Figures & data
Table 1. Actions taken based on the in-situ check of landmarks generated by the algorithm described by Rousell and Zipf (Citation2017)
Figure 1. Example of potential ambiguity. At the illustrated turning point (yellow circle), participants would have to make a left turn. The algorithm described by Rousell and Zipf (Citation2017) suggests to use the POI which is tagged with amenity:bank and named Erste Bank (green circle). Based on this, the route instruction would be (translated to English): Turn left at Erste Bank. However, this would have been ambiguous (see the red arrow) given the local spatial layout [source of background image: Filipe et. al (Citation2020)].
![Figure 1. Example of potential ambiguity. At the illustrated turning point (yellow circle), participants would have to make a left turn. The algorithm described by Rousell and Zipf (Citation2017) suggests to use the POI which is tagged with amenity:bank and named Erste Bank (green circle). Based on this, the route instruction would be (translated to English): Turn left at Erste Bank. However, this would have been ambiguous (see the red arrow) given the local spatial layout [source of background image: Filipe et. al (Citation2020)].](/cms/asset/6212fae2-d5a4-48be-8ab1-96d0debdb777/hscc_a_1942474_f0001_oc.jpg)
Figure 2. Example of overestimated salience. The algorithm by Rousell and Zipf (Citation2017) picks the beauty salon (red circle) as the most suitable landmark. However, this landmark cannot be recognized easily when approaching the turning point as can be derived from the picture on the left (for the specific spatial layout see the map on the right). The mall called Lugner City (highlighted in green) is clearly more salient and, hence, the algorithm’s choice was overruled by the experimenter [left: image by creator istefanos retrieved from Mapillary on Feb 26th, 2021, licensed under CC BY 4.0 and cropped); source of background image on the right: Filipe et. al (Citation2020)].
![Figure 2. Example of overestimated salience. The algorithm by Rousell and Zipf (Citation2017) picks the beauty salon (red circle) as the most suitable landmark. However, this landmark cannot be recognized easily when approaching the turning point as can be derived from the picture on the left (for the specific spatial layout see the map on the right). The mall called Lugner City (highlighted in green) is clearly more salient and, hence, the algorithm’s choice was overruled by the experimenter [left: image by creator istefanos retrieved from Mapillary on Feb 26th, 2021, licensed under CC BY 4.0 and cropped); source of background image on the right: Filipe et. al (Citation2020)].](/cms/asset/343591c9-9f4a-4b60-b757-1b801804cbc5/hscc_a_1942474_f0002_oc.jpg)
Figure 3. A: A sample participant in full equipment. B: GNSS receiver (PPM 10-xx38). C: During the experiment, participants requested navigation instructions using a custom-built clicker-device (circled in red) which triggers an LED light located in the backpack informing the experimenter about the request.
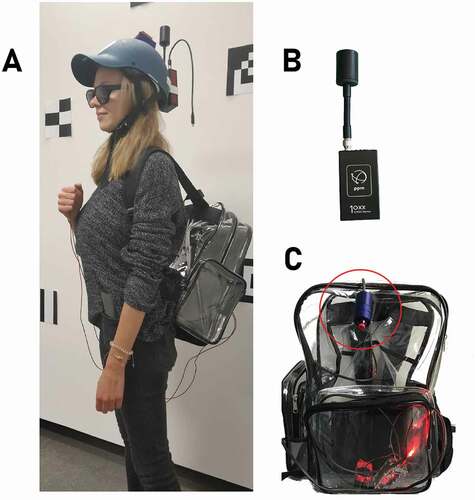
Figure 4. Segmentation process. Two possible situations: A (default case): The segment starts at the last turning point denoted as 3. The first intersection after the click position is denoted as 4 and the segment ends at this intersection. B: A route segment covering the distance from the starting point to intersection 2. It is reasonable to assume that the participant has not perceived intersection 1 as a decision point because the instruction is requested after it had been passed background image: Story Citation2013].
![Figure 4. Segmentation process. Two possible situations: A (default case): The segment starts at the last turning point denoted as 3. The first intersection after the click position is denoted as 4 and the segment ends at this intersection. B: A route segment covering the distance from the starting point to intersection 2. It is reasonable to assume that the participant has not perceived intersection 1 as a decision point because the instruction is requested after it had been passed background image: Story Citation2013].](/cms/asset/eff35af4-bd5a-499b-be4f-e9c333a2a4f6/hscc_a_1942474_f0004_oc.jpg)
Table 2. Description of influential variables according to our model results. Categories: P: participant, R: route, T: trial, E: environment. Sources: OQ: online questionnaire that was completed by participants, UA: Urban Atlas, OSM: OpenStreetMap
Table 3. Summary statistics of the observations used for model estimation. (d): denotes a dichotomous variable; for these variables column mean represents the proportion in the sample, SD: standard deviation, Min: minimum value, Max: maximum value, distance norm.: normalized distance; all other variable names are explained in
Table 4. Normalized timing of instructions based on the AFT model. The variables are explained in Table 2
Figure 5. Predicted median survival values based on the estimated AFT model compared against the observed ones.
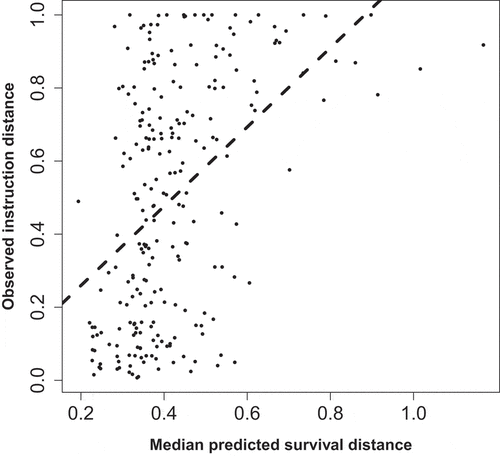
Figure 6. Empirical survival rates for two given subsets of observations (;
;
;
; left: familiar, right: unfamiliar segments), compared against the mean predicted ones. Dotted lines represent the 95% CI.
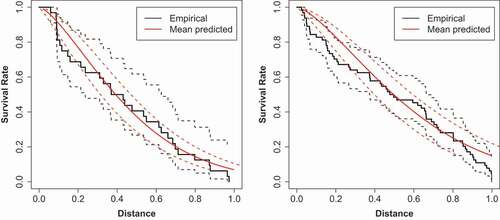
Figure 7. Variation of survival rate predictions due to explanatory variables modifications (continuous variables = 1 standard deviation (
), dummy variables =
1) compared to a base case scenario (BFI_o_low = 0, lngSegm = 1, age_gt_40 = 0, BFI_e_low = 0, unfamiliar = 1). Variable names are explained in .
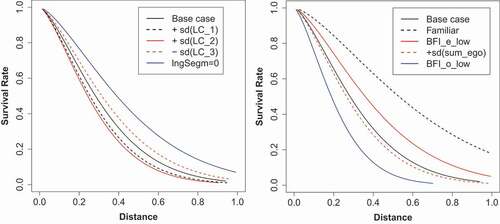