Figures & data
Figure 1. The schematic hyperplane of SVM separating positive class (+1) and negative class (−1) with the maximum margin.
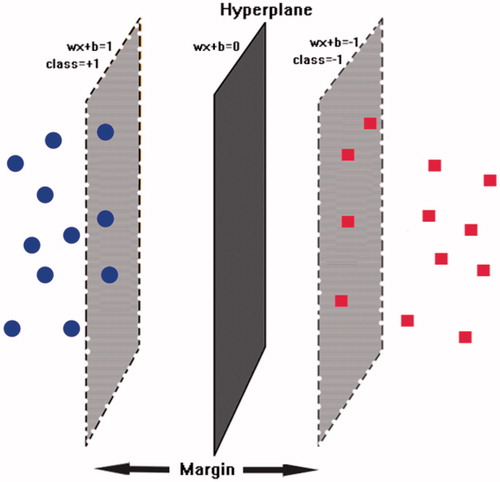
Table 1. The 52 molecular descriptors filtered by GA-SVM method for building SVM model
Table 2. The evaluation and validation result of the ten-fold cross-validation and independent test.
Figure 2. (A) The barcodes and letter mode of the amino acids interaction fingerprint generated by MOE2016 software. (B) The best pharmacophore models evaluated by training set, the purple and cyan features indicted the hydrogen-bond donor and acceptor respectively. (C) The matching of the pharmacophore with the superposed structures indicates that the pharmacophore can describe the superposition characteristics of the substituent of the 66 CDK2 inhibitors well.
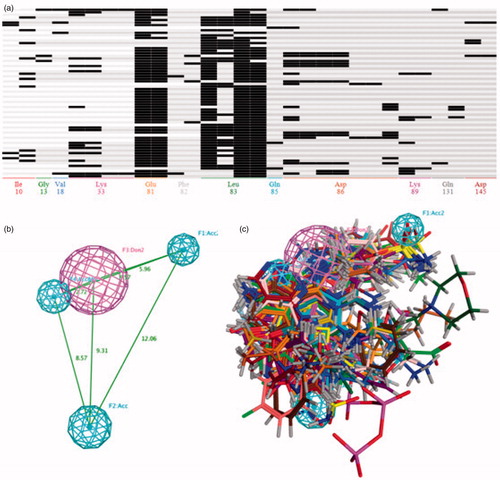
Table 3. The evaluation and validation result of the four Pharmacophore models generated by PLIF.
Table 4. The RMSD values of the eight active compounds between their docking conformation result and build-in ligand in CDK2 inhibitors.
Table 5. Validation and evaluation the various virtual screening method by the validation set that contains 375 known CDK2 inhibitors and 155528 decoys.
Figure 3. The six compounds with novel scaffolds obtained from the multistage virtual screening method.
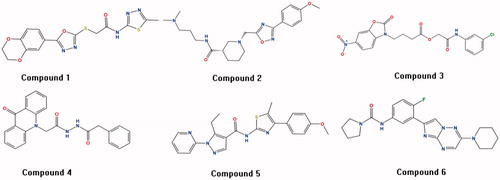
Figure 4. The molecular dynamics results of the Milciclib and six screened compounds, the RMSD of Milciclib-CDK2 complex was painted in red, the six screened compounds were painted in blue in (A–F).
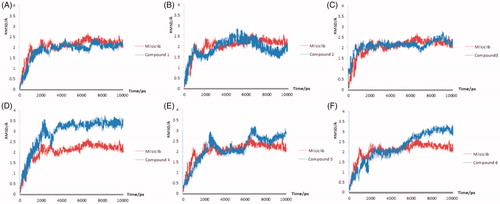
Table 6. Binding free energy of the Milciclib and six potential CDK2 inhibitors.
Figure 5. The interaction between the compounds and the amino acid residues in the CDK2 active pockets during the molecular dynamics simulation (Compound 1 in 0 ns and 5 ns was showed in (A and B), Compound 3 in 0 ns and 5 ns was showed in (C and D).
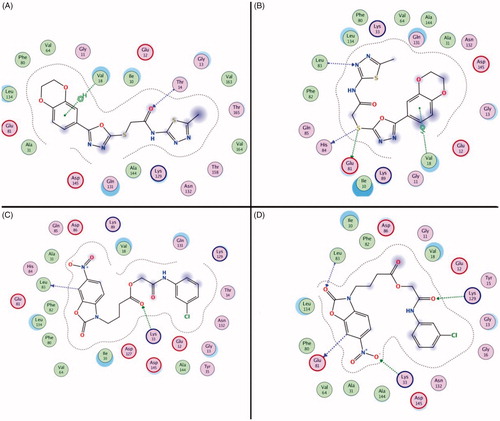
Table 7. The CDKs inhibition activity of Milciclib and two hit compounds