Figures & data
Table 1. Glossary of causal inference-related terms used in this article.
Figure 1. A simplified representation of the causal DAG relating smoking cessation and body weight. It includes the variables smoking cessation, body weight, baseline covariates, and time-varying covariates. The arrows represent the nonparametric links between them. Age, sex, ethnicity.
Body weight, socioeconomic factors, alcohol consumption, physical activity, energy intake, and comorbidities.
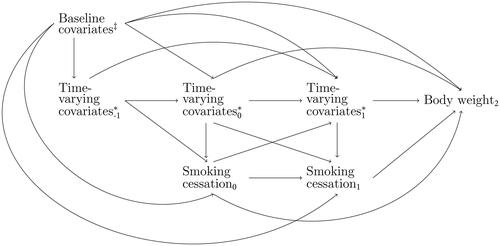
Table 2. Overview of covariates included in the LISS panel study.
Figure 2. Density of propensity scores for individuals who quit smoking versus individuals who continued smoking at time points 0 and 1 (before weighting). Propensity scores were computed using all covariates.
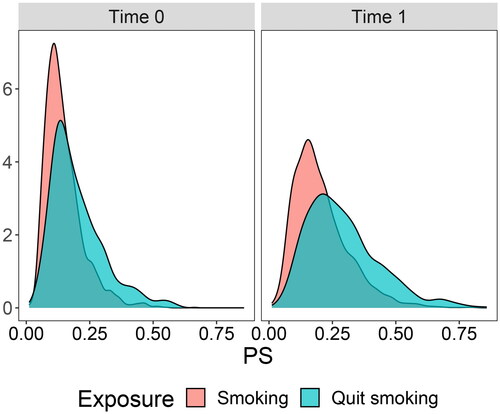
Figure 3. Visualization of covariate balance (standardized mean differences) before and after reweighing at time points 0 and 1. The asterisk * denotes binary covariates (or dummy variables) for which the displayed value is the raw (unstandardized) difference in means. PW = per week; PM = per month; P2M = per two months; PY = per year.
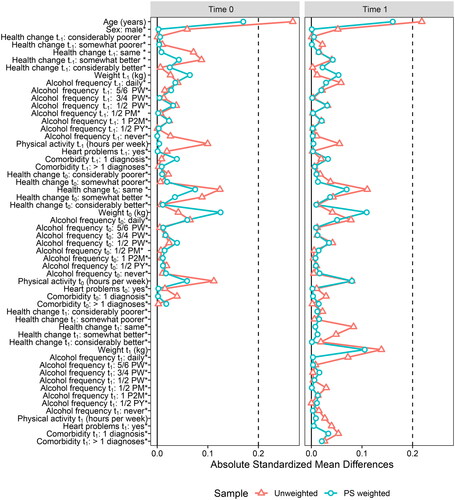
Figure 5. Overview of data generating mechanisms (DGMs) 2, 3, and 4. Bold black arrows in the DAGs indicate nonlinear dependencies. These direct effects are visualized in the plots to the right of each respective DGM, with the solid black line representing the true (nonlinear) functional relationship between two variables, and the dashed blue line representing the linear projection. DGM 1 (not illustrated here) contains only linear dependencies. DGM 5 (not illustrated here) combines the nonlinear dependencies of DGMs 2, 3, and 4.
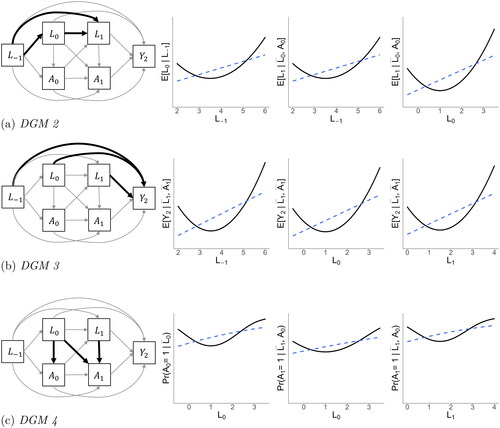
Figure 6. Bias in the point estimates of the always-exposed versus never-exposed effect across five methods: “IPW (L)” is linear IPW regression; “path (L) is linear path analysis; “IPW (L, Q)” is IPW regression with linear and quadratic terms in DGMs 3, 4, and 5; “path (L, Q)” is path analysis with linear and quadratic terms in DGMs 2, 3, 4, and 5; “unadjusted” is a linear regression without confounding adjustment. Results are presented for the case of n = 1,000, 10% and 50% exposed, and across five DGMs.
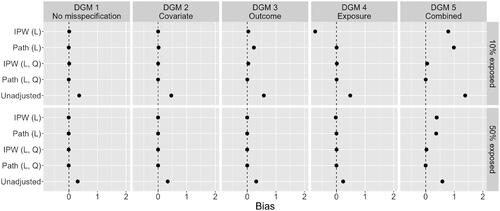
Figure 7. MSE of the point estimates of the always-exposed versus never-exposed effect across five methods, and five DGMS (n = 1,000).
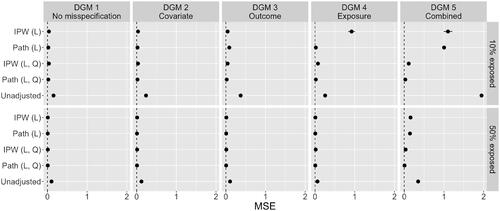
Table A1. Population values used for data generation.