Figures & data
Table 1. Considerable research for classifying foggy and clear images.
Table 2. Environment hyper-parameters.
Table 3. The data components used during experimentation of the model proposed.
Table 4. Confusion matrices for red, green and blue colour components when dropout is not used.
Table 5. Classification reports of the colour components when dropout is not used.
Table 6. Accuracy reports of the colour components when dropout is not used.
Figure 3. Training and validation loss for the (a) red (b) blue and (c) green colour components when dropout is not used: (a) training and validation loss for the red colour component; (b) training and validation loss for the green colour component and (c) training and validation loss for the blue colour component.
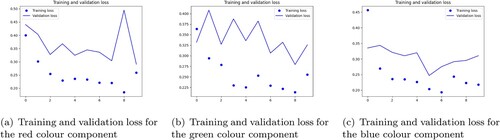
Figure 4. Training and validation accurcy for the (a) red (b) blue and (c) green colour components when dropout is not used: (a) training and validation accuracy for red colour component; (b) training and validation accuracy for the green colour component and (c) training and validation accuracy for the blue colour component.
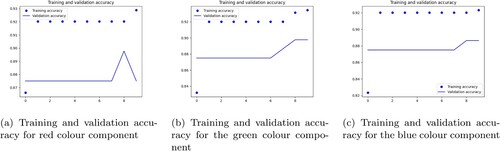
Table 7. Table for the values of step loss, accuracy, validation loss and validation accuracy for each of the colour components after using dropout.
Table 8. Confusion matrices for red, green and blue components of the final model.
Table 9. Classification reports of the colour components of the final model.
Figure 5. Training and validation loss for the (a) red (b) blue and (c) green colour components of the final model: (a) training and validation loss for the red colour component; (b) training and validation loss for the green colour component and (c) training and validation loss for the blue colour component.
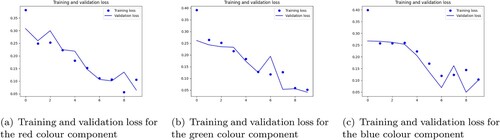
Figure 6. Training and validation accuracy for the (a) red (b) blue and (c) green colour components of the final model: (a) training and validation accuracy for the red colour component; (b) training and validation accuracy for the green colour component and (c) training and validation accuracy for the blue colour component.
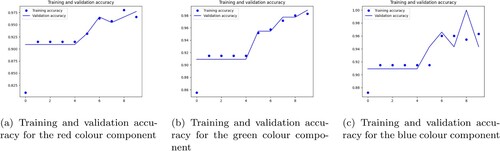
Table 10. Accuracy report for each of the colour components of the final model.
Table 11. Overall accuracy and loss report of the final model.
Figure 7. Classification of hazy and clear images on different outputs by different researchers: (a) clear images; (b) foggy images; (c) defogged by the method proposed by Li et al. [Citation3]; (d) defogged by the method proposed by Sebastian et al. [Citation37]; (e) defogged by the method proposed by Ancuti et al. [Citation38]; (f) defogged by the method proposed by Ngo et al. [Citation39]; (g) defogged by the method proposed by Li et al. [Citation40].
![Figure 7. Classification of hazy and clear images on different outputs by different researchers: (a) clear images; (b) foggy images; (c) defogged by the method proposed by Li et al. [Citation3]; (d) defogged by the method proposed by Sebastian et al. [Citation37]; (e) defogged by the method proposed by Ancuti et al. [Citation38]; (f) defogged by the method proposed by Ngo et al. [Citation39]; (g) defogged by the method proposed by Li et al. [Citation40].](/cms/asset/66bddcbd-9142-45e9-911a-3d5ede633feb/yims_a_2185429_f0007_oc.jpg)
Table 12. Comparative analysis of some existing machine learning classifiers.
Table 13. Ablation study of the proposed architecture with some existing deep learning image classification model.
Table 14. Comparative analysis of the proposed model with some existing deep learning techniques.
Table 15. Cross validation score.
Data availability
The data are available on request from the corresponding author.