Figures & data
Figure 1. (left) visualises an example predictor space with observations of two classes (blue circle and green square). (1) provides two possible separations of the predictor space. (2) chooses the better classification and applies another separation on the subspaces.
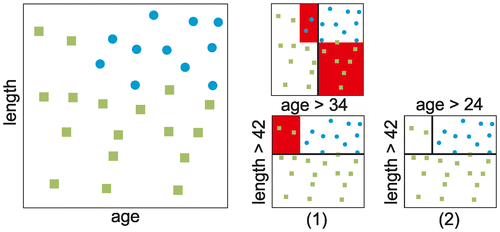
Table 1. Timetable for pipe materials that changed their deterioration patterns due to different manufacturing processes as classified by Roscher (Citation2000).
Table 2. Feature vector x of the data-set with the abbreviations used in the results. The type indicates if the feature is categorical (C) or numerical (N).
Figure 2. Histograms of the pipe network data. Material appendices 1G, 2G and 3G indicate the sub-classification of materials according to Table . The material abbreviations denote asbestos cement (AC), cast iron (CI), ductile iron (DI), glass reinforced plastic (GRP), high impact polypropylene (HIT), polyethylene (PE), polyvinyl chloride (PVC), lead (Pb) and steel (ST). The type abbreviations denote distribution pipe (DP), house connection (HC) and hydrant (HY).
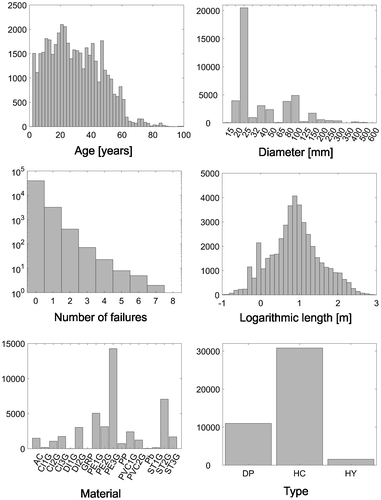
Table 3. Confusion matrices for the evaluated methods showing rates in per cent. RUSBoost is significantly different from the other methods by having a lower true positive rate and a significantly lower false positive rate.
Figure 3. ROC curve for failure pipes, predicted vs. actual response on test set. RUSBoost shows the best performance as it is closes to the ideal classification, a horizontal line with true positive rate 1 for all values of false positive rate. This is also reflected by the highest area under curve (AUC) value of .93.
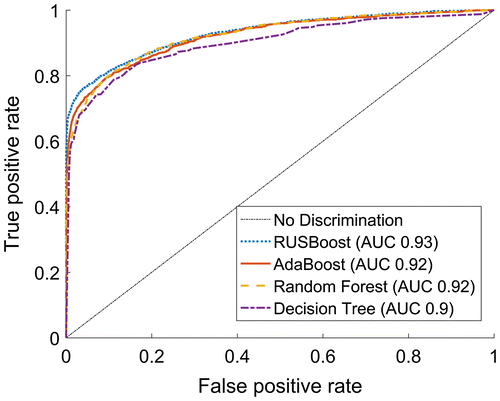
Figure 4. Comparison of predictor importance estimation. The predictors are sorted according to the importance for the first classifier (RUSBoost).
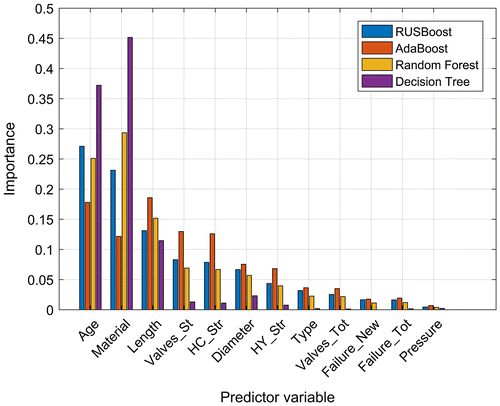
Figure 5. Visualisation of the pipe deterioration prediction for parts of the entire network for the current condition and future in five year steps. The pipe failure probability is visualised by colour intensity. The histograms show the failure probability distribution for the entire network.
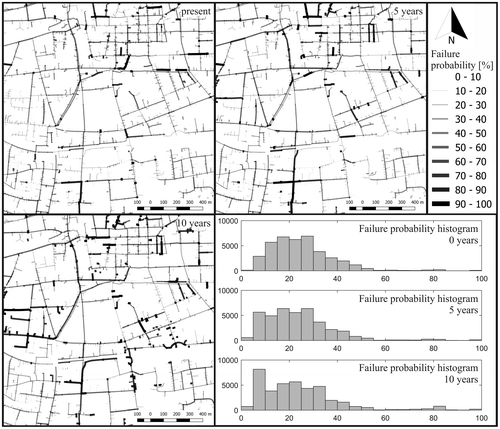