Figures & data
Table 1. Effect of β for σ = 1% (true values: k = 1.0, h = 1.0, ρc = 20.0 Bi = 1.0, α = 0.05).
Figure 4. Solution trajectories for Problem 1 using exact solutions (solid line) and a sparse grid (dashed line) for two parameters of level 3 (29 sample points) for interpolation.
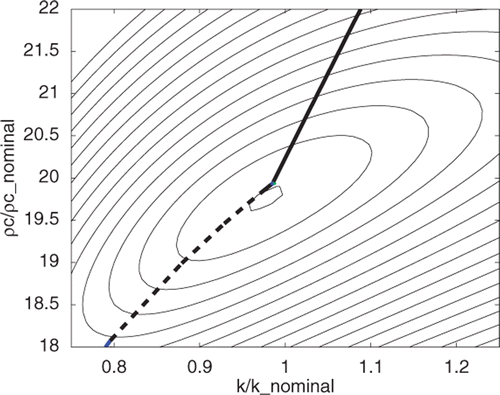
Figure 7. Contours of L(Θ) showing the solution trajectories for Problem 2 using exact solutions of M(Θ) (–-) and interpolation by a sparse grid of level 3 (69 sample points) (- - -).
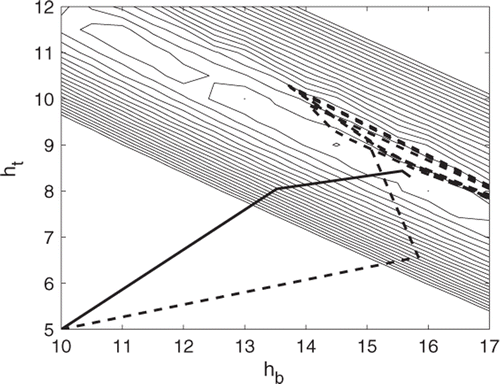
Figure 8. Level 1 and level 2 sparse grid points: (a) level 1 points (O) and (b) level 2 additional points (X).
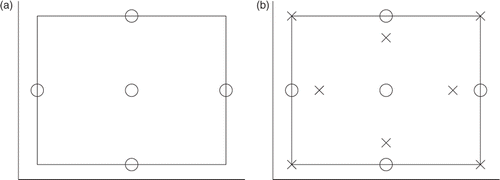
Figure 9. Reduction in maximum errors of T and ∂T/∂k as a function of sparse grid level for Problem 1 (maximum errors evaluated at x/L = [0, 0.25, 0.5, 0.75, 1.0], Fo = [0 : 1 : 41] (‘spinterp’ results evaluated at x/L = 0.5, Fo = 21).
![Figure 9. Reduction in maximum errors of T and ∂T/∂k as a function of sparse grid level for Problem 1 (maximum errors evaluated at x/L = [0, 0.25, 0.5, 0.75, 1.0], Fo = [0 : 1 : 41] (‘spinterp’ results evaluated at x/L = 0.5, Fo = 21).](/cms/asset/b9cb98e6-9644-4b2b-9aea-a04803481b55/gipe_a_675506_f0009.gif)
Figure 10. Interpolated values for sparse grids of increasing levels for Problem 1 using the Chebyshev grid (numbers denote the level): (a) T at x/L = 0.5, Fo = 21 and (b) ∂T/∂k at x/L = 0.5, Fo = 21.
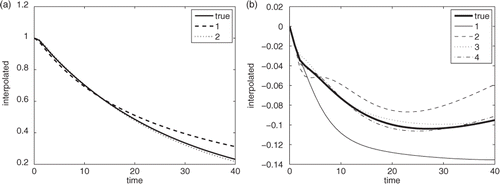
Figure 11. Interpolated values for sparse grids of increasing levels for Problem 1 using the Clenshaw–Curtis grid (numbers denote the level): (a) T at x/L = 0.5, Fo = 21 and (b) ∂T/∂k at x/L = 0.5, Fo = 21.
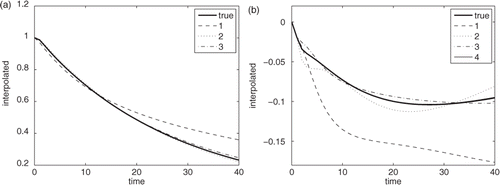
Figure 12. Interpolation error for the temperature at x/L = 0.5, Fo = 21, Problem 1: (a) Clenshaw–Curtis grid and (b) Chebyshev grid. Interpolation error for the ∂T/∂k at x/L = 0.5, Fo = 21, Problem 1: (c) Clenshaw–Curtis grid and (d) Chebyshev grid.
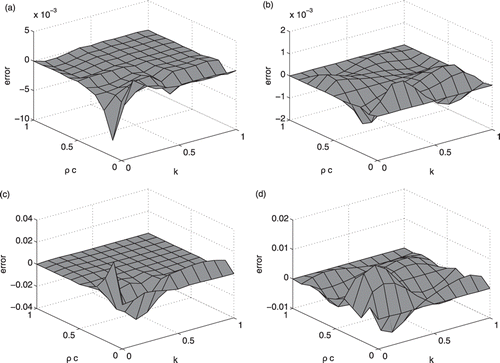
Figure 13. Trajectory for different ways of determining the sensitivities: Exact, solving using the model; SG-a, finite differencing interpolated temperatures; SG-b, differentiating interpolating polynomials representing temperature; SG-c, interpolating sensitivities.
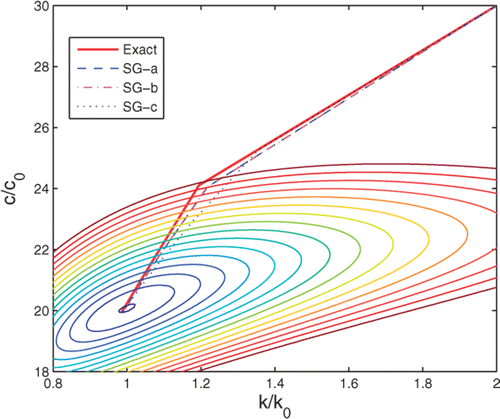
Figure 14. Effect of refining the range of parameters for in Problem 1 (ρc specified): (a) expanded range and (b) centred about LS solution using sparse grid interpolation.
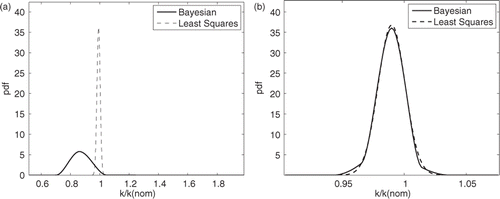