Figures & data
Figure 1. Generation of an optimal experiment design (OED) in an online procedure based on an evolving model.
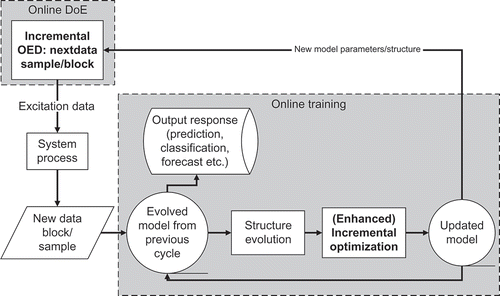
Figure 2. Contour lines of the validity functions of a local model network with six local models. The partition space is spanned by and
.
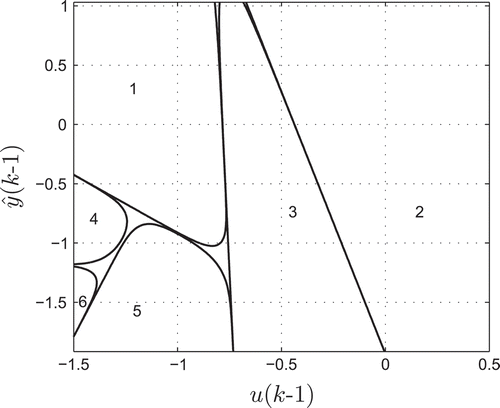
Figure 5. Illustration of an intersection plot of the smoke model. The output is depicted in the unit of filter smoke number (FSN) for a fixed exhaust gas recirculation (EGR) rate, timing of main injection and timing of pilot injection.
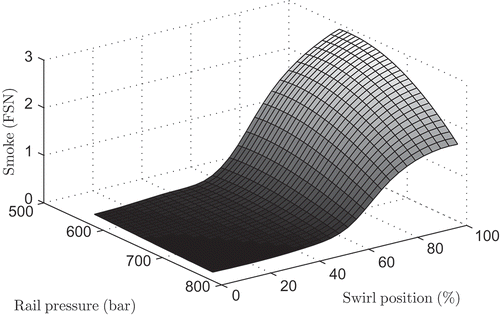
Figure 6. Comparison of normalized optimality criteria. The D criterion denotes the determinant of the Fisher information matrix and the D-S criterion denotes the hybrid criterion combining the determinant of the Fisher information matrix with a distance measure.
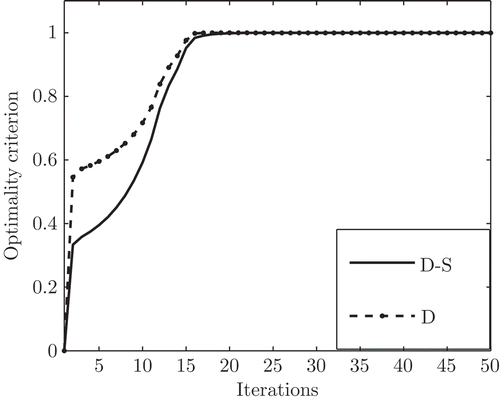
Table 1. Comparison of the number of design points for three particular model errors (MSE) and three experimental designs.
Figure 8. Illustration of the model quality in the form of the mean squared error (MSE) as a function of the sample size for the D-S-optimal design, the S-optimal design and the fullfactorial design.
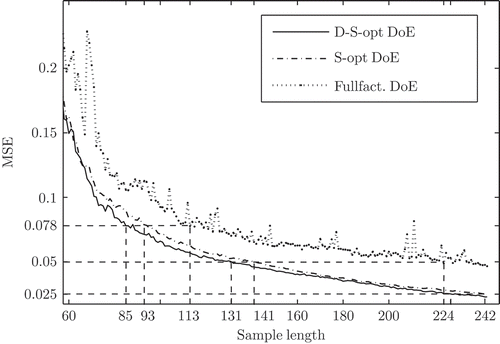
Figure 10. The upper part illustrates the design of experiments (DoE) given by the injection mass signal and the lower part indicates the associated system response (exhaust temperature). The snapshot is taken exactly when the initial DoE sequence (first 300 samples) is applied to the system. The outlook on the future model-based DoE (mDoE) ranges from the 301st to the 320th sample. For the future mDoE, the exhaust temperature is demanded to comply with the feasible range from 620K to 780K.
