Figures & data
Table 1. Drug–target interaction resources for target activity predictions
Table 2. Cell-based pharmacogenomic resources for drug efficacy predictions
Table 3. Pathway resources for understanding compounds’ mode of action
Table 4. Chemical structure databases using InchiKey searches or structure drawings
Figure 1. Schematic illustration of overlaps between cancer-related gene sets. There are both target-based and non-target-based features that can be predictive of specific drug efficacies in various cancer types. The cancer genes and protein targets should be studies separately for each tissue type (e.g. breast cancer) and inhibitor class (e.g. HER2 inhibitors). Selective efficacies are preferred in the repurposing predictions, as tissue of origin-independent targets may lead to toxic side effects
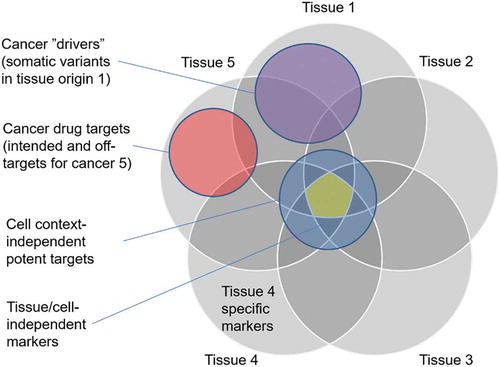