Figures & data
Figure 1. RMSE comparison in terms of free surface height (a) and velocity (b) between various configurations. PO: Perturbed observation. LC: Localized covariance approach. Standard model: no subgrid tensor parameterization. N: number of ensemble member.
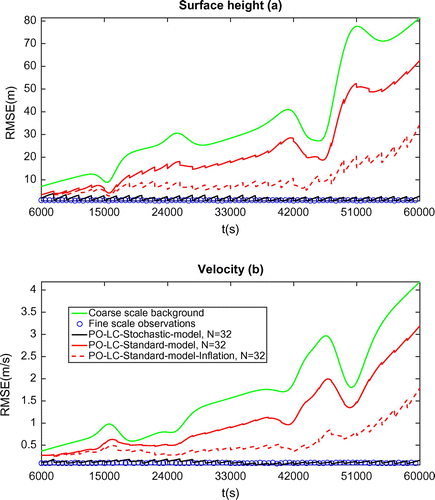
Figure 2. RMSE comparison in terms of free surface height (a) and velocity (b) between various configurations of 4DEnVar. PO: Perturbed observation. LC: Localized covariance approach. Standard model: no subgrid tensor parameterization. N: number of ensemble member. 6 DA cycle phase: s. Forecast phase:
s.
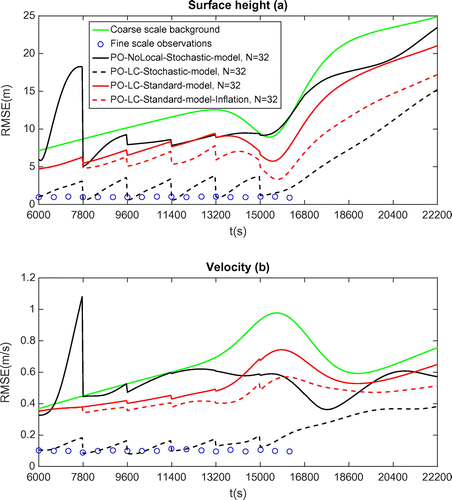
Figure 3. Ensemble spread in terms of free surface height (a) and velocity (b): standard model (circle) with ensemble in function of initial states; standard model (triangle) with ensemble in function of initial states but with multiplicative inflation technique; subgrid model (square) with ensemble in function of initial parameters.
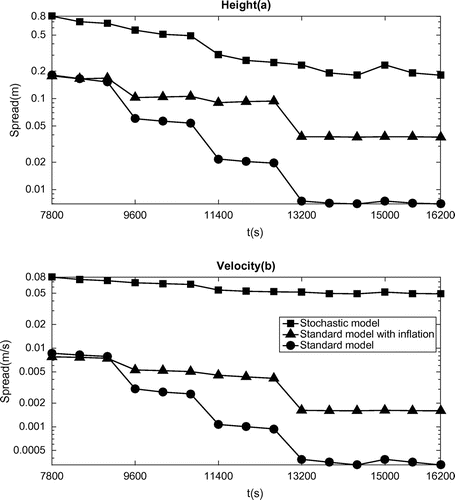
Figure 4. RMSE comparison in terms of free surface height (a) and velocity (b) between various subgrid model configurations in Table of stochastic shallow water model (17). The meaning of different is shown in Table .
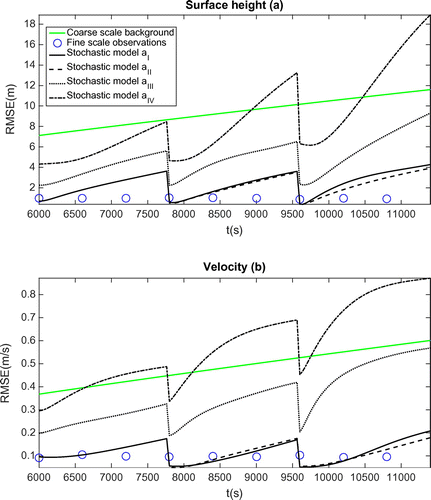
Figure 5. The RMSE of height comparison in the 1st and 2nd cycle as a function of Cut-off distance (LC, localized covariance) or local domain size (LA, local analysis).
