Figures & data
Figure 1. Location map of the study area in the south-western part of India. The ward map shows the administrative wards of Pune city. The pan-sharpened Quickbird image is from the central part of the city.
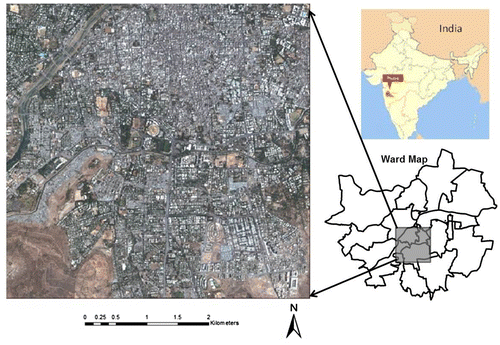
Figure 2. Slum delineations provided by a local NGO (MASHAL) displayed on the Quickbird image of Pune city.
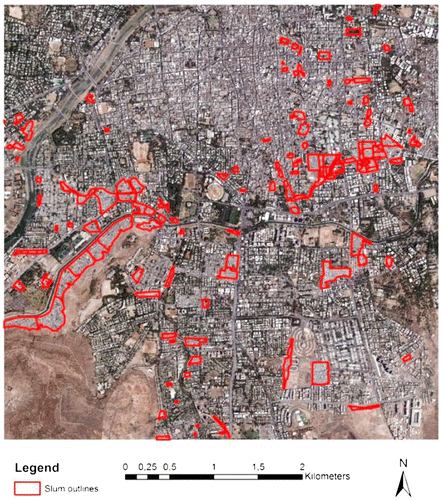
Table 1. Adaptation of GSO concepts to an ontology for Pune with OOA parameterisation at environs, settlement and object levels.
Table 2. Definitions of the parameters used in the classification. For details, refer to Trimble (Citation2014).
Figure 4. Settlement primitives at Level 2 using texture layer GLCM contrast: (a) highly dense buildings in slums outlined and displaying a clear contrast to neighbouring segments; (b) planned area with segments comprising different land cover features.
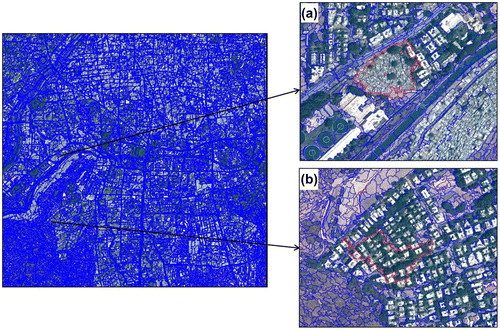
Table 3. Ruleset summary and the quantitative classification criteria with the sequence from top to bottom.
Figure 5. Classification of image at Level 1: (a) magnified view of a classified slum area; (b) segmentation result of the same area.
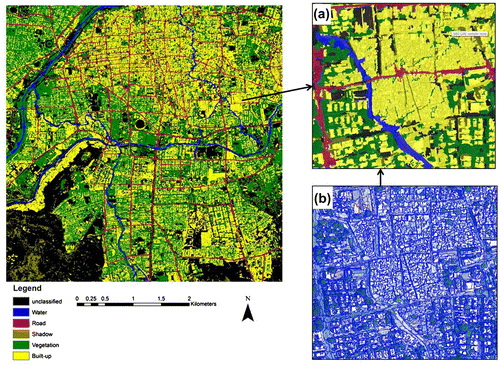
Figure 6. Final classified map of the study area with slums, ground pictures and corresponding zoomed views of the image: (a) ground photograph of buildings in the Old City; (b) ground photograph of a typical slum settlement; (c) magnified view of a part of the Old City misclassified as slum on the final map; (d) a typical slum settlement.
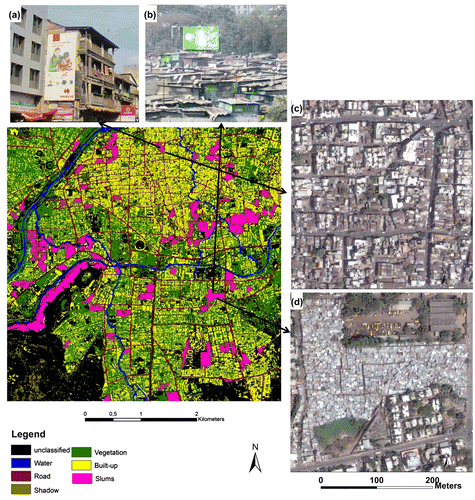
Table 4. The producer’s and user’s accuracies of the land cover classes at Level 1.
Figure 7. Figure showing sources of error in classification resulting from: (a) generalisation; (b) political/administrative definition; (c) vegetation cover; (b) false positives from the Old City.
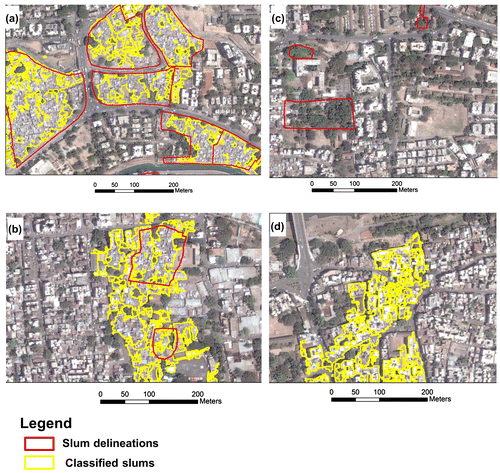