Figures & data
Figure 1. Plot of the PSNR values of the restored image using the TGV and TV+TV approach with respect to the parameters
and
. We can see that the highest achieved PSNR for the TGV
approach is related to the values
and
. For the TV+TV
model, the best PSNR corresponds to the values
and
. Note that the original image is the Pirate one corrupted by Gaussian noise of variance 0.3.
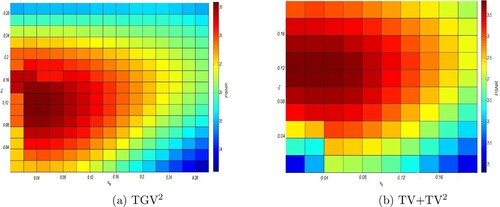
Figure 2. The obtained denoised image compared to other classical approaches for the (Pirate image), where the noise is considered to be Gaussian of : (a) Noisy image, (b) ROF model [Citation3], (c) NLM model [Citation51], (d) TV+TV
[Citation19] (e) TGV model [Citation36] and (f) Our model.
![Figure 2. The obtained denoised image compared to other classical approaches for the (Pirate image), where the noise is considered to be Gaussian of σ=0.3: (a) Noisy image, (b) ROF model [Citation3], (c) NLM model [Citation51], (d) TV+TV2 [Citation19] (e) TGV model [Citation36] and (f) Our model.](/cms/asset/0fbed0dd-f9f8-488a-bf96-1125ea42ea3e/gipe_a_1867547_f0002_oc.jpg)
Figure 3. The obtained denoised image compared to other classical approaches for the (Cameraman image), where the noise is considered to be Gaussian of : (a) Noisy image, (b) ROF model [Citation3], (c) NLM model [Citation51], (d) TV+TV
[Citation19], (e) TGV model [Citation36] and (f) our model.
![Figure 3. The obtained denoised image compared to other classical approaches for the (Cameraman image), where the noise is considered to be Gaussian of σ=0.5: (a) Noisy image, (b) ROF model [Citation3], (c) NLM model [Citation51], (d) TV+TV2 [Citation19], (e) TGV model [Citation36] and (f) our model.](/cms/asset/723fe932-8946-4e77-8eaf-d2f402adec63/gipe_a_1867547_f0003_oc.jpg)
Figure 4. The computation of the parameter λ with respect to the iteration for the two images Pirate and Cameraman: (a) The Pirate image and (b) The Cameraman image.
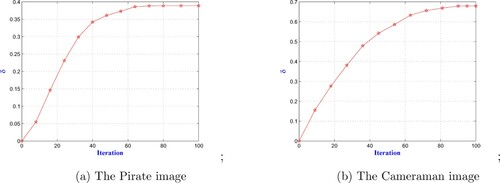
Figure 5. The obtained denoised image compared to other classical approaches for the Cameraman image, where the noise is considered to be impulse one of parameter 0.3: (a) Noisy image, (b) ROF [Citation3], (c) NLM [Citation51], (d) L-TV+TV
[Citation19], (e) L
-TGV [Citation36] and (f) Our model.
![Figure 5. The obtained denoised image compared to other classical approaches for the Cameraman image, where the noise is considered to be impulse one of parameter 0.3: (a) Noisy image, (b) ROF [Citation3], (c) NLM [Citation51], (d) L1-TV+TV2 [Citation19], (e) L1-TGV [Citation36] and (f) Our model.](/cms/asset/c90d365a-eedf-4a5d-9cc6-dbaaa0706d16/gipe_a_1867547_f0005_oc.jpg)
Figure 6. The obtained denoised image compared to other classical approaches for the (Penguin image), where the noise is considered to be impulse one of parameter 0.5: (a) Noisy image, (b) ROF model [Citation3], (c) NLM model [Citation51], (d) L-TV+TV
[Citation19], (e) L
-TGV model [Citation36] and (f) Our model.
![Figure 6. The obtained denoised image compared to other classical approaches for the (Penguin image), where the noise is considered to be impulse one of parameter 0.5: (a) Noisy image, (b) ROF model [Citation3], (c) NLM model [Citation51], (d) L1-TV+TV2 [Citation19], (e) L1-TGV model [Citation36] and (f) Our model.](/cms/asset/43fd1e32-80cb-49e3-a903-a3f4e88281c3/gipe_a_1867547_f0006_oc.jpg)
Figure 7. The computation of the parameter λ with respect to the iteration for the two images Camerman and Penquin: (a) The Pirate image and (b) The Penguin image.
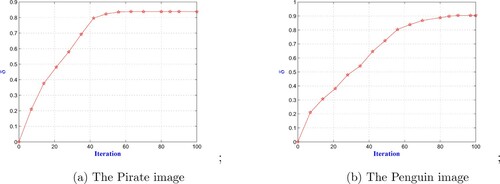
Table 1. The PSNR table.
Table 2. The SSIM table.
Table 3. The normalized distance table.
Table 4. The normalized distance table.
