Figures & data
Figure 1. The secondary plot of apparent kinetic parameters ratio appKM/appkcat versus [I] for the EGCG inhibition on HNE reduction. The dotted line refers to linear regression analysis fixing the intercept with the y-axis at the appKM/appkcat control value. Each value represents the appKM/appkcat ratio evaluated at the indicated EGCG concentrations. Data were obtained through non-linear regression analysis of vo vs [S] measurements using the Michaelis Menten equation. Bars (when not visible are within the symbols size) represent the standard error of the measurements.
![Figure 1. The secondary plot of apparent kinetic parameters ratio appKM/appkcat versus [I] for the EGCG inhibition on HNE reduction. The dotted line refers to linear regression analysis fixing the intercept with the y-axis at the appKM/appkcat control value. Each value represents the appKM/appkcat ratio evaluated at the indicated EGCG concentrations. Data were obtained through non-linear regression analysis of vo vs [S] measurements using the Michaelis Menten equation. Bars (when not visible are within the symbols size) represent the standard error of the measurements.](/cms/asset/3c8c5a25-12e2-42f5-adca-92925d03e624/ienz_a_2076089_f0001_b.jpg)
Figure 2. Inhibition models of an enzyme (E): a general model of inhibition (Panel A) and an alternative model of inhibition following “the classical approach” (Panel B). (S): substrate; (I): inhibitor; (ES): enzyme-substrate complex; (EI): enzyme-inhibitor complex; (EIS): enzyme-inhibitor-substrate ternary complex; k+1, k−1, k+2, k+3, k−3, k+4 refer to kinetic constants; Ki and K’i refer to EI and EIS dissociation constants, respectively. See text for details.
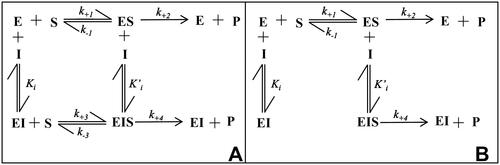
Figure 3. Incomplete uncompetitive inhibition. (Panel A) represents the dependence of appkcat on the increase of [I] according to EquationEquation (2)(2)
(2) ; (Panel B) represents the dependence of appkM on the increase of [I] according to EquationEquation (3)
(3)
(3) ; (Panel C) represents the dependence of appKM/appkcat on the increase of [I] according to EquationEquation (4)
(4)
(4) .
![Figure 3. Incomplete uncompetitive inhibition. (Panel A) represents the dependence of appkcat on the increase of [I] according to EquationEquation (2)(2) kcatapp=Ki*k+2+k+4[I]Ki*+[I] (2) ; (Panel B) represents the dependence of appkM on the increase of [I] according to EquationEquation (3)(3) KMapp=Ki*KM+k+4k+1[I]Ki*+[I](3) ; (Panel C) represents the dependence of appKM/appkcat on the increase of [I] according to EquationEquation (4)(4) KMappkcatapp=Ki*KM+k+4k+1[I]Ki*k+2+k+4[I](4) .](/cms/asset/1d376f2d-6ad4-4116-aa3b-42c8f9683b28/ienz_a_2076089_f0003_c.jpg)
Figure 4. Predicted effect of the inhibitor concentration on the apparent kinetic parameters. The dependence of 1/appkcat (Panel A) and appKM/appkcat (Panel B) on the inhibitor concentration for different incomplete uncompetitive inhibition situations is reported, according to EquationEquations (2(2)
(2) and Equation4)
(4)
(4) , respectively. The following arbitrary values were fixed: k+2 = 1, k+1 = 100, KM = 0.5 and
= 0.001. Curves 1–6 refer to k+4 values of zero (complete inhibition), 0.02, 0.05, 0.1, 0.15 and 0.2, respectively.