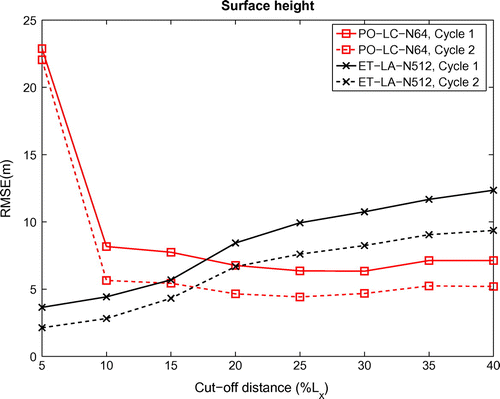
Figure 6. RMSE comparison in terms of free surface height (a) and lengthwise velocity (b) between various configurations of 4DEnVar. ET, ensemble transform. PO, perturbed observation. DE, deterministic ensemble. LC, localized covariance. LA, local analysis. ET-LA-N128-Stochastic (solid line) case with shows ensemble ‘blow up’; ET-LA-N128-Stochastic (dashed line) with
shows ensemble collapse; ET-LA-N512-Stochastic (dotted line) used ensemble relaxation inflation
technique for state ensemble; PO-LC-N64-Standard used standard model and multiplicative inflation technique for state ensemble.
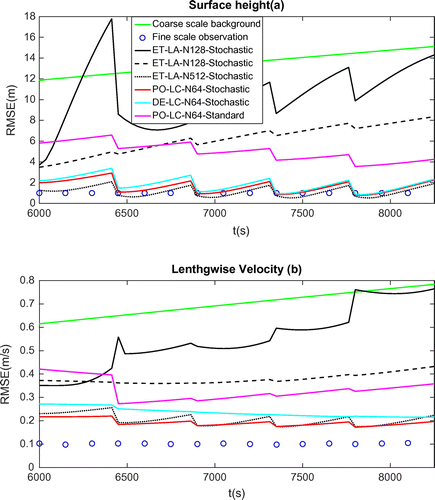
Figure 7. Power spectral density of ensemble anomalies for the forecast ensemble (a), the analysis ensemble by LC (b) and LA (c).
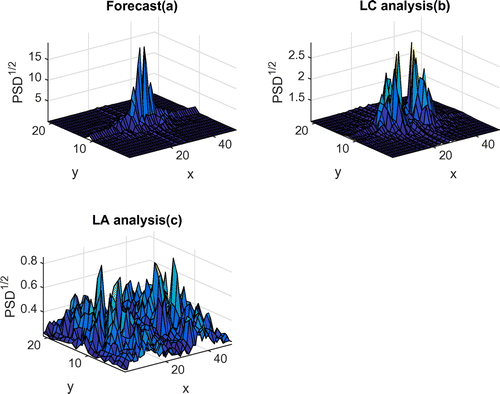
Figure 8. Comparison of various configurations of 4DEnVar w.r.t. (a) Mean surface height of the wave crest region as a function of time and (b) The RMSE against observation at observation time. 4DVar, 4DEnVar-PO-LC and 4DEnVar-ET-LA are tested with the standard model; 4DEnVar-PO-LC-Sto and 4DEnVar-ET-LA-Sto are tested with the stochastic model. LC, localized covariance. PO, perturbed observation. LA, local analysis. ET, ensemble transformation.
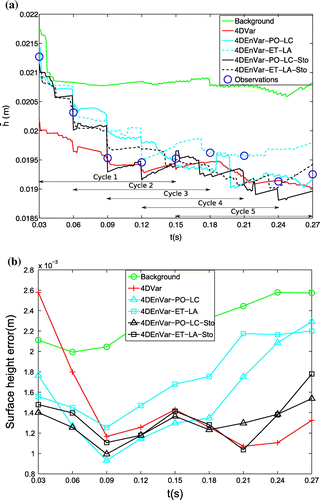
Figure 9. Ensemble Spread in terms of free surface height (a) and lengthwise velocity (b) for: standard model cycle 1 and cycle 5 (circle with solid/dashed line); stochastic model cycle 1 and cycle 5 (square with solid/dashed line).
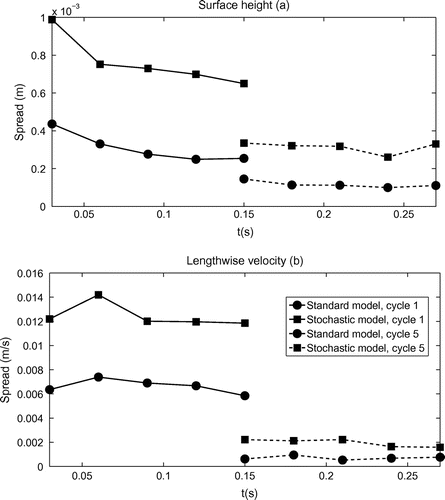
Table 1. Different combination of the terms composing the subgrid model.