Figures & data
Figure 1. (A) Summary of methods. Each row represents the method for predicting TFs in transdifferentiation which are CellNet, JSD and Mogrify. Each column represents the computational stages involved in the TFs set prediction which are input requirement, generation of differential expression profile, identifying the influence of each TF in cell conversion, criteria to prioritize the TFs and finally the predictions. CellNet classifies the source cell-type based on the user experimental microarray data and provides a ranked list of TFs for conversion. It does this by creating GRN (gene regulatory network) from GEO (gene expression omnibus) data using CLR (context likelihood of relatedness) algorithm and the target cell specific sub-network is obtained by GSA (gene set analysis). The TF influence score is calculated by the differential expression of the TF and the number of genes it regulates. Finally, CellNet outputs classification of the source cell, a list of TFs ranked by their importance in conversion and the target cell-type GRN. As input JSD method requires only the target cell-type detail. The differential expression profile is detected by comparing the target cell profile with the background data set (selected by low Pearson correlation between the expression profiles) using microarray data from GEO. Jensen-Shannon divergence (JSD) is used to measure the deviance of the observed (TF expression profile observed in data from GEO) from the ideal (TF is highly expressed only in target cell compared to the background cells) distribution. Finally, it provides a ranked list of TFs based on the JSD score and specifies top 10 as core candidate TFs. As input Mogrify method requires both source and target cell-type details. The differential expression profile between target cell-type and background dataset (all cell-types excluding the target cell-type) is obtained using the CAGE data (cap analysis of gene expression) from FANTOM5 consortium. A gene interaction network is formed with data from STRING database and MARA. Then the TFs are ranked based on differential expression of the TF, differential expression of the regulated genes and connectivity of the TF in the network. Finally, TFs expressed in the source cell are removed and a non-redundant TFs set is provided. B) Comparison of TFs used for prediction by each method and TFClass which comprises of hierarchical classification of TFs. C) Comparison of target cell-types available for the conversion from fibroblast by each method.
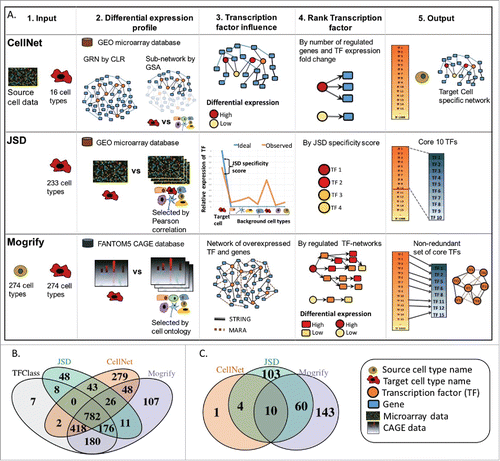
Figure 2. Subset of cell-type ontology tree of transdifferentiation. Cell-types available for transdifferentiation from fibroblast by each method is mapped onto the UBERON cell-type ontology tree. The pie charts indicate which of the methods provide a prediction for the cell-type on the tree. The heatmap represents the overlap of the predicted TFs between methods (i.e. Mogrify and JSD is M:J, JSD and CellNet is J:C and CellNet and Mogrify is C:M). The table provides the set of TFs predicted by each method for conversion from fibroblast. If there are more than one predictions mapped to the cell-type node, then it is denoted by * and the number of asterisks indicates the number of addition.
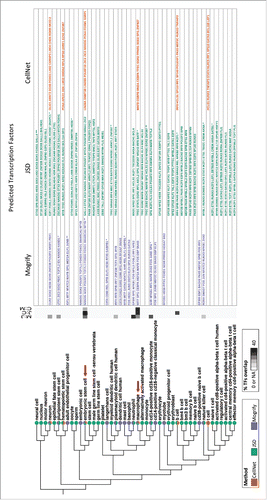
Figure 3. Fibroblast to heart conversion. Top 20 gene ontology biological processes (BPs) enriched in the predicted TFs network for each method (A) CellNet, (B) JSD and (C) Mogrify are given with the corresponding p-values. The method Mogrify is denoted as M, CellNet is denoted as C, and JSD is denoted as J. The common BP enriched between the methods for example CellNet, JSD and Mogrify is represented as C:J:M; and between Mogrify and CellNet is represented as M:C. The last column provides the TFs that enriches the same BP when individual TF along with the first neighbors in the network were used. The unique TFs predicted by each method are highlighted. BP terms related to regulation of transcription are written in gray and italics.
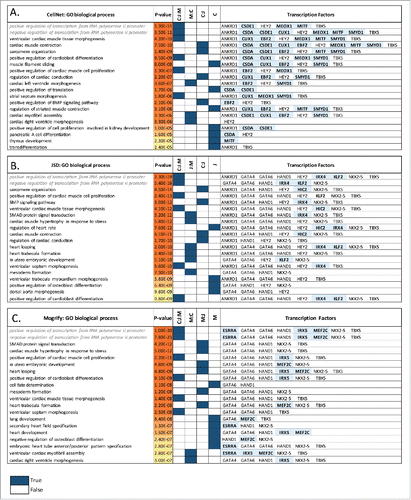
Table 1. Fibroblast to cardiomyocyte-like cells. Predicted TF sets to initiate conversion from fibroblast to heart. Those TFs shown in Bold are predicted by more than one technique and those underlined are predicted by all 3 methods.
Figure 4. Fibroblast to hepatocyte conversion. Top 20 gene ontology biological processes (BPs) enriched in the predicted TFs network for each method (A) CellNet, (B) JSD and (C) Mogrify are given with the corresponding p-values. The method Mogrify is denoted as M, CellNet is denoted as C, and JSD is denoted as J. The common BP enriched between the methods for example CellNet, JSD and Mogrify is represented as C:J:M; and between Mogrify and CellNet is represented as M:C. The last column provides the TFs that enriches the same BP when individual TF along with the first neighbors in the network were used. The unique TFs predicted by each method are highlighted. BP terms related to regulation of transcription are written in gray and italics.
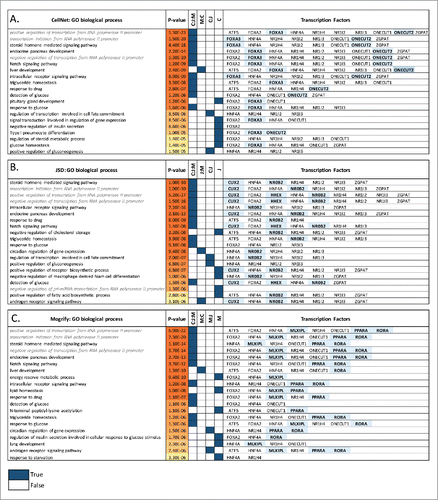
Table 2. Fibroblast to hepatocytes-like cells. Predicted TF sets to initiate conversion from fibroblast to hepatocyte. Those TFs shown in Bold are predicted by more than one technique and those underlined are predicted by all 3 methods.