Figures & data
Table 1. Prior work concerning acoustic defect detection in metal AM.
Figure 1. Schematic representation of a multi-beads printing (left) and Actual printing (right) as seen from the build direction. Geometrically defective segments lead to voids between two successive beads will affect the final printed part strength and quality.
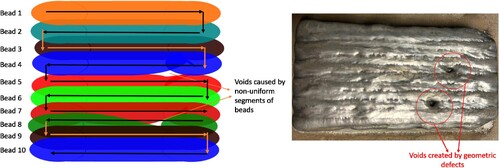
Figure 3. Experimental setup of SUTD Robotic WAAM for Bead Geometry and Acoustic Data Collection (left). (a) Original beads with plate (b) Mesh representation of beads with plate (right).
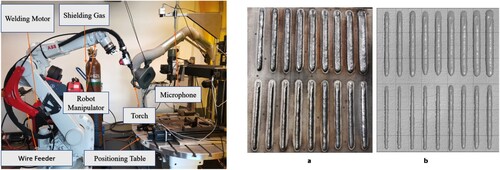
Figure 5. Range of mean curvature of geometrically good (left) and bad (right) bead segment. The red circle indicates a geometric defect.
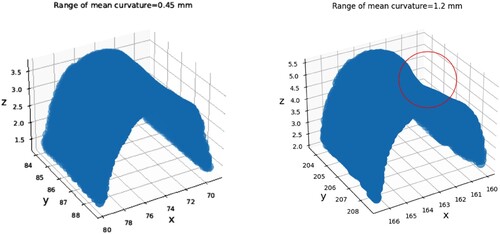
Figure 6. Bead with defective and non-defective segments (Top) with corresponding acoustic time-domain waveforms (Middle) and range curvature (Bottom).
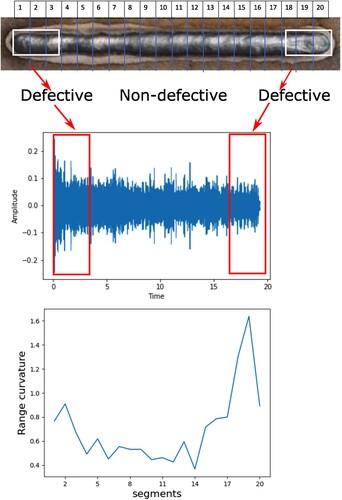
Figure 9. Distribution plots for good and bad segments of beads for different thresholds (First three plots). Different thresholds vs overlapping area plot(last plot).
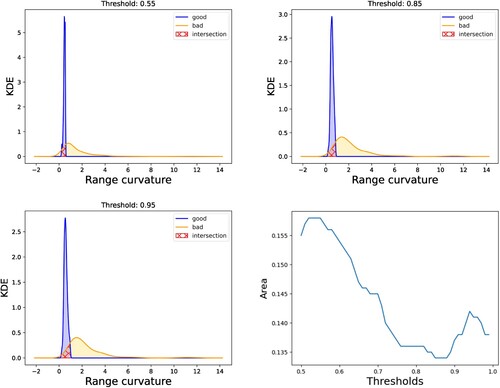
Figure 10. KNN classification error based on the number of nearest neighbour K and weight function for PCA features (top plot) and MFCC features (bottom plot).
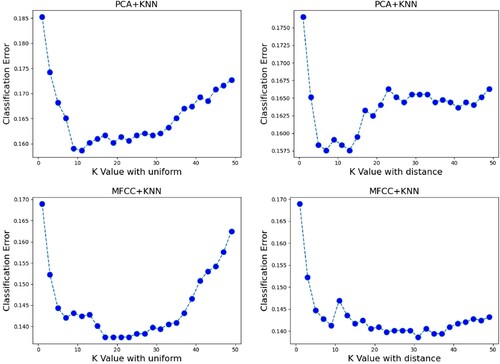
Figure 11. SVM classification error based on the choice of γ and C for the RBF and Sigmoid kernels with PCA features (top) and MFCC features (bottom).
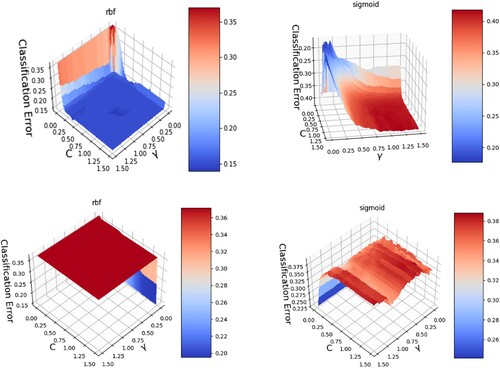
Figure 12. RF classification error based on the number of trees for PCA features (left plot) and MFCC features (right plot).
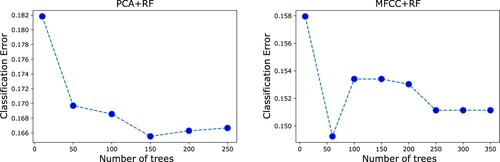
Figure 13. NN Classification accuracy based on the number of layers of a NN architecture for PCA features (left plot) and MFCC features (right plot).
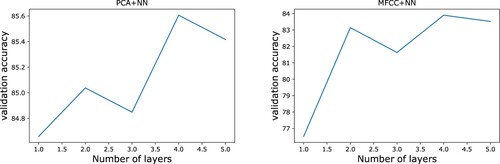
Table 2. Different Combination of number of neurons for PCA+NN.
Table 3. Different Combination of number of neurons for MFCC+NN.
Figure 14. CNN classification accuracy based on the number of filters within its architecture for PCA features (left plot) and MFCC features (right plot).
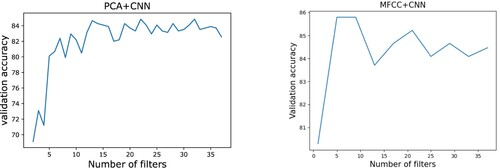
Figure 15. Average validation accuracy for the different defect identification models vs the number of principal components used as a feature.
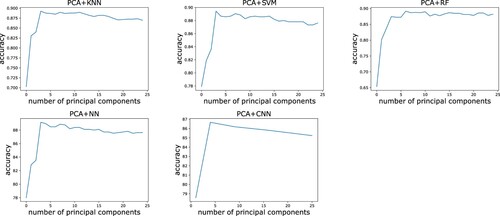
Figure 16. The loss curve for PCA+NN and MFCC+NN (top) The loss curve for PCA+CNN and MFCC+CNN (bottom).
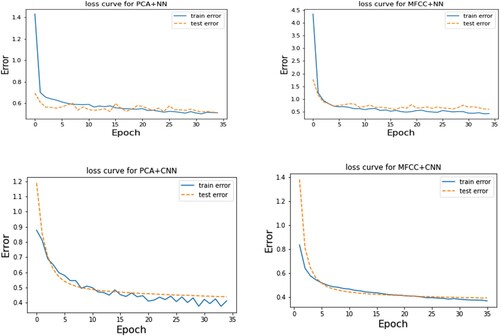
Table 4. Comparative study of all of the proposed defect identification model based on F1 score, precision, and recall.
Table 5. Comparative study of all of the proposed defect identification model based on Confusion Matrix.