Figures & data
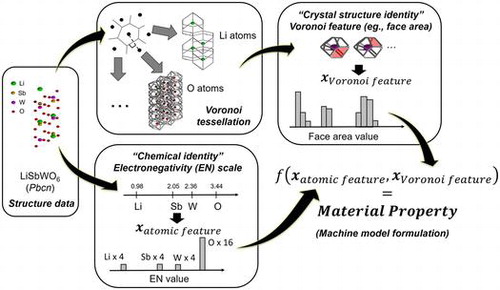
Figure 1. Example of feature binning procedure for (a) electronegativity and (b) Voronoi feature face area (in red) in Pbcn LiSbWO6 with 4 Li, 4 Sb, 4 W, and 16 O atoms in the unit cell.
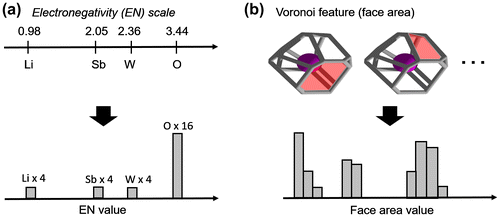
Table 1. Applied smoothing parameters for various histograms.
Figure 2. (a) Schematic workflow for the extraction procedure implemented for Voronoi tessellation of crystal structures, (b) Test case crystal structure Pbcn LiSbWO6 (green spheres are Li atoms, brown spheres are Sb atoms, magenta spheres are W atoms, and red spheres are O atoms). Voronoi tessellation for LiSbWO6 (c) with all atoms considered (1st nearest-neighbor (NN) information), (d) with Li atoms only (2NN information), and (e) with O atoms only (2NN information).
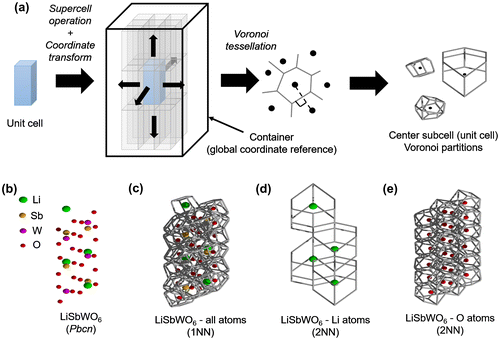
Table 2. Fitting conditions employed for GBR and SVR models.
Figure 3. Test data-set grand average errors for DFT-CE and DFT-BG by (a), (c) GBR (total: 420 trees/data split × 100 data splits = 4200 fitting instances for CE and BG, respectively) and (b), (d) SVR fitting. Test data-set error surface with respect to hyperparameter combination (C and ) for SVR fitting for (c) DFT-CE and (d) DFT-BG; a total of 18,600 regularly-spaced hyperparameter coordinate sets (or fitting instances) each, respectively.
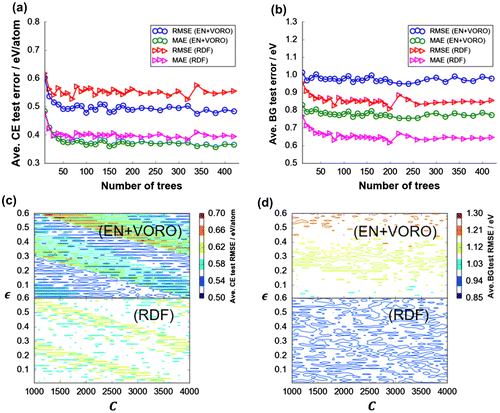
Figure 4. Fitting quality of final models: (a) CE with GBR, (b) CE with SVR, (c) BG with GBR, and (d) BG with SVR. Insets in (a) and (c) show deviance (error residuals) plots terminating at the optimal number of base tree learners for GBR fitting (50 trees for CE and 70 trees for BG, respectively). Optimal hyperparameters (C and ) for SVR fitting are indicated in (b) and (d).
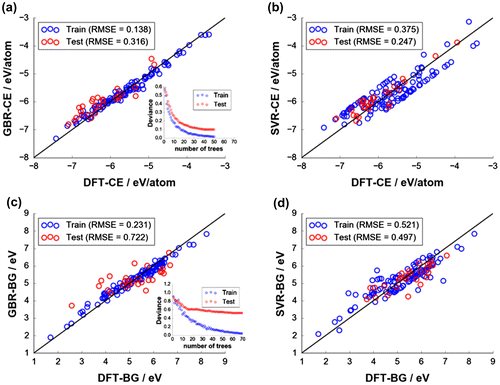
Table 3. Comparison of predictive accuracy between EN+VORO and RDF-based final machine models with ICSD-based DFT calculated data-set (140 compounds).
Figure 5. Test data-set grand average errors (from 100 random data splitting) for (a) DFT-CE, (c) DFT density (DFT-d), (e) DFT-BG, and (g) DFT decomposition energy (DFT-Ed) by GBR fitting. Final models have (b) 120, (d) 160, (f) 300, (h) 430 trees, respectively.
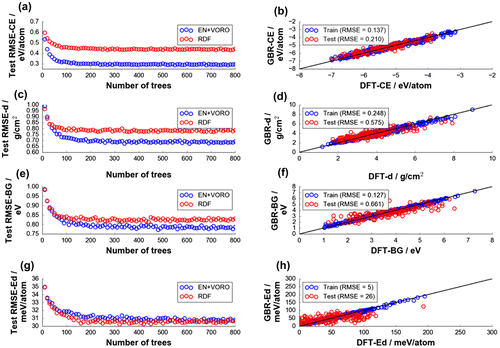
Table 4. Comparison of predictive accuracy between EN+VORO- and RDF-based final machine models using Materials Project data-set (1000 compounds).
Figure 6. Scaled variable importance (VI) plot of bin features from the final GBR models for (a) DFT-CE and (b) DFT-BG.
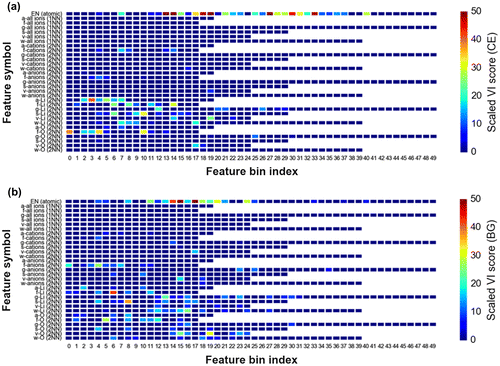
Figure 7. (a) Average number of vertices vs. counted average face area <0.2 from Voronoi tessellations of atom centers, (b) histogram for face areas relative to average per Voronoi cell for Pbcn LiSbWO6 crystal structure.
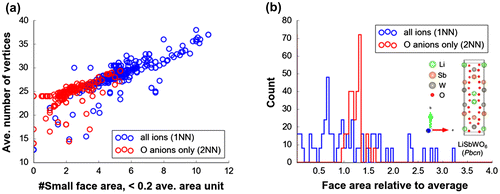