Figure 8. The restored image and the parameter λ using the two admissible sets of the Lena image: (a) Noisy, (b) X using , (c) λ using
, (d) X using
and (e) λ using
.
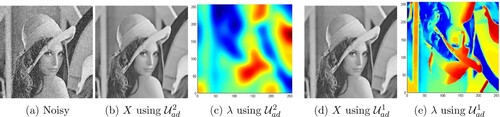
Figure 9. The restored image X and the corresponding weighted parameter λ using the admissible set . The Baboon image is contaminated by Gaussian noise with
, Penguin image is contaminated by
, Zebra image is contaminated by
, Tiger image is contaminated by
. The used parameters for these tests are:
,
and
: (a) Noisy, (b) Obtained X, (c) λ and (d) Image of λ.
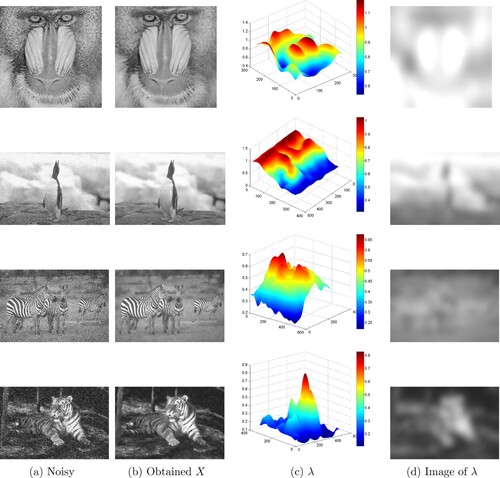
Figure 10. The restored image X and the corresponding weighted parameter λ using the admissible set . the Baboon image is contaminated by Gaussian noise with
, Penguin image is contaminated by
, Zebra image is contaminated by
, Tiger image is contaminated by
. The used parameters for these tests are:
,
and
: (a) Noisy, (b) Obtained X, (c) Obtained λ and (d) Image of λ.
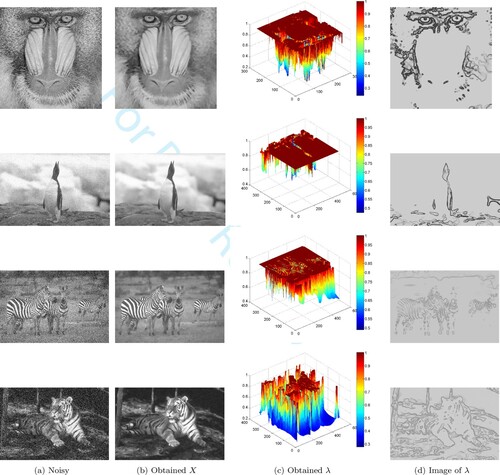
Figure 11. Comparison between the obtained clean image X and the SDRTV method with the respective computation of the spatially dependent parameter λ of the Head image: (a) Original, (b) Noisy, (c) λ initial, (d) SDRTV, (e) Obtained λ, (f) Image of λ, (g) Proposed PDE, (h) Obtained λ and (i) Image of λ.
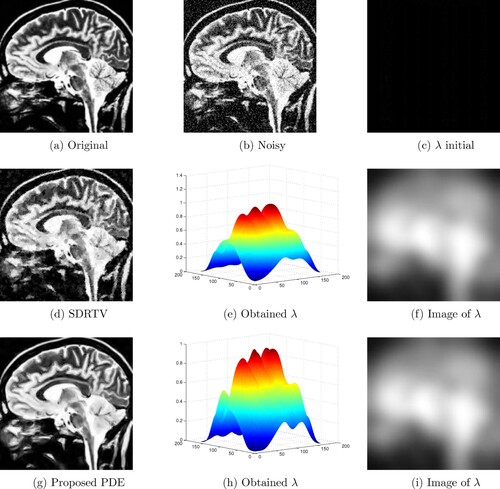
Figure 12. Comparison between the obtained clean image and the SDRTV method and the respective computation of the spatially dependent parameter λ of the Brain image: (a) Original, (b) Noisy, (c) λ initial, (d) SDRTV, (e) Obtained λ, (f) Image of λ, (g) SDRTV, (h) Obtained λ and (i) Image of λ.
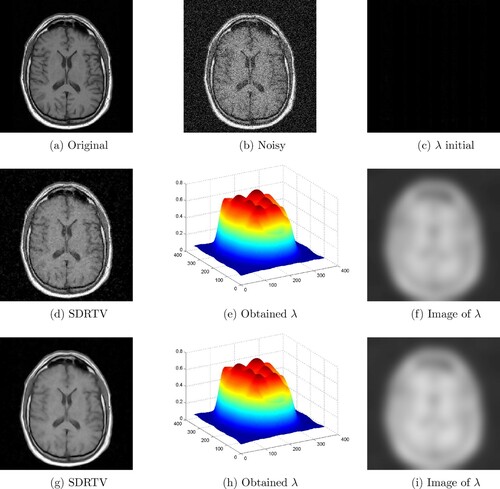
Figure 13. Comparison between the obtained clean image X and the SDRTV method with the respective computation of the spatially dependent parameter λ of the Dolphins image: (a) Original, (b) Noisy, (c) λ initial, (d) SDRTV, (e) Obtained λ, (f) Image of λ, (g) Obtained X, (h) Obtained λ and (i) Image of λ.