![Figure 4. Predicted effect of the inhibitor concentration on the apparent kinetic parameters. The dependence of 1/appkcat (Panel A) and appKM/appkcat (Panel B) on the inhibitor concentration for different incomplete uncompetitive inhibition situations is reported, according to EquationEquations (2(2) kcatapp=Ki*k+2+k+4[I]Ki*+[I] (2) and Equation4)(4) KMappkcatapp=Ki*KM+k+4k+1[I]Ki*k+2+k+4[I](4) , respectively. The following arbitrary values were fixed: k+2 = 1, k+1 = 100, KM = 0.5 and Ki* = 0.001. Curves 1–6 refer to k+4 values of zero (complete inhibition), 0.02, 0.05, 0.1, 0.15 and 0.2, respectively.](/cms/asset/2df4caaf-3e91-4cc8-bc83-d8b847b37c05/ienz_a_2076089_f0004_c.jpg)
Figure 5. Predicted effect of the inhibitor concentration on the apparent kinetic parameters. The dependence of 1/appkcat (Panels A and D), appKM (Panels B and E) and appKM/appkcat (Panels C and F) on the inhibitor concentration for different incomplete mixed inhibition situations is reported, according to EquationEquations (6–8), respectively. The following arbitrary values were fixed: k+2 = 1, k+1 = 100, KM = 0.5. Curves 1–6 refer to k+4 values of zero (complete inhibition), 0.02, 0.05, 0.1, 0.15 and 0.2, respectively. (Panels A–C) refer to and Ki values of 0.001 and 0.01, respectively. (Panels D–F) refer to
and Ki values of 0.01 and 0.001 respectively. In (Panels B and E), curves 1–6 are essentially superimposable. Having imposed a reasonable rather low value of k+2/k+1 (0.01), k+4/k+1 will be negligible making the curves of appKM versus [I] at different k+4 are essentially superimposable.
![Figure 5. Predicted effect of the inhibitor concentration on the apparent kinetic parameters. The dependence of 1/appkcat (Panels A and D), appKM (Panels B and E) and appKM/appkcat (Panels C and F) on the inhibitor concentration for different incomplete mixed inhibition situations is reported, according to EquationEquations (6–8), respectively. The following arbitrary values were fixed: k+2 = 1, k+1 = 100, KM = 0.5. Curves 1–6 refer to k+4 values of zero (complete inhibition), 0.02, 0.05, 0.1, 0.15 and 0.2, respectively. (Panels A–C) refer to Ki* and Ki values of 0.001 and 0.01, respectively. (Panels D–F) refer to Ki* and Ki values of 0.01 and 0.001 respectively. In (Panels B and E), curves 1–6 are essentially superimposable. Having imposed a reasonable rather low value of k+2/k+1 (0.01), k+4/k+1 will be negligible making the curves of appKM versus [I] at different k+4 are essentially superimposable.](/cms/asset/55ba230d-8b8a-4509-8358-8295bdfe90ed/ienz_a_2076089_f0005_c.jpg)
Figure 6. Inhibition model of an enzyme (E) following “the non-classical approach”. (S): substrate; (I): inhibitor; (ES): enzyme-substrate complex; (EI): enzyme-inhibitor complex; (EIS): ternary enzyme-inhibitor-substrate complex; k+1, k−1, k+2, k+3, k−3, k+4 are kinetic constants; Ki is the EI complex dissociation constant. See text for details.
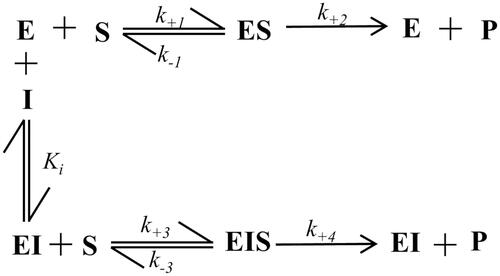
Figure 7. Non-classical approach for complete inhibition. (Panel A) represents the dependence of appKM on the increase of [I] according to EquationEquation (10)(10)
(10) ; (Panel B) represents the dependence of 1/appkcat on the increase of [I] according to EquationEquation (11)
(11)
(11) ; Panel C represents the dependence of appKM/appkcat on the increase of [I] according to EquationEquation (12)
(12)
(12) .
