Figures & data
Figure 2. Isolating neighbourhoods for equilibria u1, u2, u3 from Figure 1 as a function of parameter q and a fixed r. The isolating neighbourhood for u0 is omitted for clarity.
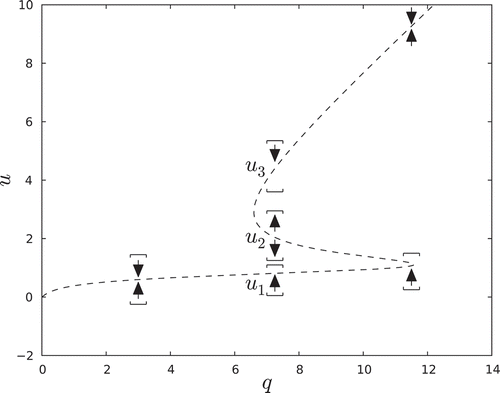
Figure 3. Morse graph for Morse decomposition for M (Sq), q = 7.5, see Figure 2. (u0 has been included.)
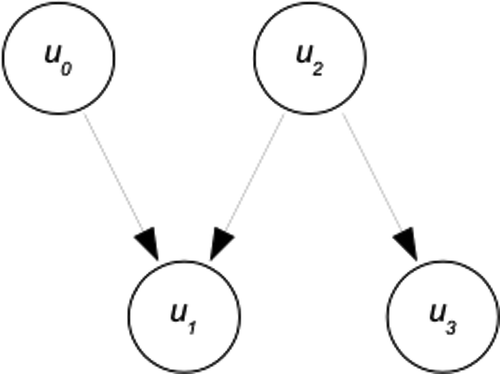
Figure 4. (a) Equilibria states in a general cusp catastrophe model. In the region of the fold, three equilibrium states exist. One equilibrium exists in other regions. The dashed vertical line shows the arrangement of the equilibria in phase space for an arbitrary pair of parameters (q, r) in the cusp region. The repeller and attractors in the Morse graph in Figure 3 correspond to u1, u2 and u3. (b) Parameter space showing the projection of the folded region on the right.
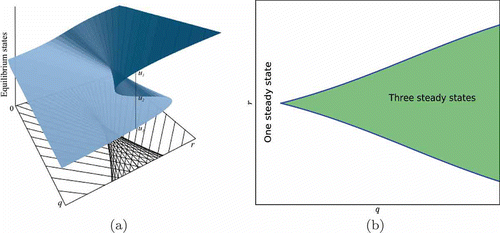
Figure 5. A slice of parameter space at z = 1. (a) Continuation classes from the original paper by Arai et al.; (b) Classes obtained without machine learning algorithms, but using the coarser notion of equivalence. Classes labels, C*, correspond between (a) and (b) as well as to those in the figures below (colour online).
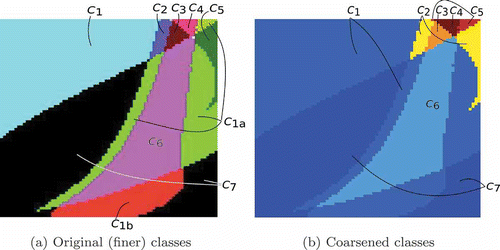
Table
Table
Figure 6. (a) z slice 1, training set size 10% of , misclassification of 244 points, or 3.8125% classification error; (b) z slice 9, training set size 20% of
, misclassification of 79 points, or 1.234% classification error. As in Figure 5, classes that correspond across the slices are labelled the same. Notice that in (b) class C′2 is a newly detected class, while the dynamics detected in classes C2 and C3 do not show up in the z = 9 slice of parameter space.
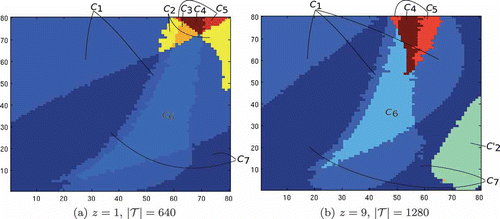
Table 1. Results for compilation of 2D z slices for AlgorithmD, AlgorithmN and Uniform Sampling.
Figure 8. Plot of training set for AlgorithmN on z = 1, , misclassification of 123 grid elements, or 1.92% of
; the shade of a training set element depends upon when it was selected in the process of constructing
. Classes correspond to those in Figures 5 and 6.
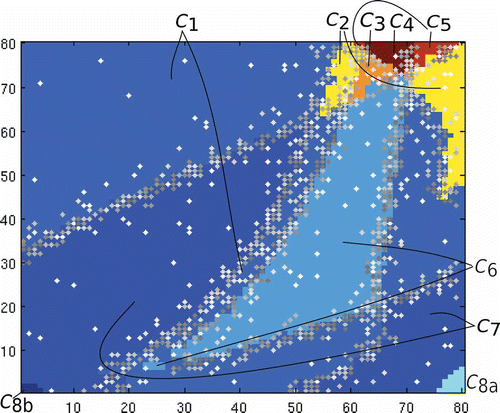
Table 2. Results for 3D Variants of AlgorithmN and Uniform Sampling.