Figures & data
Table 1. Summary table of network-wide day clustering.
Figure 1. Overview of the methodological framework for revealing the representative set of day-types Y with internal and external evaluation, including dimensionality reduction, day clustering. External evaluation process is highlighted.
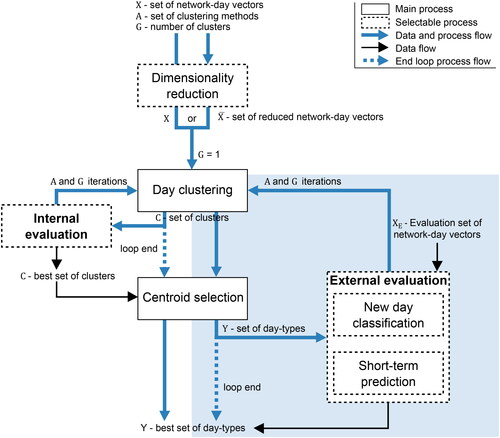
Table 2. Notation
Table 3. Sets of calendar-based clustering categories.
Figure 2. Case study of motorway control system sensors of Stockholm, Sweden active at least one day 2017-2018. The sensors with complete data in case study period are highlighted. (a) Complete network of active sensors. (b) Area around the city center. (c) Zoom-in illustration of the sensor density.
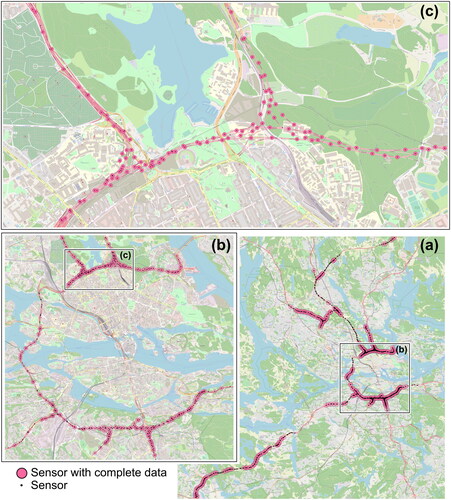
Table 4. Internal evaluation indices for calendar-based clusterings.
Figure 3. Calendar visualization of calendar-based clusterings for year 2017. White cells are days with missing data.
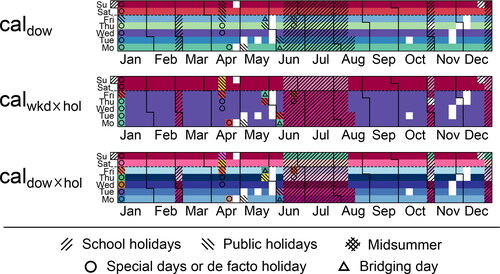
Figure 4. Calendar visualization of data-driven clustering methods clustered on original dataset White cells are days with missing data.
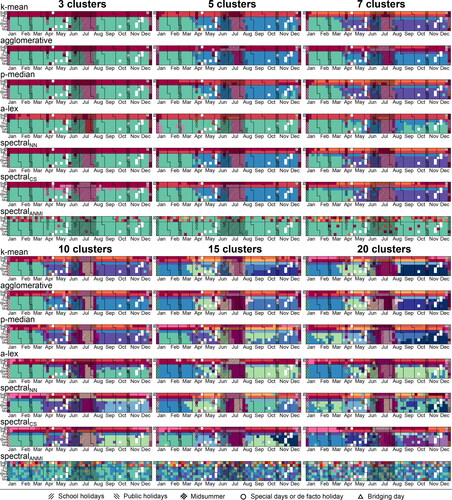
Figure 5. Day-type centroids for p-median and a-lex with 10 clusters. (a) Calendar visualization (b) Aggregated network-wide day-time profiles. (c,d) Space-time matrices of flows across all sensors in the network and all considered time intervals. (c) for p-median and (d) for a-lex.
