Figures & data
Figure 1. GSA-based approaches proposed in this article (black arrows) for articulating different research activities contributing to the generation of context-specific evidence and knowledge generalisation, and thereby addressing current knowledge gaps and limitations in LSS research.

Table 1. Purposes, methods and current application of geographic similarity analysis in Land System Science research.
Figure 2. Overview of combinations of GSA methods with other established LSS research methods proposed for addressing current knowledge gaps and limitations in LSS.
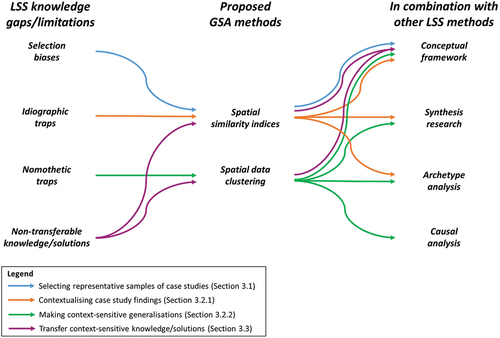
Figure 3. a. SIPATH conceptual framework (adapted from Helfenstein et al., Citation2020). b. Locations in Europe considered as potential case study sites for the SIPATH project, based on existing collaboration networks.
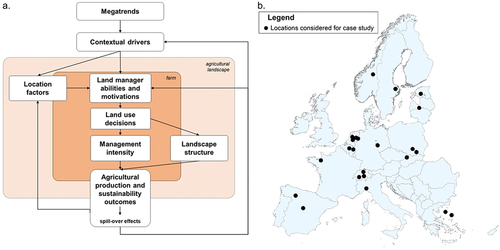
Figure 4. Representativeness analysis on the four social-ecological dimensions considered in the SIPATH project for the case study site in Lielvircava, Latvia. The similarity indices range from 0 to 1, with 1 indicating that a location is fully identical to the case study site in terms of considered social-ecological variables. The representativeness categories were defined as follows: Very low representativeness: 0–0.25; Low representativeness: 0.25–0.50; Moderate representativeness: 0.50–0.75; High representativeness: 0.75–1.
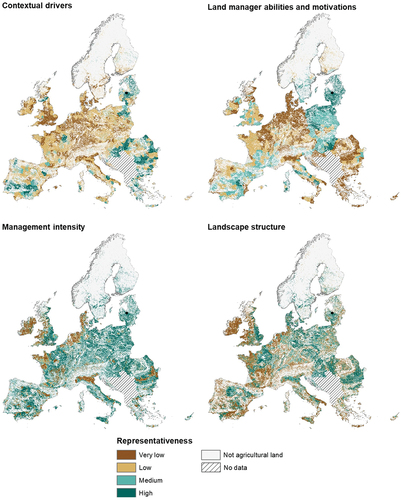
Figure 5. Number of considered social-ecological dimensions for which the selection of SIPATH case studies is highly representative in Europe, and the share of each category in terms of the total agricultural land area in Europe.
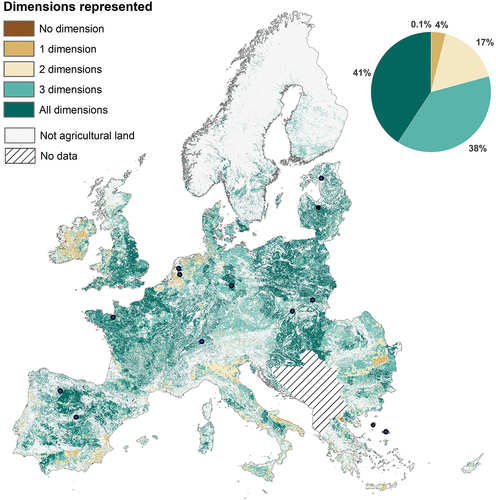
Figure 6. Archetypical development pathways at the farm and landscape levels identified in the SIPATH case studies (adapted from Helfenstein et al., 2023), and respective large-scale archetypical land system change trajectories (adapted from Levers et al., Citation2018).
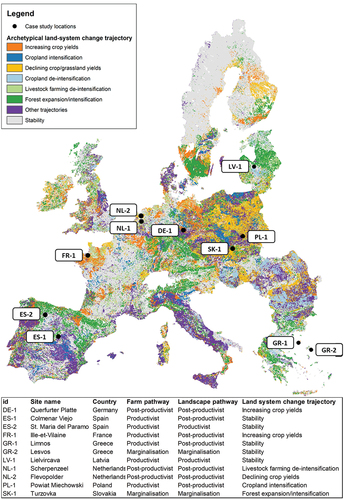
Figure 7. Modes for context-sensitive knowledge transfer in LSS research (adapted from Bennett et al., Citation2021). The colours of the circles represent (distant) locations with high degree of similarity in terms of social-ecological features, the size of the circles the magnitude impact of the knowledge transfer (e.g. in terms of geographical area or option space), and the different arrows the knowledge element that is transferred between locations. Learning: transferring solutions from locations that are contextually similar to the case studies where the solutions were generated. Aggregation: quantifying the cumulative effect of upscaling a solution, assuming that the solution is adopted across regions with a high degree of similarity to the original case study where the solution was generated. Contagion: transferring the procedures used to generate solutions in participatory processes across locations that are contextually similar; new solutions and procedures may iteratively emerge every time such participatory processes take place. Compensation: identifying similar locations where trade-offs from interventions in one location could be potentially offset by interventions in the other location(s).
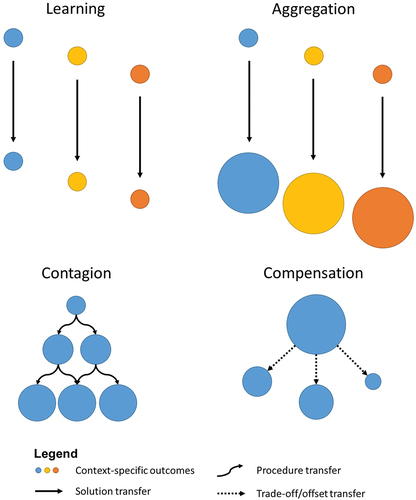
Figure 8. Toolbox of GSA-based approaches for addressing current knowledge gaps and limitations in LSS research.
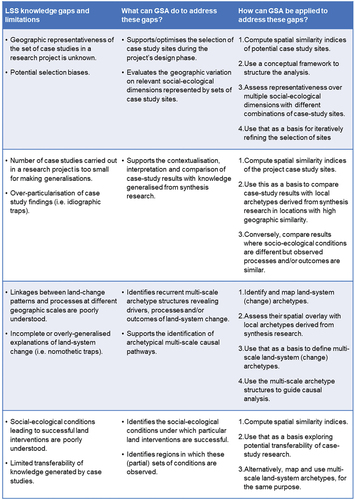