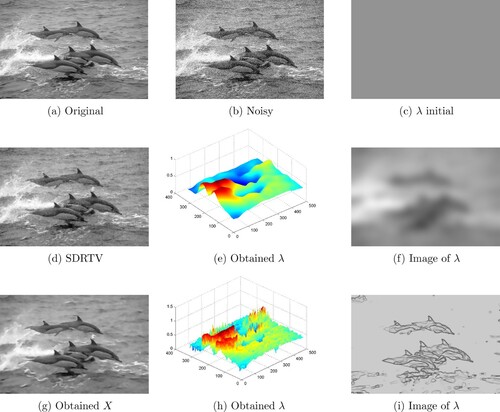
Figure 14. Comparison between the obtained clean image X and the SDRTV method with the respective computation of the spatially-dependent parameter λ of the Plane image: (a) Original, (b) Noisy, (c) λ initial, (d) SDRTV, (e) Obtained λ, (f) Image of λ, (g) Obtained X, (h) Obtained λ and (i) Image of λ.
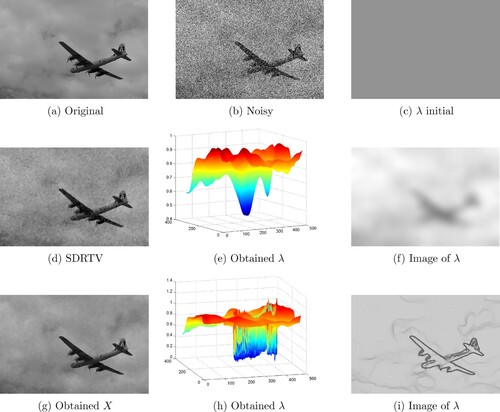
Figure 15. Comparison between the obtained clean image X and the respective computation of the spatially dependent parameter λ for the scalar case and for it two set of admissible values and
for the Fishes image: (a) Original, (b) Noisy, (c) scalar λ, (d) weighted
and (e) weighted
.
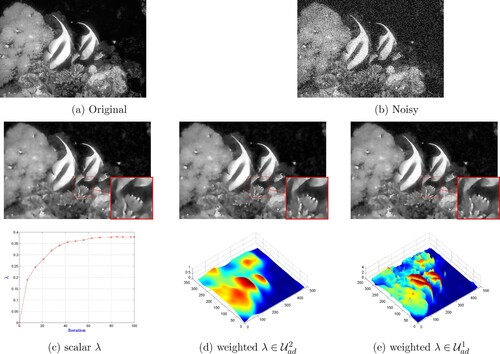
Figure 16. The obtained denoised image compared to other PDE approaches with respect to both quality measures PSNR and SSIM. First row: noisy images. Second row: FOPDE. Third row: AEFD. Fourth row: AFOD. Fifth row: Our approach. (a) PSNR = 19.17, SSIM = 0.327. (b) PSNR = 15.40, SSIM = 0.377. (c) PSNR = 15.12, SSIM = 0.310. (d) PSNR = 14.08, SSIM = 0.286. (e) PSNR = 23.79, SSIM = 0.539. (f) PSNR = 22.61, SSIM = 0.588. (g) PSNR = 20.66, SSIM = 0.548. (h) PSNR = 20.41, SSIM = 0.422, (i) PSNR = 26.19, SSIM = 0.719, (j) PSNR = 23.95, SSIM = 0.650, (k) PSNR = 23.62, SSIM = 0.647. (l) PSNR = 28.48, SSIM = 0.747. (m) PSNR = 26.94, SSIM = 0.746, (n) PSNR = 25.62, SSIM = 0.741, (o) PSNR = 22.81, SSIM = 0.627, (p) PSNR = 24.96, SSIM = 0.597, (q) PSNR = 28.14, SSIM = 0.826, (r) PSNR = 27.65, SSIM = 0.821, (s) PSNR = 26.03, SSIM = 0.765 and (t) PSNR = 31.91, SSIM = 0.872.
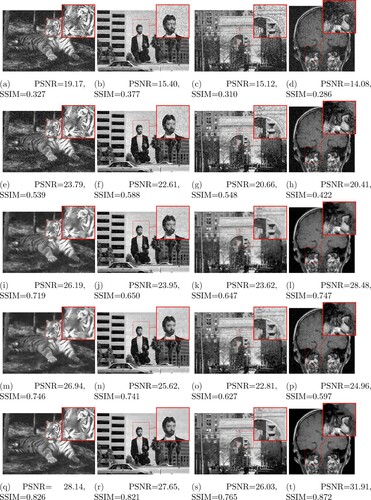