous time HMC trajectories with different event specifications for a bivariate standard Gaussian target distribution . In all cases,
and the shown trajectories correspond 100 units of time t. Events are indicated with red circles, and the common initial q-coordinate is indicated with a cross. In the rightmost panel, an event rate favoring events when the distance between the q-coordinate and its projection onto the subspace spanned by
(indicated by a gray line) is small.
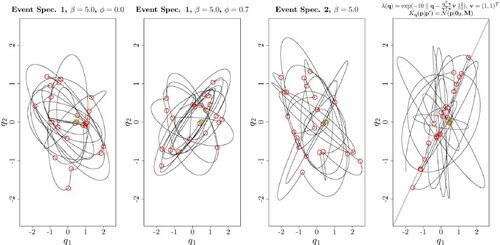
Fig. 3 RMSE of estimates of from NGRHMC processes using event specification 1 for different values of the mean inter-event time parameter β. The panels correspond to the different univariate target distributions
, which all have zero mean and unit variance. Unit mass matrix M = 1 was used. The estimates of the mean are based on trajectories of length
, and the RMSE estimates are based on 10,000 independent replica for each value of β. Black circles correspond continuous sampling (7), red ×-s to 1000 equally spaced samples, blue squares to 500 equally spaced samples and green triangles to samples recorded at events only. The horizontal lines give the RMSE of 1000 iid samples. The results are obtained using the numerical methods described in Section 4.1 with
.
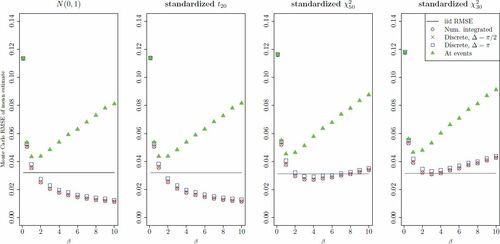
Table 2 Results for the “smile”-shaped target distribution (22,23).
Fig. 4 Numerical errors incurred by RKN integration on the estimation the first and second order moments of a bivariate Gaussian target distribution. The numerical errors are relative to a NGRHMC trajectory using the same random numbers but with exact Hamiltonian dynamics. Both exact and numerically integrated results are based on continuous sampling. The horizontal axis gives the error tolerances (in all cases with ) applied in the numerical integrator, and both horizontal and vertical axis are logarithmic. Dotted lines indicate the root mean squared errors associated with estimating the indicated moments across many exact NGRHMC trajectories of different length T.
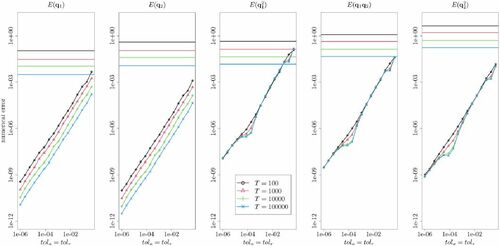
Table 3 ESSes and time weighted ESSes for the logistic regression model (24,25) applied to the German credit data.
Table 4 Effective sample sizes and computing times for the dynamic inverted Wishart model (26,29).