Figures & data
Figure 1. An abstract depiction of the major cells involved in EAE and its associated regulatory recovery. MBP is the target of autoimmunity and is a protein expressed in the CNS. Adapted from [Citation20].
![Figure 1. An abstract depiction of the major cells involved in EAE and its associated regulatory recovery. MBP is the target of autoimmunity and is a protein expressed in the CNS. Adapted from [Citation20].](/cms/asset/59471c42-f05b-4612-a531-cb504840d6e6/nmcm_a_601419_o_f0001g.gif)
Figure 2. Examples of clinical data pertaining to the progression of EAE in mice. EAE severity is scored on a scale of 0–5, ‘1, flaccid tail; 2, hind limb weakness; 3, hind limb paralysis; 4, whole body paralysis; 5, death’ [Citation21]. (a) The mean EAE severity experienced by groups of 6–8 mice of different strains following the same induction of EAE administered in each group. Adapted from [Citation22]. (b) EAE severity experienced by each of 5 mice under different interventions (boxes A–C), over time (in days). Taken from [Citation21]. The interventions involve injecting mice experiencing EAE with varying quantities of Treg cells. (c) An indication of the number of CD4Th1 cells (EI on the y-axis) residing in a selection of organs at various times following the induction of EAE from [Citation23]. Organs examined were lymph nodes (LN), spleen (Sp), blood (Bl), lungs (Lu), bone marrow (BM), liver(Li), mesenteric lymph nodes (mLN) and thymus (Thy).
![Figure 2. Examples of clinical data pertaining to the progression of EAE in mice. EAE severity is scored on a scale of 0–5, ‘1, flaccid tail; 2, hind limb weakness; 3, hind limb paralysis; 4, whole body paralysis; 5, death’ [Citation21]. (a) The mean EAE severity experienced by groups of 6–8 mice of different strains following the same induction of EAE administered in each group. Adapted from [Citation22]. (b) EAE severity experienced by each of 5 mice under different interventions (boxes A–C), over time (in days). Taken from [Citation21]. The interventions involve injecting mice experiencing EAE with varying quantities of Treg cells. (c) An indication of the number of CD4Th1 cells (EI on the y-axis) residing in a selection of organs at various times following the induction of EAE from [Citation23]. Organs examined were lymph nodes (LN), spleen (Sp), blood (Bl), lungs (Lu), bone marrow (BM), liver(Li), mesenteric lymph nodes (mLN) and thymus (Thy).](/cms/asset/82598254-2575-43cb-a372-af9e50be9839/nmcm_a_601419_o_f0002g.gif)
![Figure 2. Examples of clinical data pertaining to the progression of EAE in mice. EAE severity is scored on a scale of 0–5, ‘1, flaccid tail; 2, hind limb weakness; 3, hind limb paralysis; 4, whole body paralysis; 5, death’ [Citation21]. (a) The mean EAE severity experienced by groups of 6–8 mice of different strains following the same induction of EAE administered in each group. Adapted from [Citation22]. (b) EAE severity experienced by each of 5 mice under different interventions (boxes A–C), over time (in days). Taken from [Citation21]. The interventions involve injecting mice experiencing EAE with varying quantities of Treg cells. (c) An indication of the number of CD4Th1 cells (EI on the y-axis) residing in a selection of organs at various times following the induction of EAE from [Citation23]. Organs examined were lymph nodes (LN), spleen (Sp), blood (Bl), lungs (Lu), bone marrow (BM), liver(Li), mesenteric lymph nodes (mLN) and thymus (Thy).](/cms/asset/2d690afb-3bd4-4869-9650-f1de7884f4b6/nmcm_a_601419_o_f0009g.gif)
Figure 3. The baseline behaviour of the EAE simulation. (a) The baseline behaviour of the EAE simulation, following calibration, in the presence of regulation. Graph shows the number of effector T cells in the system over time and the number of neurons killed per hour (×10 for clarity). This is the median of 1000 simulation runs. (b) The baseline behaviour of the EAE simulation, following calibration, with regulatory activity disabled by preventing CD8Tregs from killing CD4Th1 cells. This represents the median behaviour of 1000 individual runs such as that depicted here. (c) An example of a single simulation run, in the absence of regulatory activity. The number of CD4Th1 cells declines heavily to a minimum at around day 80, but rises again thereafter. This reflects the relapsing nature of EAE seen in absence of regulation in some mice. The median progressions shown in and (b) are compiled from unique individual executions such as that of (c).
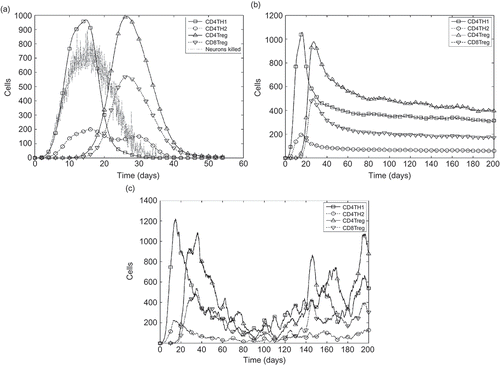
Figure 4. The nine responses adopted to analyse the EAE simulation, shown on a graph of the number of effector T cells over time. For each of the four T cell species, both the maximum number reached and the time at which that number was reached are assumed as a separate response (these are indicated as crosshairs on the diagram). The ninth response is the number of autoimmune CD4Th1 effector cells remaining at 1000 hours.
