Figures & data
Fig. 1 Realizations from Gaussian processes on with zero mean, unit variance, and correlation function
(top right) and
(bottom right), and their corresponding Vecchia approximations for
(from left to right), using a coordinate-based ordering.
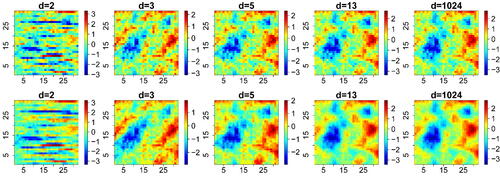
Fig. 2 Left: Root mean squared error of the composite likelihood estimator (dashed lines) with
(thin to thick) and cutoff distance δ = 2 (keeping about 10% of pairs), and the Vecchia likelihood estimator
(solid lines) with
(thin to thick) and based on a coordinate ordering. Right: Relative efficiency of
with respect to
for
(thin to thick). The Brown–Resnick model is considered here with parameters σ = 10 and
(weak to strong dependence).
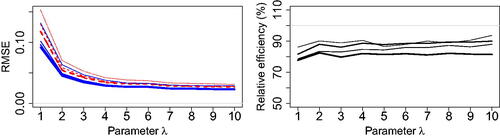
Table 1 Root mean squared error () for the composite likelihood estimator
(left) with
and cutoff distance
, and of the Vecchia likelihood estimator
(right) with
and coordinate-based (p1), random (p2), middle out (p3) and maximum–minimum (p4) orderings.
Table 2 Computational time (hr) for the composite likelihood estimator (left) with
and cutoff distance
, and of the Vecchia likelihood estimator
(right) with
and coordinate-based (p1), random (p2), middle out (p3) and maximum–minimum (p4) orderings.
Fig. 3 Left: Map of the study domain, with the spatial grid (dots) covering the Red Sea at which SST data are available. Ellipses show contours of the fitted extremal coefficient function , with respect to the grid cell at the center, obtained by fitting the anisotropic Brown–Resnick max-stable model using the best Vecchia likelihood estimator. Right: Validation locations (dots) used in our cross-validation study.
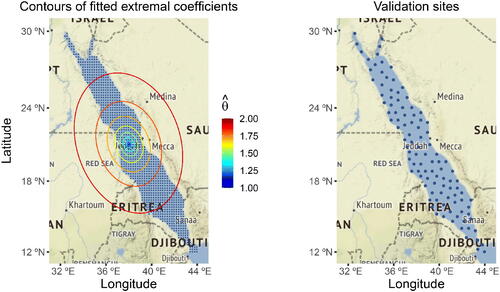
Table 3 Negative conditional log score S in (11) for each scenario based on a selection of 10% evenly spread validation locations.
Table 4 Computational time used in each scenario, measured in seconds.
Fig. 4 Binned bivariate empirical extremal coefficients (boxplots), plotted as a function of the Mahalanobis distance , and their model-based counterparts (dashed curves) for the best anisotropic models obtained using the Vecchia likelihood approach (left) and traditional composite likelihood approach (right). The settings of these four “best models” can be read from .
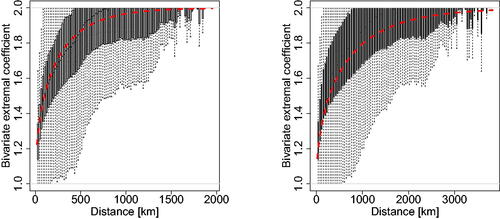
Fig. 5 Binned empirical extremal coefficients (boxplots) and their model-based counterparts (curves) for different directions, that is, (subpanels from top left to bottom right), computed by fitting the best anisotropic models obtained with the Vecchia method (solid curves) and the composite likelihood method (dashed curves) based on sub-datasets of size
of the complete dataset (light to dark). The different shades of gray of the binned boxplots correspond to the number of data points used in each boxplot, with darker gray corresponding to more points.
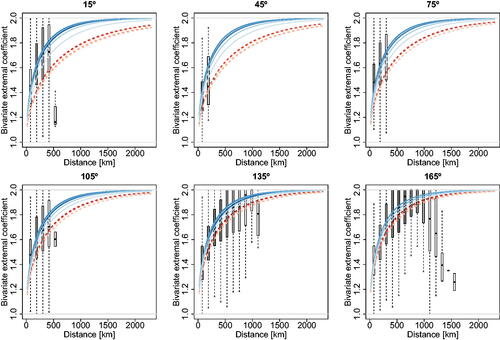