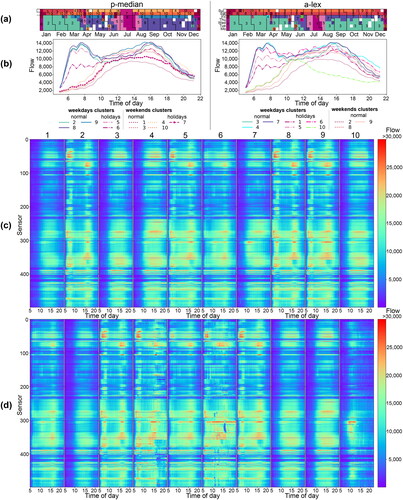
Figure 6. Total within-cluster variance (TWCV) as a function of the number of clusters across clustering methods. (a) is the original dataset and (b) the reduced dataset
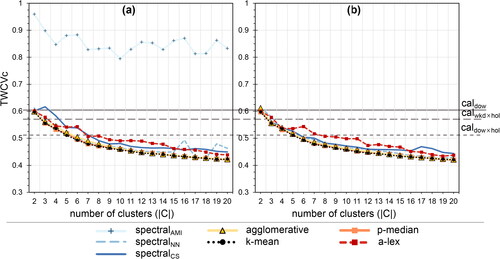
Figure 7. Total cluster dissimilarity (TCD) as a function of the number of clusters across clustering methods. (a) is the original dataset and (b) the reduced dataset
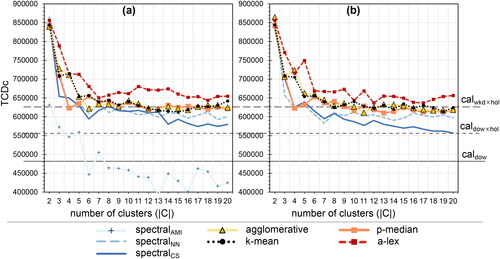
Figure 8. Silhouette score (SC) as a function of the number of clusters across clustering methods. (a) is the original dataset and (b) the reduced dataset
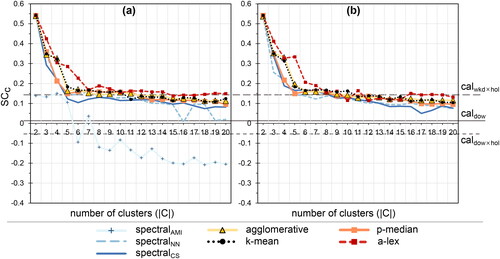
Figure 9. Davies-Bouldin (DB) index as a function of the number of clusters across clustering methods. (a) is the original dataset and (b) the reduced dataset
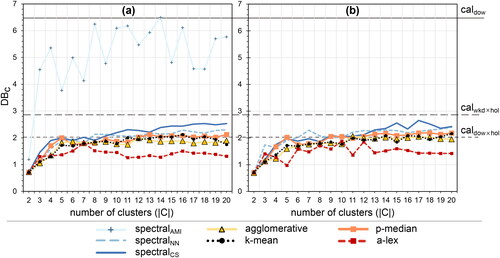
Figure 10. Short-term prediction performance external validation index as a function of the number of clusters across clustering methods. (a) is the original dataset and (b) the reduced dataset
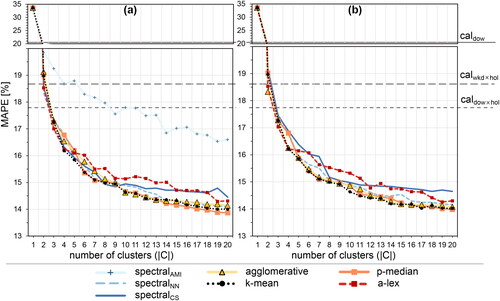
Figure 11. Exponential smoothing short-term prediction performance external validation index of the clustering C as a function of the number of clusters. (a) is the original dataset and (b) the reduced dataset
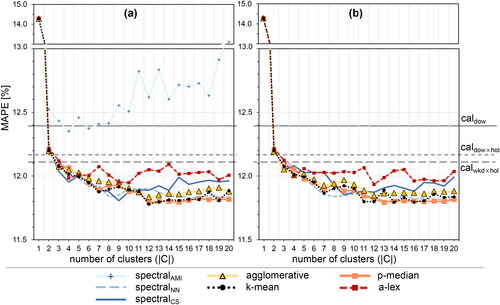
Table 5. Best performing number of clusters according to considered internal indices per clustering method. The clusterings considered as best and reasonable are highlighted in bold.
Table 6. Total computational time for clustering methods to run 19 clusterings with 2-20 clusters.