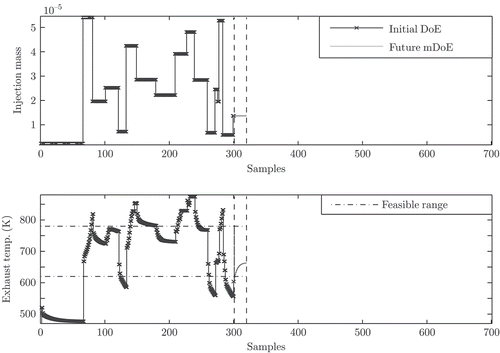
Figure 11. The upper part illustrates the design of experiments (DoE) given by the injection mass signal and the lower part indicates the associated system response (exhaust temperature). The snapshot of the DoE procedure has been taken after Figure 10 when 190 samples of the model-based DoE sequence (mDoE) have already been applied to the system. The applied mDoE sequence (301st to 490th sample, grey line) generates a system response that stays well within the feasible exhaust temperature range. The outlook on the future model-based DoE (solid black line) ranges from the 491st to the 510th sample.
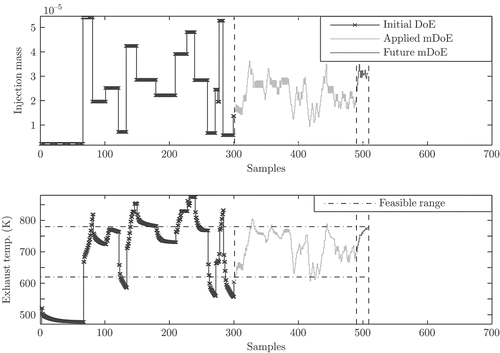
Figure 12. The upper part illustrates the design of experiments (DoE) given by the injection mass signal and the lower part indicates the associated system response (exhaust temperature). The snapshot of the DoE procedure has been taken after Figure 11 when 380 samples of the model-based DoE (mDoE) sequence have already been applied to the system (301st to the 680th sample, grey line). The applied mDoE sequence generates a system response that stays well within the feasible exhaust temperature range. The outlook on the future model-based DoE (solid black line) ranges from the 681st to the 700th sample.
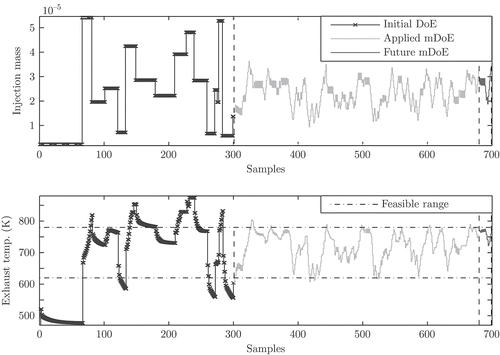
Figure 13. Distribution of the exhaust temperature for the model-based design of experiments (mDoE) and the amplitude modulated pseudo random binary sequence (APRBS). The optimal excitation signal is generated with a minimal and a maximal exhaust temperature of 620K to 780K.
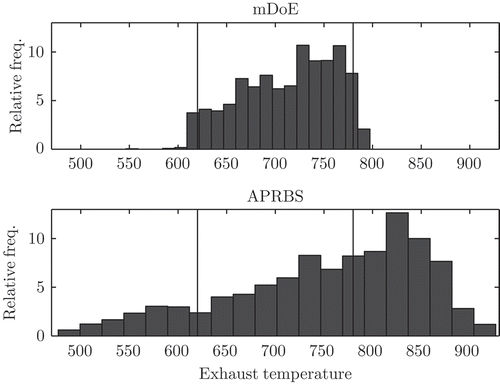