Figures & data
Figure 1. Intuition of our context-aware co-attention-based image captioning model. It consists of relation-aware attention, region-aware attention, transformer-based co-attention module, and -
-based language decoders. The relation-aware attention module is used to generate interactive words based on the relationships between objects. The relation-aware attention module is used to focus on related image regions to generate entity words. Transformer based co-attention module is used to capture the intra-modal and inter-modal interaction between image regions and objects.
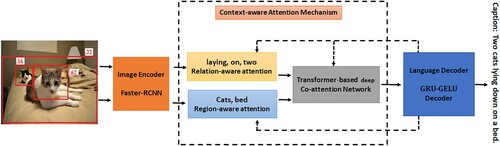
Table 1. Limitations of previous works.
Figure 2. An overview of our context-aware co-attention network-based image captioning model which consists of region-aware attention, relation-aware attention, -
decoder pair, and transformer-based co-attention network modules.
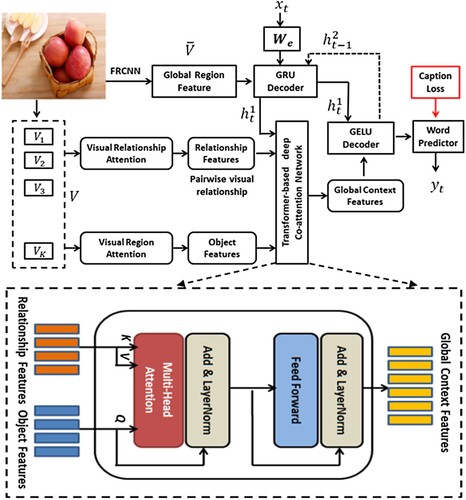
Figure 3. Transformer model-based co-attention network with multi-head attention for relationship features and object features to generate context-aware global features.

Table 2. Hyperparameter values.
Table 3. Ablation tests conducted on MSCOCO Karparthy test split.
Table 4. Results of our context-aware co-attention based image captioning model and compared models on MSCOCO Karpathy test split with cross-entropy loss.
Table 5. Results of our context-aware co-attention based image captioning model and compared models on MSCOCO Karpathy test split with CIDEr score optimization.
Table 6. Results of our context-aware co-attention based image captioning model and compared models on the Flickr30k dataset.
Table 7. Comparison with the existing models in terms of the number of parameters in million (M), training time on GPUs, Flops, layers, width and MLP on MSCOCO.
Figure 4. Qualitative results of our model and other models on MSCOCO. Ours indicates the captions generated by our model. EnsCaption [Citation28] and M2-Transformer [Citation23] are the strong comparative models. For each image, we have shown one interaction and two entity words. Highest attention weights are shown in red colour.
![Figure 4. Qualitative results of our model and other models on MSCOCO. Ours indicates the captions generated by our model. EnsCaption [Citation28] and M2-Transformer [Citation23] are the strong comparative models. For each image, we have shown one interaction and two entity words. Highest attention weights are shown in red colour.](/cms/asset/384c2567-fb0f-4198-8ba1-57fd55a4bef7/yims_a_2179992_f0004_oc.jpg)
Figure 5. Qualitative results of our model and other models on Flickr30k. Ours indicates the captions generated by our model. EnsCaption [Citation28] and Mul_Att [Citation11] are the strong comparative models. For each image, we have shown one interaction and two entity words. Highest attention weights are shown in red colour.
![Figure 5. Qualitative results of our model and other models on Flickr30k. Ours indicates the captions generated by our model. EnsCaption [Citation28] and Mul_Att [Citation11] are the strong comparative models. For each image, we have shown one interaction and two entity words. Highest attention weights are shown in red colour.](/cms/asset/fdfb68b3-ba86-4fd1-9b88-2815b395d17f/yims_a_2179992_f0005_oc.jpg)
Figure 6. Visualization results of attended regions/objects of our context-aware co-attention module during the decoding phase. Higher attention weights are shown in the form of brighter regions. (Best viewed in colour and ).
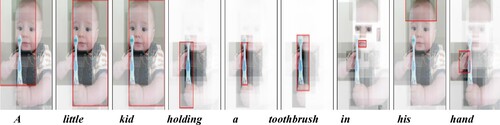
Figure 7. Same caption is generated by the proposed model for the left and middle images, as these two images are visually similar. In the rightmost image, our model fails to identify “onion” and “orange” and thus generates the wrong caption.
