Abstract
Existing interfaces between mathematics and art, and geography and art, began overlapping in recent years. This newer overarching intersection partly is attributable to the scientific visualization of the concept of an eigenvector from the subdiscipline of matrix algebra. Spectral geometry and signal processing expanded this overlap. Today, novel applications of the statistical Moran eigenvector spatial filtering (MESF) methodology to paintings accentuates and exploits spatial autocorrelation as a fundamental element of art, further expanding this overlap. This paper studies MESF visualizations by compositing identified relevant spatial autocorrelation components, examining a particular Van Gogh painting for the first time, and more intensely re-examining several paintings already evaluated with MESF techniques. Findings include: painting replications solely based upon their spatial autocorrelation components as captured and visualized by certain eigenvectors are visibly indistinguishable from their original counterparts; and, spatial autocorrelation supplies measurements allowing a differentiation of paintings, a potentially valuable discovery for art history.
GRAPHICAL ABSTRACT
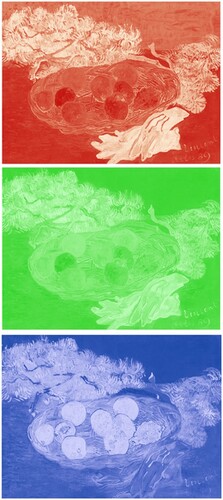
Acknowledgements
Daniel A. Griffith is an Ashbel Smith Professor of Geospatial Information Sciences.
Disclosure statement
No potential conflict of interest was reported by the author(s).
Notes
1 The algebraic multiplicity of eigenvalues for the type of n-by-n symmetric matrix C discussed in this paper is either 1 or 2. Those with multiplicity 1 are distinct and hence linearly independent, whereas those with multiplicity 2 have extensions of the form of these preceding eigenvectors that also are linearly independent (see Griffith, Citation2000). In other words, the construction of n linearly independent vectors is possible for matrix C, and hence it can be diagonalized.
2 Double centering simultaneously removes the principal eigenvector of matrix C and inserts an eigenvector proportional to the vector 1 with an accompanying eigenvalue of 0. Consequently, the largest eigenvalue decreases from 2{cos[π/(P + 1)] + cos[π/(P + 1)]} to 2{cos[2π/(P + 1)] + cos[π/(P + 1)]} or 2{cos[π/(P + 1)] + cos[2π/(P + 1)]}.
3 Powerful computer algorithms, such as WAIVS (Workflows for Analysis of Images and Visual Stylometry; http://www.waivs.org/info.phtml), are emerging that seek to achieve these outcomes. One fruitful research avenue would be to incorporate spatial autocorrelation analysis into these tools.