![Figure 7. Non-classical approach for complete inhibition. (Panel A) represents the dependence of appKM on the increase of [I] according to EquationEquation (10)(10) KMapp=kM(1+[I]Ki)1+[I]kMKiKM′ (10) ; (Panel B) represents the dependence of 1/appkcat on the increase of [I] according to EquationEquation (11)(11) kcatapp=KiKM′k+2KiKM′+kM[I](11) ; Panel C represents the dependence of appKM/appkcat on the increase of [I] according to EquationEquation (12)(12) KMappkcatapp=KMk+2+KMKik+2[I] (12) .](/cms/asset/f8238889-4083-41e8-832d-1f0dbba978bc/ienz_a_2076089_f0007_c.jpg)
Figure 8. Non-classical approach for incomplete inhibition. (Panel A) represents the dependence of appKM on the increase of [I] according to EquationEquation (14)(14)
(14) ; (Panel B) represents the dependence of 1/appkcat on the increase of [I] according to EquationEquation (15)
(15)
(15) ; (Panel C) represents the dependence of appKM/appkcat on the increase of [I] according to EquationEquation (16)
(16)
(16) .
![Figure 8. Non-classical approach for incomplete inhibition. (Panel A) represents the dependence of appKM on the increase of [I] according to EquationEquation (14)(14) KMapp=KiKMKM′+KMKM′[I]KM′Ki+KM[I](14) ; (Panel B) represents the dependence of 1/appkcat on the increase of [I] according to EquationEquation (15)(15) kappcat= k+2KM′Ki+k+4KM[I]KM′Ki+ KM[I](15) ; (Panel C) represents the dependence of appKM/appkcat on the increase of [I] according to EquationEquation (16)(16) KMappkappcat=KiKMKM′+KMKM′[I]k+2KM′Ki+k+4KM[I](16) .](/cms/asset/efcd6220-73d8-40be-849b-905f816d7db4/ienz_a_2076089_f0008_c.jpg)
Figure 9. Kinetic analysis by the classical approach of EGCG inhibition of the AKR1B1-dependent reduction of HNE. (Panel A) Hanes-Wolff representation of rate measurements of HNE reduction in the presence of the indicated concentrations of EGCG. Bars (when not visible are within the symbols size) represent the standard deviations of the means from at least three independent measurements. (Panels B–D) secondary plots of apparent kinetic parameters at different [I] derived from the non-linear regression Michaelis-Menten analysis of primary kinetic data. Bars (when not visible are within the symbols size) represent the standard error of the measurements. The parameters appKM, 1/appkcat and appKM/appkcat versus [I] were interpolated by non-linear regression analysis according to EquationEquations (4)(6)
(6) , Equation(6)
(7)
(7) and Equation(7)
(4)
(4) , respectively. See the text for details.
