Figures & data
Table 1. Summary of basic findings from review of recent lighting papers and papers relating to spatial brightness research since 2000.
Table 2. Assumptions of parametric statistical tests.
Fig. 2. Quantile–quantile plots of simulated data with (left) normal and (right) nonnormal distributions.
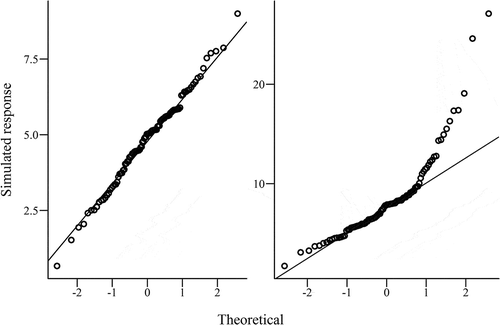
Fig. 4. Probability that the Shapiro-Wilk test will be significant for a sample taken from marginally normal population, by sample size.
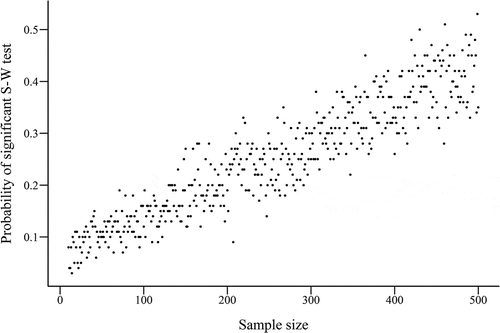
Fig. 5. Example plots of fitted values compared with residuals for linear regression model, showing data with (left) equal variance and (right)unequal variance.
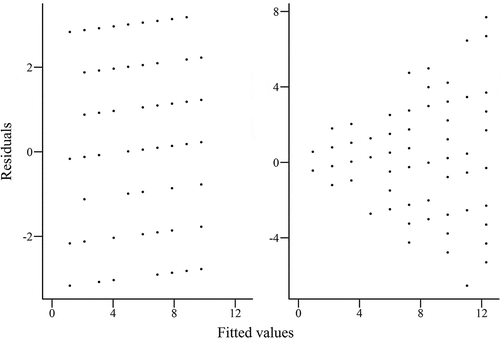
Fig. 6. Density plots showing distributions of two populations of simulated reaction time data, generated using the “pastecs” package in R.
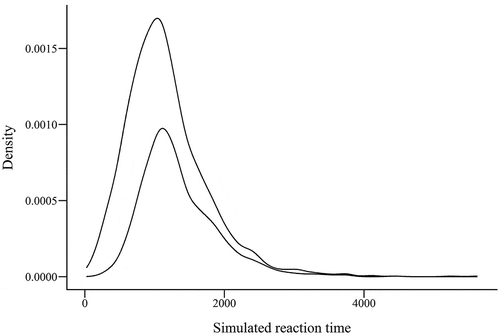
Table 3. Nonparametric alternatives to commonly used parametric tests (see Motulsky Citation1995).
Table 4. Small, medium, and large effect size criteria conventions, for different effect size measures, based on statistical tests used in studies published in Lighting Research & Technology and LEUKOS in 2017.
Fig. 7. Calculated power of one-tailed independent t-test for between-subjects designs (left) and dependent t-test for within-subjects designs (right), by sample size and effect size (Cohen’s d for between subjects, Cohen’s dz for within subjects), assuming an alpha of 0.05. Independent t-test uses group sample size, not total sample size.
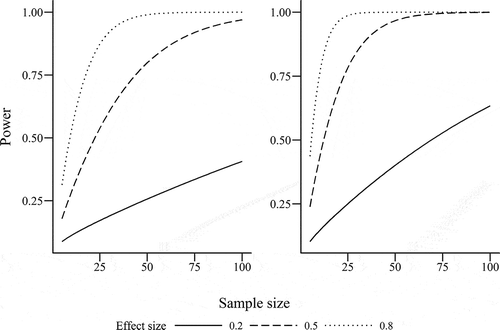
Fig. 8. Estimated power of statistical tests used in reviewed papers, based on sample size and other parameters such as number of measurements (in within-subjects methods), for small (top), medium, (center) and large (bottom) effect sizes. Vertical dashed line indicates conventional minimum recommended power of 0.8 (Cohen Citation1988).
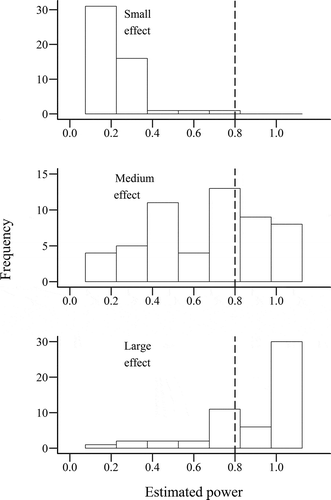