Figures & data
Figure 1. Distribution of pNOEC values as counts and normalized distribution functions a) for the chemicals in the training, DEV, and VAL set as defined in the Model development section, and b) according to their main pesticidal indication
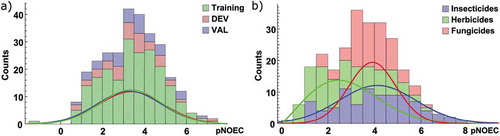
Table 1. Comparison of descriptor packages and their performance in training (r2) and cross-validation (q2) for a less complex model (3 latent variables (LVs) with 5 descriptors each) and a more complex model (5 LVs without regularization)
Figure 2. Goodness of fit (r2) and cross-validated q2 of sPLS models with increasing numbers of latent variables (LV) and descriptors per LV from the packages CDK, RDKit, PaDEL, Dragon, QM, and pEC50 daphnid
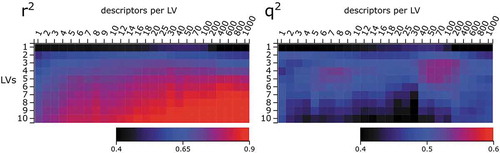
Figure 3. Average similarity to the 5 most similar compounds from the training set, based on Tanimoto distance of Unity fingerprints. For the training (left) and DEV (right) sets, all compounds from the training set were considered, while for the cross-validation (middle) only compounds from the other CV-batches were considered
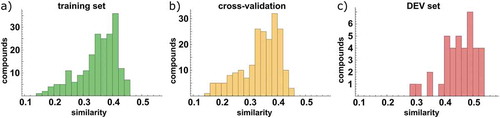
Table 2. Model performance summary along the major development steps, starting from all descriptors (CDK, RDKit, PaDEL, Dragon, QM and pEC50 daphnid) and gradually refining the model while reducing its complexity
Table 3. Model coefficients (ci) and descriptors for use with Equationequation 3(3)
(3) and model M6 (for more detailed descriptions see Table S2 in the Supporting Information)
Figure 4. Loadings plot of the 9 descriptors and pNOEC for the two latent variables in model M6. See descriptor explanations in Table 3
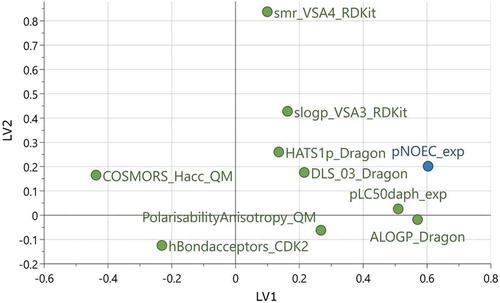
Figure 5. Model M6: Predictions with confidence intervals vs. experiment, for the training set compounds (a) and test sets (b), coloured in red (DEV), blue (VAL), and grey (EXT)
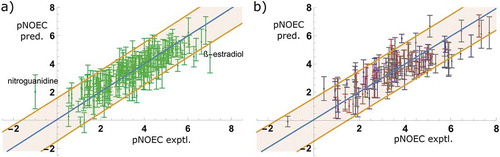
Figure 6. Error distribution of pNOEC predictions by model M6 as counts and normalized distribution functions a) according to set membership and b) for pesticide classes
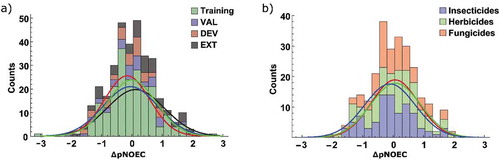
Figure 7. Error distribution of pNOEC predictions for pesticides by model M6, coloured according to mode of action (for an explanation of MoA acronyms see SI Table S8, and Figures S6-S8 for histograms on individual MoAs)
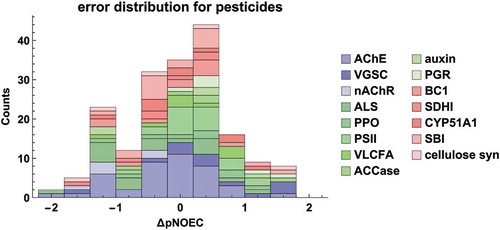
Figure 10. Representation of the chemical space covered by the full data set with 338 molecules. We used a t-stochastic neighbour embedding (tSNE) based on Tanimoto distances to embed chemical similarities in two dimensions. Training set (orange) and test set (blue) compounds of the models by Furuhama et al. highlighted in colour
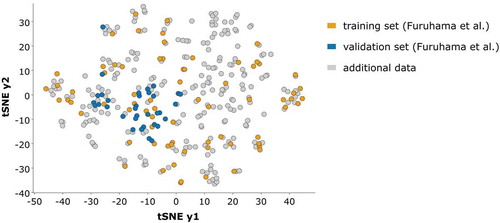
Table 4. Comparison of QSAAR model performance with models by Furuhama et al. [Citation15]
Supplemental Material
Download PDF (1.1 MB)Data availability Statement
The data that support the findings of this study are openly available via figshare at http://doi.org/10.6084/m9.figshare.c.5194022. The model workflow is available in KNIME Hub at https://kni.me/w/CEyXPUo_n1i4pUiR.