Figures & data
Table 1. Penalized vs. ordinary LSEs for L1.
Figure 1. Bootstrap estimate of the LSE covariance for the L1 system. True asymptotic LSE covariance matrix vs. diffusion bootstrap covariance matrices for L1 systems with varying n (via Algorithm 1). To facilitate comparisons, the penalty was removed, i.e. for all cases considered.
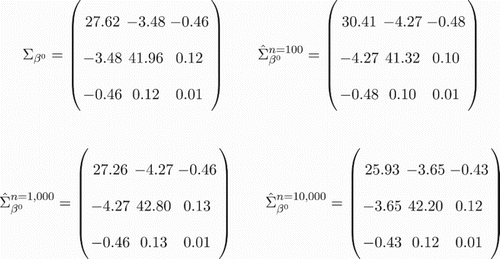
Figure 2. LSE covariance recovery via bootstrap for the L1 system. The contour plots for the true asymptotic LSE bivariate marginal distributions (top panel) and their LNA bootstrap estimates (bottom panel) for the LSE in the L1 system with . The recovery of the multivariate Gaussian distribution covariance structure by the bootstrap procedure is clearly visible.
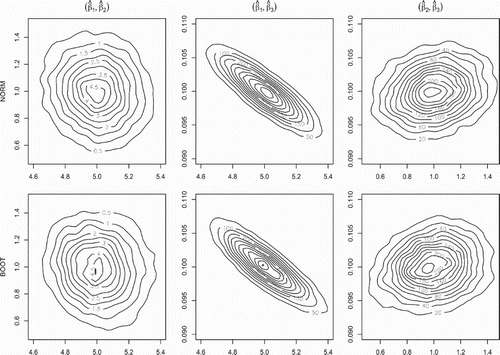
Table 2. Time-course RT-PCR experiment results for L1 gene.
Figure 3. Bootstrap likelihood profiling. The LNA bootstrap () plots approximating for several different n values the residuals distributions for the model (Equation18
(18)
(18) ) fitted to data in Table and comparing them with the actual observed residuals (grey vertical lines). As seen from the plots, around the value
the mode of the residuals distribution best aligns with the observed value. Note that the alignment is very poor for
, which is the estimated volume used in [Citation16].
![Figure 3. Bootstrap likelihood profiling. The LNA bootstrap (M=5000) plots approximating for several different n values the residuals distributions for the model (Equation18(18) c1β(t)=β1β3[1+(1+tβ2)e−β2t].(18) ) fitted to data in Table 2 and comparing them with the actual observed residuals (grey vertical lines). As seen from the plots, around the value n=5000 the mode of the residuals distribution best aligns with the observed value. Note that the alignment is very poor for n=1000, which is the estimated volume used in [Citation16].](/cms/asset/8a4744af-309b-4f9c-acdc-b3d3dd0e33a8/tjbd_a_1033022_f0003_b.gif)
Figure 4. Confidence envelopes for different n values. The ODE model data (Equation18(18)
(18) ) fitted to the trajectory data in Table plotted along with the fits 95% confidence envelopes for two different estimated system sizes. The left panel shows the bounds obtained in [Citation16] for the system size
whereas the right panel shows the bounds obtained by taking
.
![Figure 4. Confidence envelopes for different n values. The ODE model data (Equation18(18) c1β(t)=β1β3[1+(1+tβ2)e−β2t].(18) ) fitted to the trajectory data in Table 2 plotted along with the fits 95% confidence envelopes for two different estimated system sizes. The left panel shows the bounds obtained in [Citation16] for the system size n=1000 whereas the right panel shows the bounds obtained by taking n=5000.](/cms/asset/be70fc5b-d6c7-475a-8b72-ed6051e127e4/tjbd_a_1033022_f0004_c.jpg)