Figures & data
Table 1. Information sensitivity states of companies.
Figure 3. The evolution of the number of information sensitive companies in time.
Note: The following events are displayed with vertical lines. FED emergency meeting in March 14th, 2008: The Federal Reserve board had an emergency weekend meeting regarding Bear Stearns. Lehman Bankruptcy in September 15th, 2008: Lehman Brothers filed for bankruptcy. Greek election in June 17th, 2012: The centre-right wins legislative elections in Greece. Draghi Speech in July 26th, 2012: ECB president Mario Draghi gives the famous ‘the ECB is ready to do whatever it takes to preserve the euro’ - speech. COVID-19 in February 11th, 2020: WHO names the new virus as COVID-19.
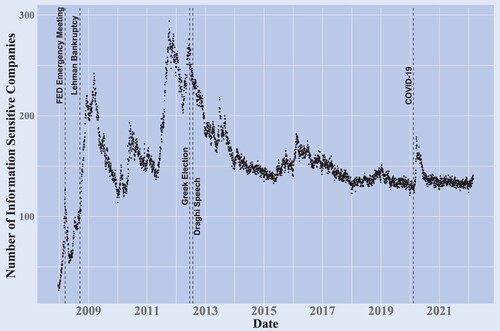
Figure 4. Hierarchical clustering of topics. The dendrogram plots the result of a hierarchical clustering model estimated with the topic word-distributions.
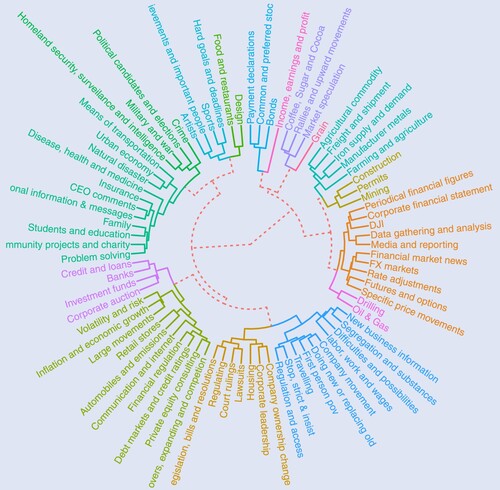
Figure 5. Prevalence of news topics in Time. The figure plots the topic distributions of each topic k aggregated to a monthly level across the period 1890–2022.
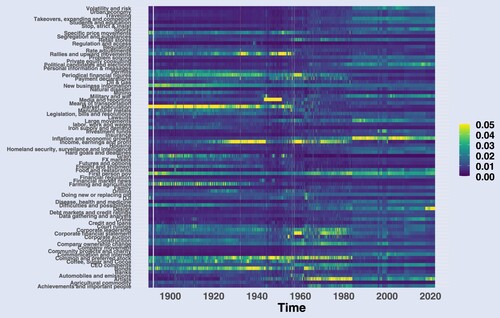
Figure 6. Evolution of unexpected news in selected topics. The figure plots the unpredictable part of topic's daily prevalence for each topic aggregated to a monthly level across the period 2006–2022.
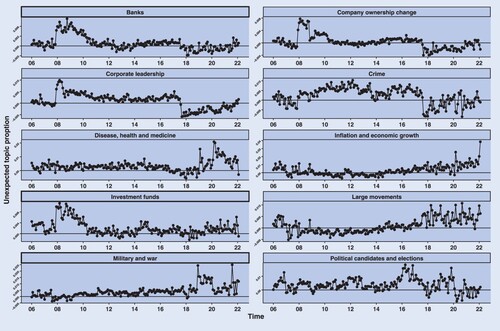
Figure 7. Evolution of unexpected news in time. The figure plots the unpredictable part of topic's daily prevalence for each topic k aggregated to a monthly level across the period 2006–2022.
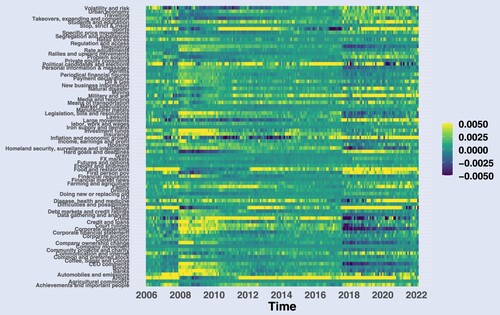
Figure 8. Distribution of the primordial - conceptual word share difference across authors. A total amount of 3062 authors and 74 articles per author on average. (a) Mean of . (b) SD of
and (c) Autocorrelation of
.
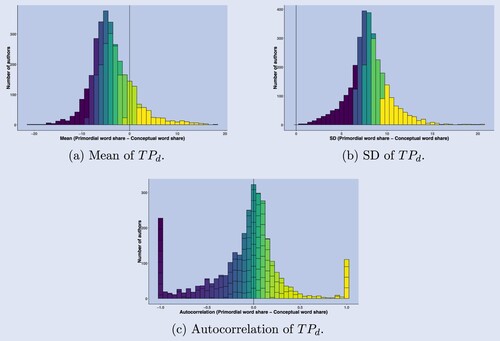
Figure 9. Primordial-conceptual word share difference across time. (a) Daily thinking process leaning across time. The figure plots the 25th and 75th (shaded area) quantiles and the average for each year in the period 1890–2022 and (b) Monthly correlation of topics and primordial - conceptual word share difference across time and topics.
