Figures & data

Figure 1. Three examples of artificially measured spectra with Gaussian noise. The solid line is the true curve and the dots are the artificially measured spectral data: (a)
and
, (b)
and
, and (c)
and
.
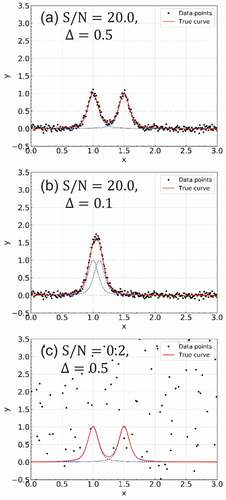
Table 1. Settings of the inverse temperature corresponding to Eq. (18).
Figure 2. (a)–(c) Results of model selection by Bayesian estimation respectively corresponding to spectral data in )–(c).
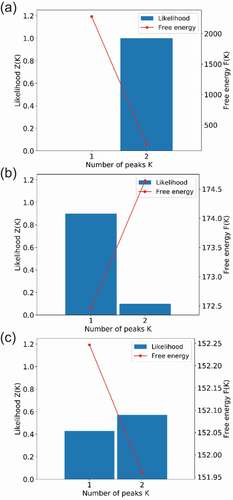
Figure 3. Results of model selection for various peak-to-peak distances and S/N ratios. The values in the figure indicate the posterior probability
for
.
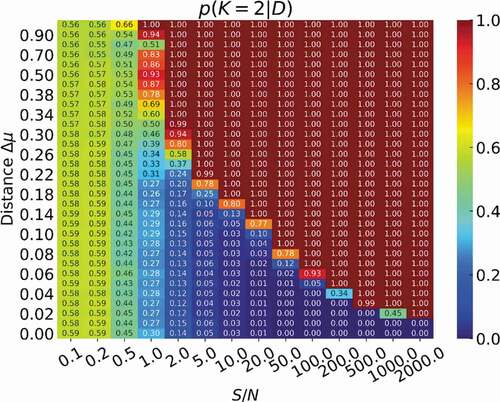
Figure 4. Posterior distribution of each parameter when Bayesian estimation is performed on the spectral data in ). Dashed lines indicate the true parameter values used to generate the spectral data.
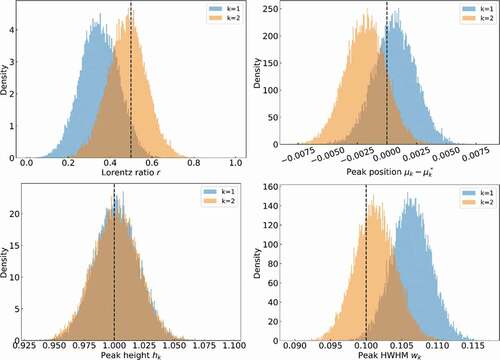
Figure 5. STD of the posterior distribution as a function of the S/N ratio for Δ = 0.5. Triangles and inverted triangles are respectively the STDs of the parameters for the first and second peaks.
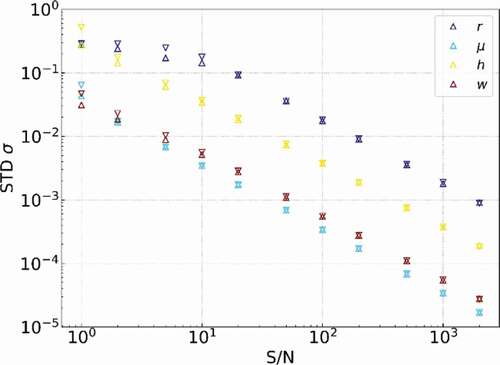
Figure 6. STD as a function of the peak-to-peak distance for S/N = 100.0. Triangles and inverted triangles are respectively the STDs of the parameters for the first and second peaks.
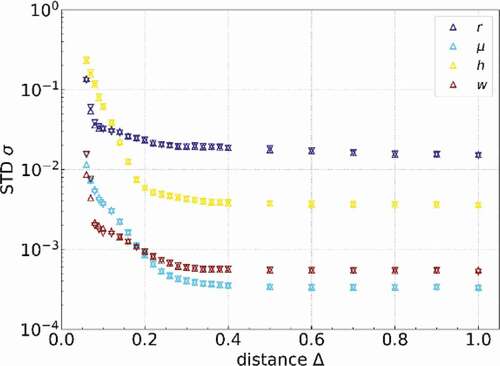
Table 2. Values of the fitted parameters 2 in Eq. (19).
Figure 7. Schematic diagrams of values obtained using EquationEq. (19)(A-19)
(A-19) . (a) Scaled STD
as a function of S/N
for
and
, where the peak-to-peak distance
is sufficiently large:
and
. (b)
as a function of the peak-to-peak distance
for several conditions of
when we set the prefactor of EquationEq. (19
(A-19)
(A-19) )
.
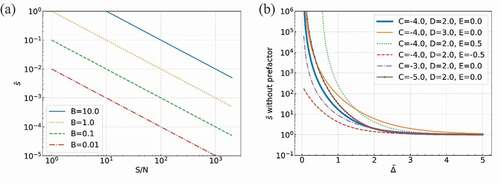
Figure 8. Results of regression using the fitting function in EquationEq. (19)(A-19)
(A-19) of the STDs of the posterior distributions for each parameter. Parameters are the (a) Lorentz–Gauss mixing ratio
, (b) peak position
, (c) peak height
, and (d) HWHMs of the peaks
.
