Figures & data
Table 1. List of reviewed research papers, including the explanatory factors and the techniques used to model the degradation of sewer pipes.
Table 2. Main statistics of the numerical predictors.
Table 3. Input variables considered for the development of the model.
Figure 1. a) box plot showing the distribution of pipe age within damage classes. b) count of inspections that fall within each damage class.
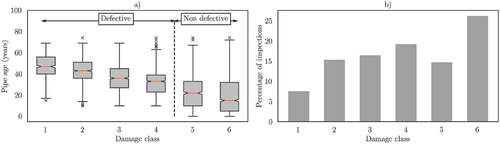
Figure 2. Descriptive statistics of the main variables considered for the development of the model. (VC: vitrified clay, PP: polypropylene, CI: cast iron, PVC: polyvinyl chloride, PRC: polymer concrete, GRP: glass reinforced plastic, PE: polyethylene, PVCU: unplasticised PVC).
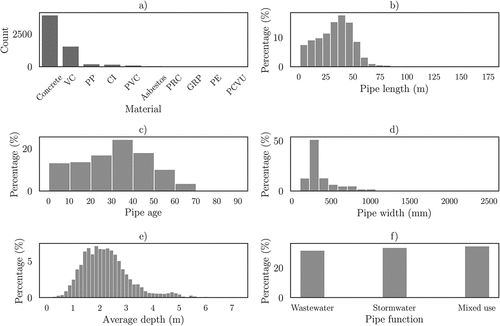
Figure 5. Degradation curves showing the probability of failure of four different sewer pipes according to the trained models.
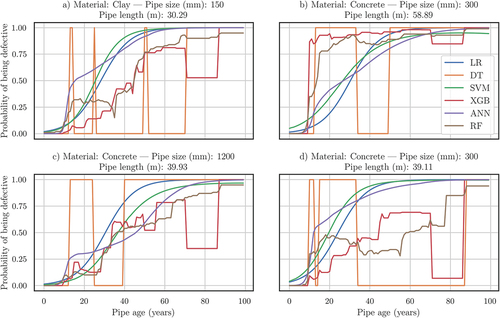
Table 4. Comparison of the average performance metrics (and standard deviation) of the tested models. Random Forest yields the best results for all the metrics.
Table 5. Metrics of the models in terms of the monotonicity of the degradation curves compared across every sample in the dataset.
Table 6. Coefficient estimates, significance and Odds ratios of the variables used in the Logistic Regression model.
Figure 6. The map shows the density of population of the area under study, represented by the approximated count of inhabitants in each pixel. The color of the pipes represent the probability of failure at age 20 of each pipe. Pipes located in the upper left part of figure show a higher probability of failure than the ones located in the opposite side of the area under study. Population density map provided by WorldPop tatem (Citation2017) worldpop.
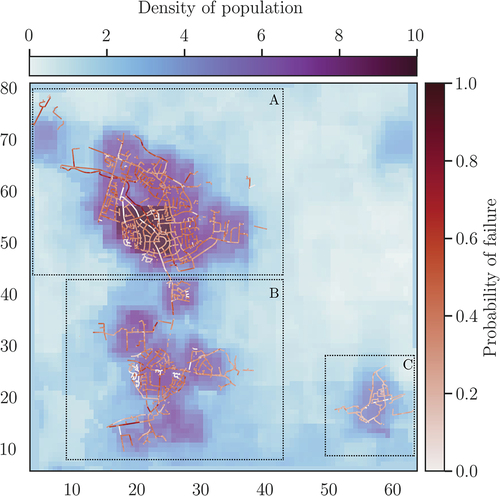
Figure 7. a) comparison of alternative scenarios considering different probability thresholds with the current scenario b) difference of pipe ages at inspection between the current strategy and the results of a model with a probability threshold of 50%.
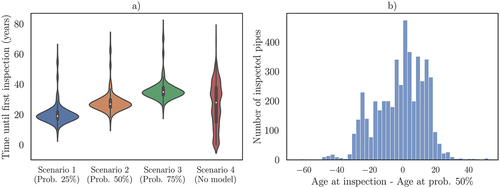
Data availability statement
The data used in this study are available upon request from the corresponding author or can be accessed through the following GitHub repository: https://github.com/Fidaeic/sewer-pred.