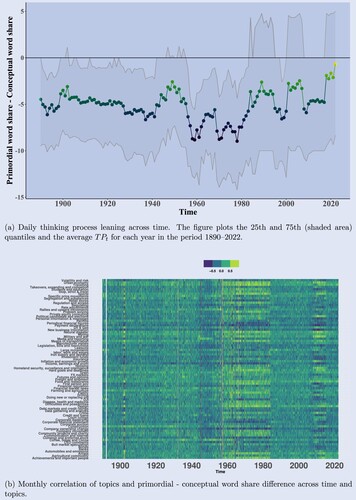
Figure 10. Narrative triggers of information sensitivity. The figure plots the coefficients of equation (Equation8
(8)
(8) ) with 95% confidence intervals for topics with a positive and significant last period coefficients and at least 15 coefficients that are statistically significant at a 5% level between 1–30 day horizons. Statistical significance is calculated with standard errors clustered at the day and company level.
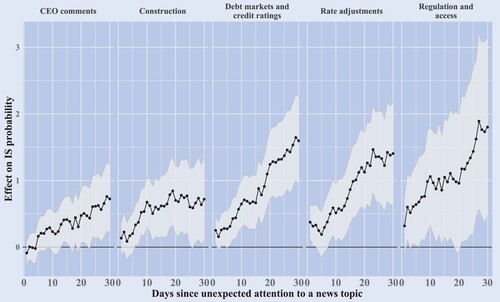
Figure 11. Individual company attention and narrative triggers of information sensitivity. The figure plots the and the
coefficients of equation (Equation8
(8)
(8) ) with 95% confidence intervals for topics with a positive and significant last period coefficients and at least 15 coefficients that are statistically significant at a 5% level between 1–30 day horizons. Statistical significance is calculated with standard errors clustered at the day and company level.
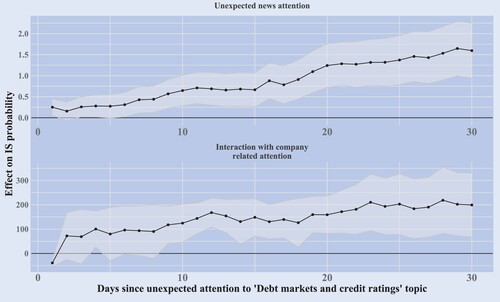
Figure 12. Narrative triggers of information sensitivity and different economic states. The figure plots the coefficients of equation (Equation8
(8)
(8) ) with 95% confidence intervals for topics with positive and significant last period coefficient and at least 15 coefficients in total that are positive and statistically significant at a 5% level between 1–30 day horizons in at least one state. Statistical significance is calculated with standard errors clustered at the day and company level. The strong (red) coefficients refer to coefficients in the economically strong state and the weak (blue) refer to coefficients in the economically weak state.
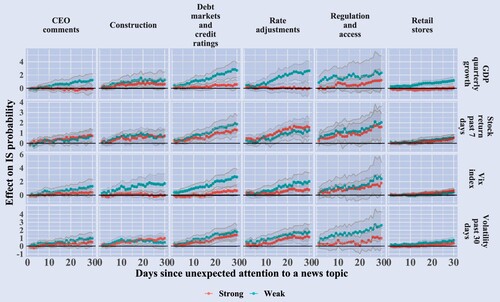
Figure 13. Narrative triggers of information sensitivity and different economic states. The figure plots the coefficients of equation (Equation8
(8)
(8) ) with 95% confidence intervals for selected group of topics for which the coefficients are not statistically significant at a 5% level between 1–30 day horizons. Statistical significance is calculated with standard errors clustered at the day and company level. The strong (red) coefficients refer to coefficients in the economically strong state and the weak (blue) refer to coefficients in the economically weak state.
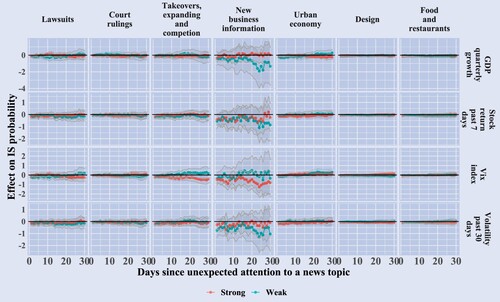
Figure 14. Journalists' thinking process-related language and narrative triggers of information sensitivity. The figure plots the coefficients of equation (Equation11
(11)
(11) ) with 95% confidence intervals for statistically significant trigger topics where the last period, and at least 15
coefficients overall, are statistically significant at a 5% level between 1–30 day horizons. Statistical significance is computed with standard errors clustered at the day and company level.
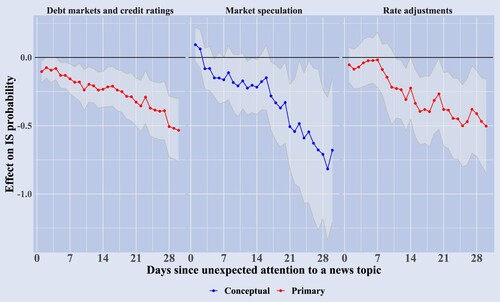
Table A1. Topic labels and the predictability of the attention to topics 1–40.
Table A2. Topic labels and the predictability of the attention to topics 41–80.
Data availability statement
The paper uses text data from ProQuest historical newspapers. The actual text data is proprietary and it cannot be shared publicly. The data collection procedure is documented in detail in the paper. The website for ProQuest Historical newspapers is https://about.proquest.com/en/products-services/pq-hist-news/https://about.proquest.com/en/products-services/pq-hist-news/. One needs to have a payed subscription to the database to get access to the actual text data. In addition, the CDS spread data is proprietary and it cannot be shared publicly. It has been collected from Refinitiv Datastream and one needs to have a payed subscription to the database to get access to the CDS data.