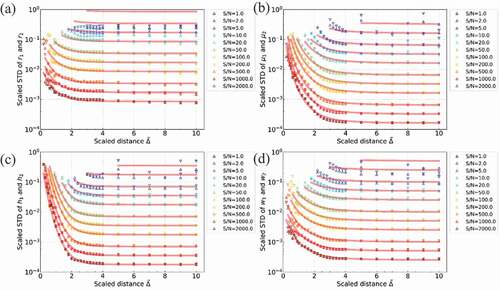
Figure 9. Fitted spectra from Bayesian estimation (a) and BIC-fitting (b) for the experimental valence spectrum of SiO2. Open circles are the experimental spectrum, the orange line is the fitted spectrum, the green line is the background, and the black lines are all peaks above the background.
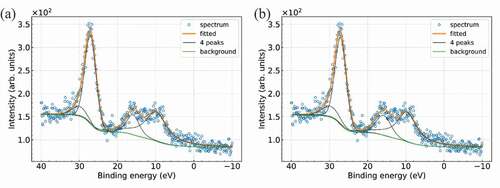
Figure 10. Results of model selection through Bayesian estimation (a) and BIC-fitting (b) for the experimental valence spectrum of SiO2. The red circle in (b) indicates the model with the minimum BIC.
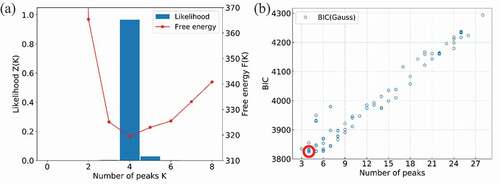
Table 3. Calculated confidence intervals of the posterior distribution for all peak parameters and those estimated using Eq. (19).
Figure 12. Posterior probability densities of peak parameters for two peaks located at about
and
eV for the valence spectrum of SiO2.
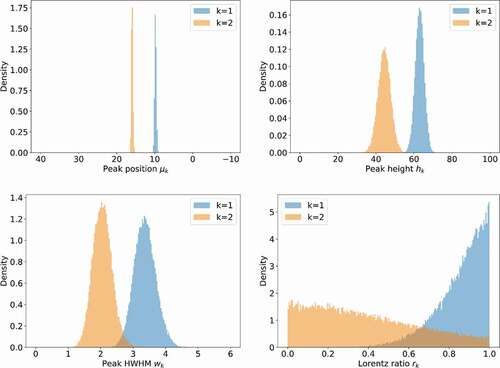
Figure A-1. Results of model selection and posterior distributions and
. (a)–(e) only differ in the initial random seeds used in generating the spectral data sets under the same conditions as those in ).
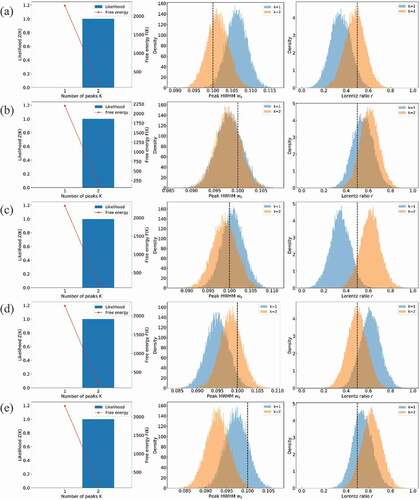
Figure A-2. Two-dimensional distribution of parameters . The diagonal components of the figure show the histogram of the posterior distribution of each parameter. The lower off-diagonal components are two-dimensional distributions, whereas the upper off-diagonal components show the coefficients of correlation between two parameters. The dotted lines indicate the true parameter values used to generate the spectral data.
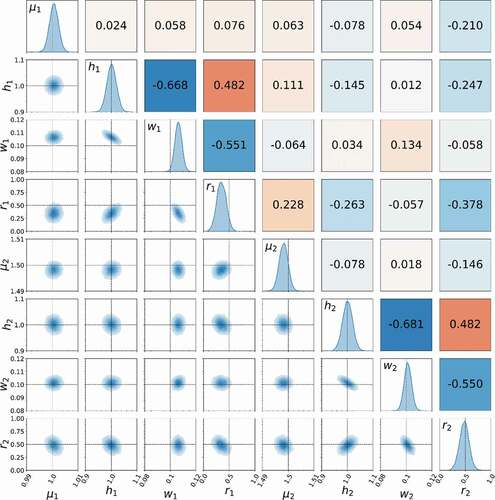
Figure E-1. Two examples of artificial spectra that have two peaks with different heights: (a) , (b)
. The other parameters are the same. Open circles are the artificial spectrum, the orange line is the fitted spectrum, and the black lines are the peak components.
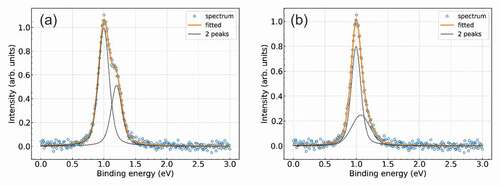
Figure E-2. Posterior distribution of each parameter when Bayesian estimation is performed on the spectral data in . The dashed lines indicate the true parameter values used to generate the spectral data.
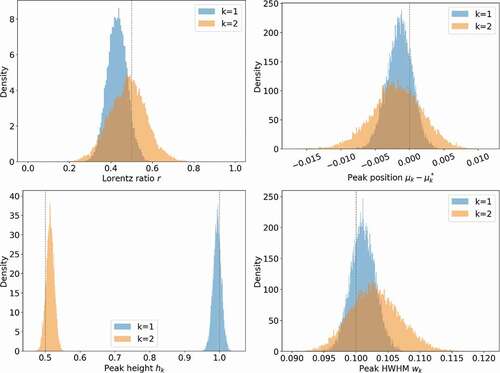