Abstract
In recent years, outlier detection has attained great attention with machine learning techniques due to its wide range of applications. By considering the input data’s distributive nature and large dimensionality, outlier detection becomes a challenging issue. Robust outlier detection systems are crucial for data pattern prediction without labeled data. This research develops a novel approach based on stacking auto encoders over Long-Short Term Memory (LSTM) for outlier prediction. The detection accuracy of outlier detection is improved with the hyperparameters optimized with the Chaotic Gravitational Search Algorithm (CGSA). CGSA minimizes the training loss with enhanced detection accuracy in the proposed outlier detection process. The auto encoder in outlier detection transforms the input into a latent space representation to generate the original input sequence. The involvement of learning parameters computes and minimizes the errors between input and generated sequences. The proposed work is experimented and compared with state-of-the-art approaches of recent research. Using the proposed approach, the performance of outlier prediction is improved with an accuracy of98.6%, sensitivity of 96.1%, specificity of 97.8%, G-mean of 96%, Area Under Curve (AUC) of 0.935, Hit rate of 92.3%. Also, the outlier detection errors are minimized, showing the proposed approach’s efficiency.
Disclosure statement
No potential conflict of interest was reported by the author(s).
Data availability statement
Data sharing not applicable to this article.
Additional information
Notes on contributors
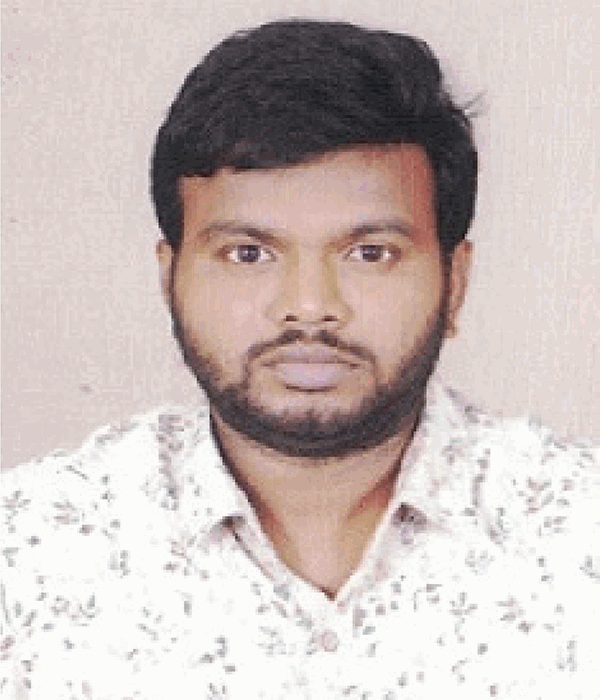
Chigurupati Ravi Swaroop
Chigurupati Ravi Swaroop is Research Scholar at Annamalai University, Faculty of Engineering and Technology, Department of Information Technology, Chidambaram, Tamilnadu, India. He Received B.Tech degree in Sagi Ramakrishnam Raju Engineering College, Department of Information Technology in 2012, He Holds a M.Tech degree in Sagi Ramakrishnam Raju Engineering College, Department of Information Technology in 2018. His research areas are Image Processing, Bioinformatics, Machine Learning, Deep Learning, and Data Mining. He can be contacted at email: [email protected].
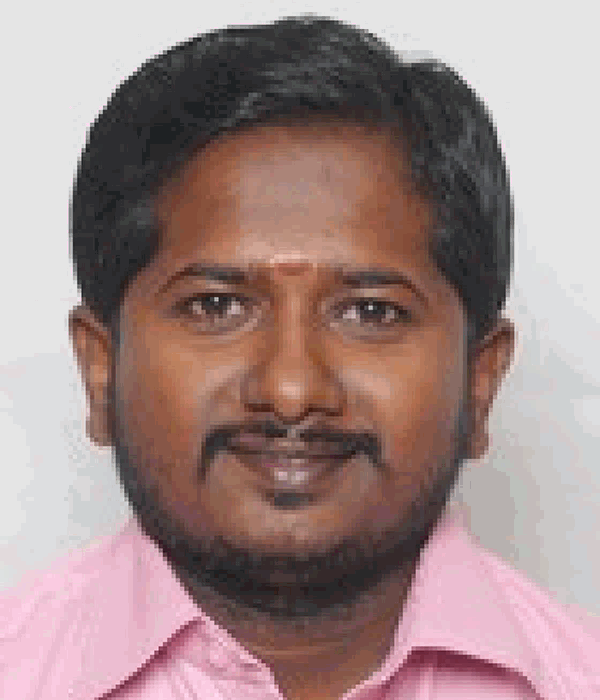
K. Raja
Dr. K. Raja is Assistant Professor at Department of Information Technology, Faculty of Engineering and Technology, Annamalai University, Tamilnadu, India. He Received B.E degree at Annamalai University, in 2006, He Holds a M.E degree at Annamalai University ,in 2010. He Received Ph.D degree at Annamalai University, in 2018, His research areas are Wireless Sensor Networks, Image Processing, Machine Learning, Deep Learning, and Data Mining. He can be contacted at email: [email protected].