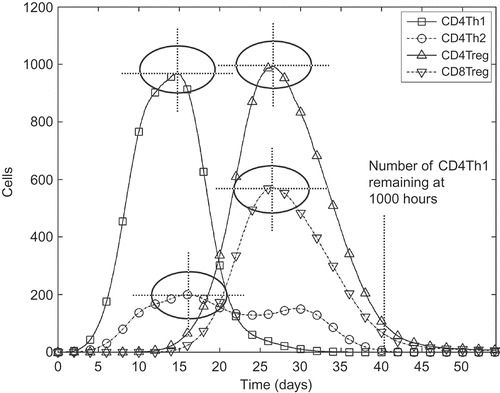
Table 1. The magnitude of effect size indicated by A test score [24]
Figure 5. Example robustness analysis of a parameter in the EAE simulation. Perturbation of this parameter has a large effect on all the responses. The parameter investigated here dictates the rate of TNF-α, a substance harmful to neurons in sufficient concentration, secretion by activated microglia cells in the CNS. (a) A test scores for the response distributions arising from parameter perturbations. The default value for this parameter is 100 and is where all lines on the graph converge. (b) The distribution of response data (maximum number of CD4Th1 cells reached) for each parameter perturbation. A dash, a circle and a cross above each box respectively indicate the default value, a change in distribution not considered large and a change that is considered large.
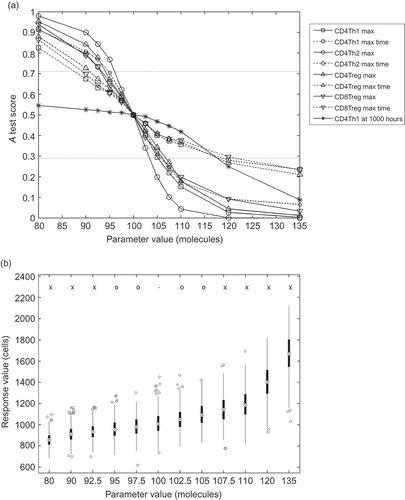
Figure 6. The maximum A test score achieved for each response over 20 dummy parameter experiments. The sample size represents the number of simulation runs used in compiling the response distributions for each dummy parameter experiment. Note that all scores below 0.5 are assigned corresponding values above 0.5 before maximum are performed; we are interested only in the magnitude of effect, not its direction above or below 0.5. The three effect magnitude boundaries for the A test are indicated.
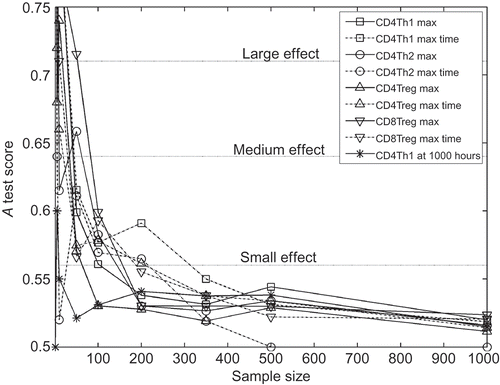
Figure 7. The effect of aleatory uncertainty on the results of the robustness analysis, for various sample sizes used when obtaining representative results from the simulation. The x-axes are labelled ‘dummy parameter’ as no parameters are actually being changed. The tests are designed to ascertain how the number of samples (simulation executions) from which median data are compiled affects the consistency of results. A test scores for 20 dummy parameter permutations, using sample sizes of (a) 5, (b) 50 and (c) 500.
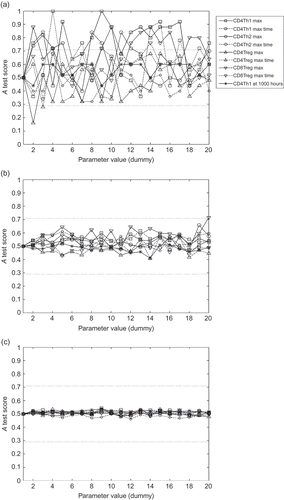
Table 2. An example of how sample size can reduce aleatory uncertainty, as measured by A test scores
Figure 8. Examples of latin hypercube design approach to determining relative parameter influence on simulation behaviour. The horizontal and vertical lines on (b) and (c) indicate the default parameter value and its associated response value. (a) An example latin hypercube design for two parameters. Ten samples are taken from the parameter space, within the specified domain of each parameter. Each of the 10 sub-domains (indicated by dotted lines) contains a sample, ensuring that the full domain of each parameter is explored. (b) This simulation parameter has a large influence on the time at which the maximum number of CD4Treg effector cells is reached during simulation execution. The rank correlation coefficient is 0.65. The parameter analysed here dictates the mean time that a T cell spends in a proliferative state before differentiating into an effector cell. (c) This simulation parameter has a small influence on the time at which the maximum number of CD4Treg effector cells is reached during simulation execution. The rank correlation coefficient is −0.001. The parameter analysed here is the rate of type 1 cytokine (an immune system messenger molecule) secretion by activated CD8Treg cells.
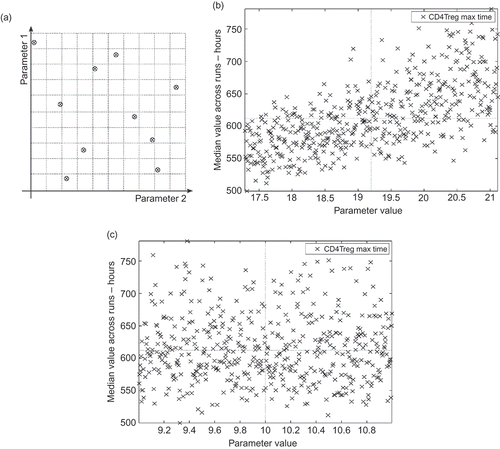