![Figure 9. Kinetic analysis by the classical approach of EGCG inhibition of the AKR1B1-dependent reduction of HNE. (Panel A) Hanes-Wolff representation of rate measurements of HNE reduction in the presence of the indicated concentrations of EGCG. Bars (when not visible are within the symbols size) represent the standard deviations of the means from at least three independent measurements. (Panels B–D) secondary plots of apparent kinetic parameters at different [I] derived from the non-linear regression Michaelis-Menten analysis of primary kinetic data. Bars (when not visible are within the symbols size) represent the standard error of the measurements. The parameters appKM, 1/appkcat and appKM/appkcat versus [I] were interpolated by non-linear regression analysis according to EquationEquations (4)(6) kcatapp=Ki*k+2+k+4[I]Ki*+[I] (6) , Equation(6)(7) KMapp=KMKi*+(Ki*KiKM+k+4k+1)[I]+k+4Kik+1[I]2Ki*+[I] (7) and Equation(7)(4) KMappkcatapp=Ki*KM+k+4k+1[I]Ki*k+2+k+4[I](4) , respectively. See the text for details.](/cms/asset/bbcccd58-c4c3-4f53-b7b9-fe5231aeecff/ienz_a_2076089_f0009_b.jpg)
Figure 10. Kinetic analysis by the classical approach of EGCG inhibition of the AKR1B1-dependent reduction of L-idose. (Panel A) Hanes-Wolff representation of rate measurements of L-idose reduction in the presence of the indicated concentrations of EGCG. Bars (when not visible are within the symbols size) represent the standard deviations of the means from at least three independent measurements. (Panels B–D) secondary plots of apparent kinetic parameters at different [I] coming from the non-linear regression Michaelis-Menten analysis of primary kinetic data. Bars (when not visible are within the symbols size) represent the standard error of the measurements. The parameters appKM, appkcat and appKM/appkcat versus [I] were interpolated by non-linear regression analysis through EquationEquations (4)(6)
(6) , Citation(6) and Citation(7), respectively.
![Figure 10. Kinetic analysis by the classical approach of EGCG inhibition of the AKR1B1-dependent reduction of L-idose. (Panel A) Hanes-Wolff representation of rate measurements of L-idose reduction in the presence of the indicated concentrations of EGCG. Bars (when not visible are within the symbols size) represent the standard deviations of the means from at least three independent measurements. (Panels B–D) secondary plots of apparent kinetic parameters at different [I] coming from the non-linear regression Michaelis-Menten analysis of primary kinetic data. Bars (when not visible are within the symbols size) represent the standard error of the measurements. The parameters appKM, appkcat and appKM/appkcat versus [I] were interpolated by non-linear regression analysis through EquationEquations (4)(6) kcatapp=Ki*k+2+k+4[I]Ki*+[I] (6) , Citation(6) and Citation(7), respectively.](/cms/asset/ff6748ce-e48a-4c9e-a72f-7024e53f35e3/ienz_a_2076089_f0010_b.jpg)
Figure 11. Kinetic analysis by the non-classical approach of EGCG inhibition of the AKR1B1-dependent reduction of L-idose. The parameters 1/appkcat, appKM and appKM/appkcat obtained from data in , were reported as a function of [I] and interpolated by non-linear regression analysis through EquationEquations (10)–(12), respectively. Bars (when not visible are within the symbols size) represent the standard error of the measurements.
![Figure 11. Kinetic analysis by the non-classical approach of EGCG inhibition of the AKR1B1-dependent reduction of L-idose. The parameters 1/appkcat, appKM and appKM/appkcat obtained from data in Figure 10(A), were reported as a function of [I] and interpolated by non-linear regression analysis through EquationEquations (10)–(12), respectively. Bars (when not visible are within the symbols size) represent the standard error of the measurements.](/cms/asset/4def16d7-8c5a-4b4b-97de-3ed61f69659c/ienz_a_2076089_f0011_b.jpg)
Figure 12. Kinetic analysis by the non-classical approach of EGCG inhibition of the AKR1B1-dependent reduction of HNE. The parameters 1/appkcat, appKM and appKM/appkcat obtained from data in , were reported as a function of [I] and interpolated by non-linear regression analysis through EquationEquations (14)–(16), respectively. Bars (when not visible are within the symbols size) represent the standard error of the measurements.
![Figure 12. Kinetic analysis by the non-classical approach of EGCG inhibition of the AKR1B1-dependent reduction of HNE. The parameters 1/appkcat, appKM and appKM/appkcat obtained from data in Figure 9(A), were reported as a function of [I] and interpolated by non-linear regression analysis through EquationEquations (14)–(16), respectively. Bars (when not visible are within the symbols size) represent the standard error of the measurements.](/cms/asset/b485db72-96f2-49f2-a751-cdef23a8b081/ienz_a_2076089_f0012_c.jpg)