Figures & data
Figure 1. 57 Districts and Counties in the Huaihai economic zone core city cluster study area. The scope of the study area follows the hierarchical relationship of provincial, city, and county levels from large to small. Figure a shows the spatial location of the Huaihai economic zone in China and its provincial relationships with the four surrounding provinces; figure b shows the extent of the city-level areas and core cities included in the Huaihai economic zone; and figure c shows the 57 county-level areas included in the core cities of the Huaihai economic zone.
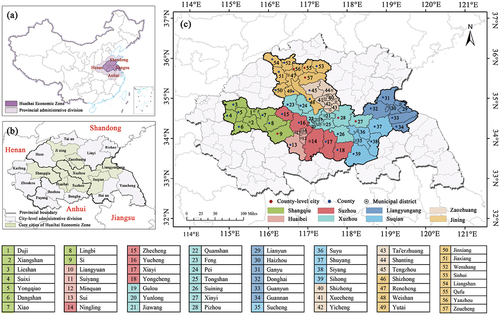
Table 1. Verification of spatial autocorrelation.
Table 2. Energy carbon emission factors.
Table 3. County carbon dioxide emissions in a linear regression model using data on evening light levels in a grayscale format.
Table 4. Spatial autocorrelation verification.
Table 5. Definition of carbon emission impact factors indicators.
Table 6. Statistical description of relevant variables.
Figure 5. Trends of total carbon emissions in core cities and counties of the Huaihai economic zone, 2001–2021.
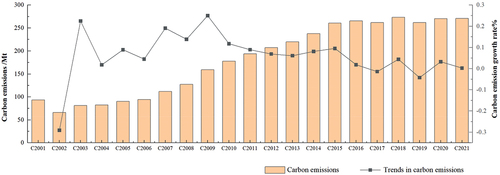
Figure 7. (a) State of carbon emission in the Huaihai economic zone in 2001, (b) state of carbon emission in the Huaihai economic zone in 2005, (c) state of carbon emission in the Huaihai economic zone in 2009, (d) state of carbon emission in the Huaihai economic zone in 2013, (e) state of carbon emission in the Huaihai economic zone in 2017, (f) state of carbon emission in the Huaihai economic zone in 2021; spatial and temporal changes in carbon emissions 2001–2021.
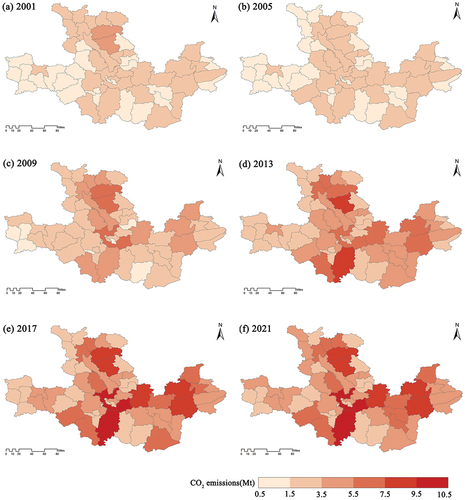
Table 7. Global Moran’s I of CO2 emissions at the county level, 2001– 2021.
Figure 8. Spatial autocorrelation local clustering analysis of carbon emissions. (a) Carbon emission local cluster analysis, 2001. (b) Carbon emission local cluster analysis, 2005. (c) Carbon emission local cluster analysis, 2009. (d) Carbon emission local cluster analysis, 2013. (e) Carbon emission local cluster analysis, 2017. (f) Carbon emission local cluster analysis, 2021.
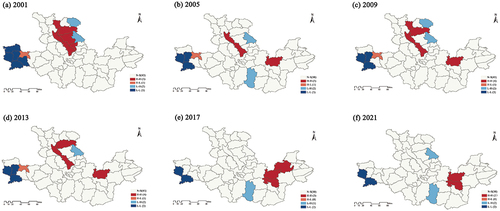
Figure 9. Spatial autocorrelation local significance analysis of carbon emissions. (a) Carbon emission local significance analysis, 2001. (b) Carbon emission local significance analysis, 2005. (c) Carbon emission local significance analysis, 2009. (d) Carbon emission local significance Analysis, 2013. (e) Carbon emission local significance analysis, 2017. (b) Carbon emission local significance analysis, 2021.
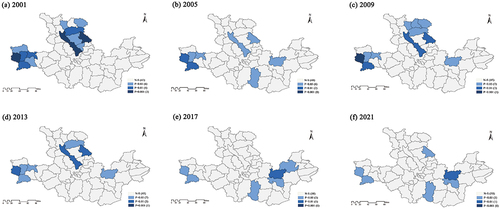
Table 8. Multi-collinearity test.
Table 9. Comparison of model evaluation indexes.
Table 10. Descriptive statistics of regression coefficients of the GTWR model.
Figure 10. Time series change trend of GTWR regression coefficients. (a) F1 (POP) time series trends of regression coefficients, 2001–2021; (b) F2 (DOP) time series trends of regression coefficients, 2001–2021; (c) F3(GOP)Time series trends of regression coefficients, 2001–2021; (d) F4(PGOP) time series trends of regression coefficients, 2001–2021; (e) F5 (SIJND) time series trends of regression coefficients, 2001–2021; (f) F6(UR)time series trends of regression coefficients, 2001–2021; (g) F7(UR)Time series trends of regression coefficients, 2001–2021.
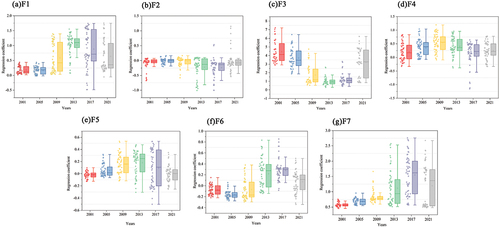
Figure 11. Spatial distribution patterns of carbon emission influencing factors based on GTWR model. (a) F1(POP)Spatial distribution characteristics of county territory. (b) F2(DOP)Spatial distribution characteristics of county territory. (c) F3(GOP)Spatial distribution characteristics of county territory. (d) F4 (Pgop)spatial distribution characteristics of county territory. (e) F5(SIJND)Spatial distribution characteristics of county territory. (f) F6(UR)Spatial distribution characteristics of county territory. (g) F7(EI) spatial distribution characteristics of county territory.
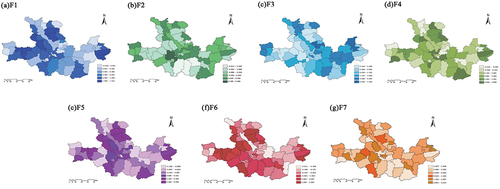