Figures & data
Figure 1. Artificial intelligence for precision medicine. (a) Categorization of AI, ML, and DL. (b) Performance of ML and DL as a function of sample size. (c) The relation between the model complexity, interpretability and translatability. (d) Gartner hype cycle for AI in precision medicine.
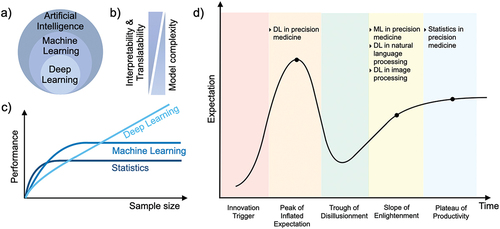
Figure 2. Artificial intelligence in pharmacogenomics and precision medicine. (a) Statistical methods are the foundation of precision medicine, e.g. (b) leveraging ANOVA models to investigate genetic biomarkers in melanoma cell lines treated with the BRAF inhibitor PLX-4720. (c) BRAF mutant cancer cell lines are sensitive to PLX-4270 treatment. (d) ML models enable predictive modeling in pharmacogenomics. (e) Clustering of melanoma cell lines according to their mutational status visualized in the UMAP space. (f) Prediction of IC50 values of cell lines treated with a BRAF inhibitor using a linear regression model. (g) Classification of drug response using a random forest model. (h) DL architectures empower modeling of complex associations in drug discovery. For instance, (i) an autoencoder architecture for creating a two dimensional manifold of melanoma cell lines for consecutive downstream analysis, and (j) a feed-forward neural network architecture for drug response classification.
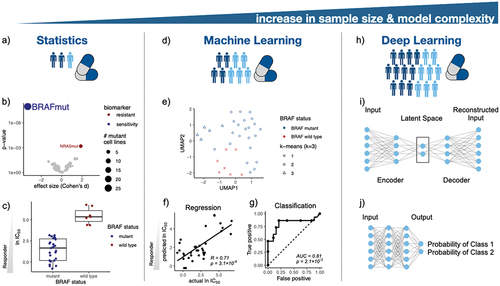