Figures & data
Figure 1. Schematic representation of the behavioural model used in the individual-based simulations. Arrows correspond to conditional/probabilistic transitions between behavioural states. For example, ‘Forage’ immediately leads to subsequent ‘Return’, but to initiate ‘Search’, bees have a certain probability to leave the inactive state in each time step. This leads to varying durations in each state. The transition from ‘Search’ to ‘Forage’ depends on how quickly the bee actually finds a food source in the spatially explicit simulation.
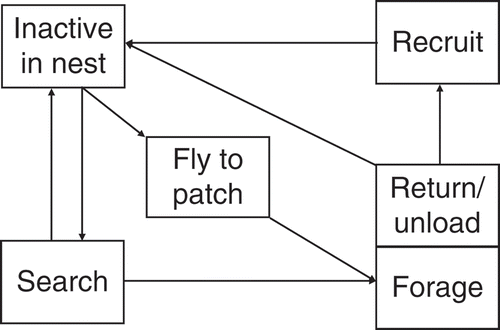
Figure 2. Total energy collected by the simulated bees as it depends on group size. Each data point is a simulation run. The ‘quality’ of patches corresponds to the volume of nectar available per trip (in μl). No effect of group size on energy collected per bee was found. (a) and (b) show foraging success for a poor habitat; and (c) and (d) show foraging success for a rich habitat. (a) and (c) are simulations without recruitment; (b) and (d) are simulations with recruitment.
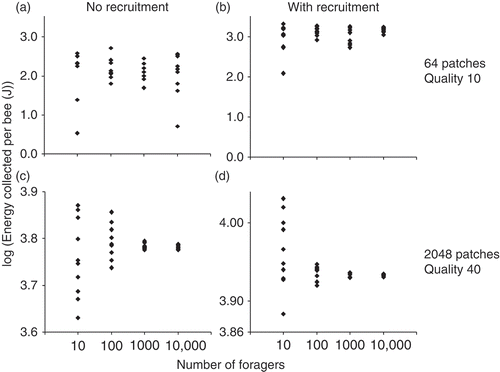
Figure 3. (a) In our model, bees had a higher net energy gain in patchy environments (ones with few, rich resources) compared with ones with many small resources. Boxes show median and interquartile interval, whiskers show range; grey: with recruitment; white: without recruitment. (b) In the same environments, the benefits of recruitment, however, were always the same (no relationship with patchiness). Each data point is the ratio of energy collected in a simulation run with recruitment over that collected in a simulation run without recruitment.
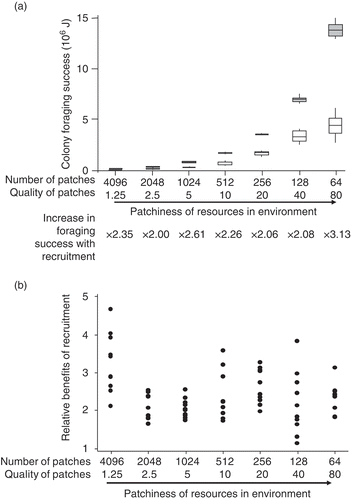
Figure 4. (a) Relative benefits of recruitment (foraging success with/foraging success without communication) decrease with number of patches in the environment and with quality of patches (amount of nectar available per trip). Benefits of recruitment are thus highest with few, low-quality patches (for full analysis see [Citation14]). (b) The proportion of the energy collected with recruitment that was dependent on recruitment being present [(E recr–E norec)/E recr] also decreases with increasing number or quality of resources.
![Figure 4. (a) Relative benefits of recruitment (foraging success with/foraging success without communication) decrease with number of patches in the environment and with quality of patches (amount of nectar available per trip). Benefits of recruitment are thus highest with few, low-quality patches (for full analysis see [Citation14]). (b) The proportion of the energy collected with recruitment that was dependent on recruitment being present [(E recr–E norec)/E recr] also decreases with increasing number or quality of resources.](/cms/asset/3c2e14fc-7b1e-4741-8497-e9b5357f90bc/nmcm_a_601422_o_f0004g.gif)
Figure 5. Schematic representation of the task allocation model. Different task allocation rules are compared by changing the ‘Select task object’ routine. The simulation is spatially explicit with demand for each task continuously increasing at every grid cell and workers moving around on the grid randomly to search for work.
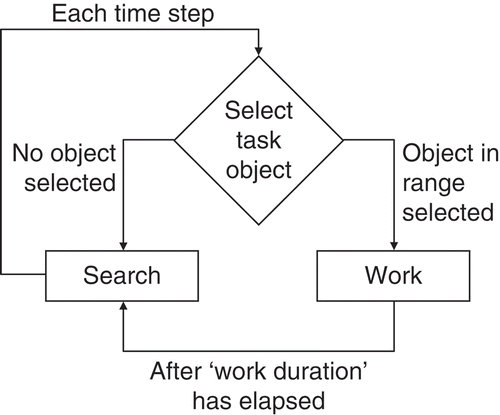
Figure 6. Three different measures of group-level performance all show that strategies differ significantly in the level of task completion achieved. In addition, not only group size but also the density of workers in a given work area affect which strategy performs optimally. (a) ‘Task demand’, the simulation parameter that shows whether workers have attended to a particular task type in a particular position, is averaged over all positions and task types at the end of the simulation. ‘Proportion of work accomplished’, shown here, is defined as (1 – average remaining demand level) (shown are averages of 10 simulation runs with standard deviations). Different letters above bars indicate significant differences in Tukey's post hoc test (for each condition separately). (b) Another measure is the evenness with which all positions and task types are attended to. Here, the amount of variation produced by the different task allocation strategies is shown as the standard deviation in task demand level at the end of the simulation. (c) The third measure shown here reflects how many positions received extremely low levels of work across the simulation. n.s. denotes no significant difference among strategies.
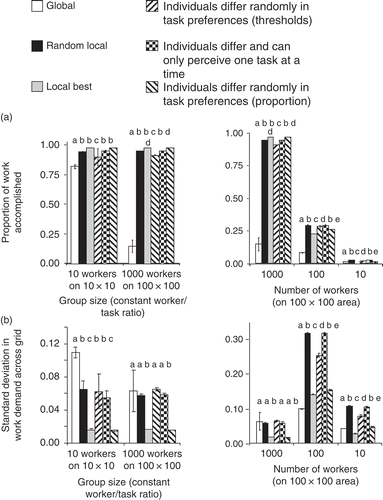
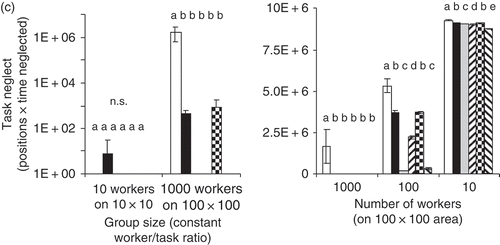