Figures & data
Fig. 1 The Italian seismic dataset for machine learning (INSTANCE). (a) Earthquake locations; (b) Seismic stations used for waveforms extraction. The symbol sizes are proportional to earthquake magnitude and number of arrival phases recorded by stations, respectively; (c) Seismic waveforms of some events with magnitude in the range . Vertical lines indicate the seismic waves’ arrival times. Source: Michelini et al. (Citation2021).
![Fig. 1 The Italian seismic dataset for machine learning (INSTANCE). (a) Earthquake locations; (b) Seismic stations used for waveforms extraction. The symbol sizes are proportional to earthquake magnitude and number of arrival phases recorded by stations, respectively; (c) Seismic waveforms of some events with magnitude in the range [2,4]. Vertical lines indicate the seismic waves’ arrival times. Source: Michelini et al. (Citation2021).](/cms/asset/bebd9ee8-67fe-4b3e-933d-b9244b145389/ucgs_a_2206441_f0001_c.jpg)
Fig. 2 (a) Simulated earthquake locations. (b) Simulated waveform marking the highlighted point on panel (a). (c) Result of the global test.
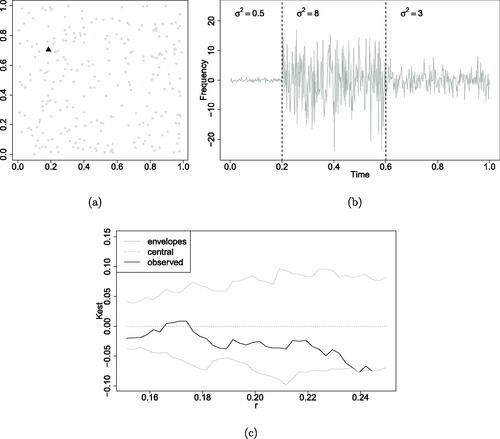
Fig. 3 (a) Result of global test for the spatially dependent simulated data. (b) Output of the local test: the black triangles are the significant points for which the hypothesis of random labeling is rejected.
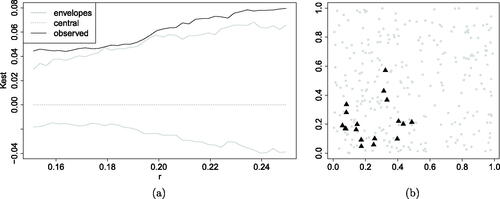
Fig. 4 Simulation scenarios. (a)–(c) Spatial ground patterns; (d)–(f) Functional marks of model (11) (in gray) and of the marking models in item 1, 2, and 3, respectively (in black).
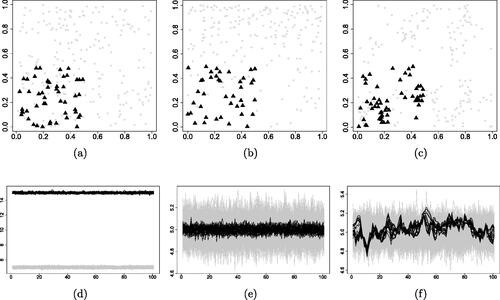
Table 1 Results of the local test averaged over 100 simulated point patterns with an expected point count of 250 each.
Fig. 6 Local K-functions. (a) The K-function is based on a kernel intensity estimate whose bandwidth is selected by Diggle’s (Citation2013) rule. (b) The bandwidth is chosen as in Cronie and Van Lieshout (Citation2018).
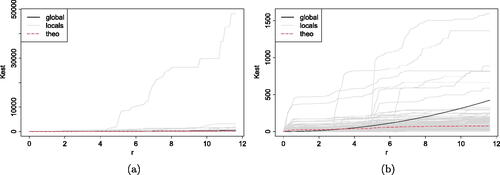
Fig. 7 Results of the local test at . Nonsignificant events are displayed as gray points and significant events are the black triangles. (a) The K-function is based on a kernel intensity estimate whose bandwidth is selected by Diggle’s (Citation2013) rule. (b) The bandwidth is chosen as in Cronie and Van Lieshout (Citation2018).
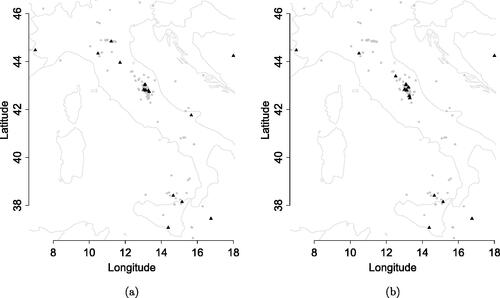