Figures & data
Figure 1. The data sets of interest from [Citation9,Citation25]. The total polymerized mass is measured by Thioflavin T (ThT), which is one of the most common experimental tools for in vitro protein polymerization [Citation25,Citation29]. (available in colour online)
![Figure 1. The data sets of interest from [Citation9,Citation25]. The total polymerized mass is measured by Thioflavin T (ThT), which is one of the most common experimental tools for in vitro protein polymerization [Citation25,Citation29]. (available in colour online)](/cms/asset/9e065c6a-b2d5-4983-ac1f-8a0ef4d0931f/tjbd_a_1050465_f0001_c.jpg)
Figure 3. Plots with simulated data: (a) correct cost function vs. time ; (b) incorrect cost function vs. time
.
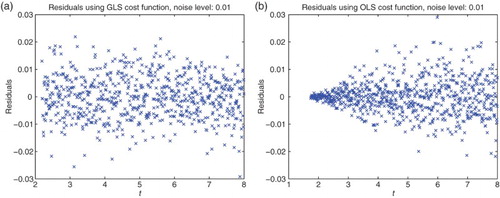
Figure 4. Plots with simulated data: (a) correct cost function vs. model ; (b) incorrect cost function vs. model
.
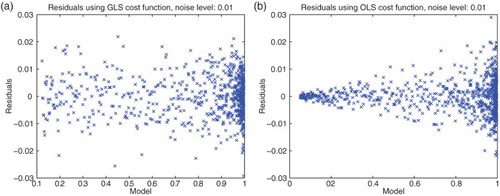
Table
Table
Table
Table
Table
Table
Table
Table
Table
Table
Figure 20. Three parameters estimation (,
and
): bootstrapping distribution for
. We used GLS and
runs.
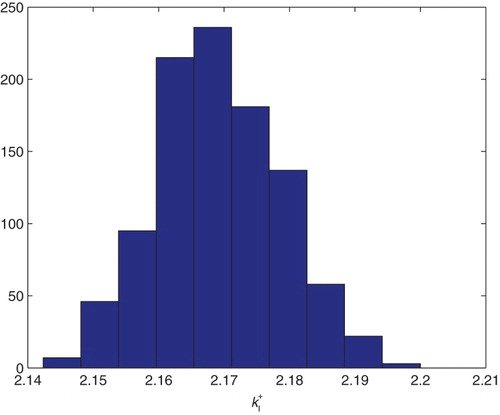
Figure 21. Three parameters estimation (,
and
): bootstrapping distribution for
. We used GLS and
runs.
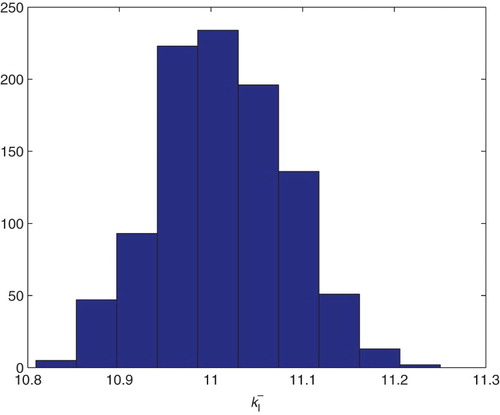
Figure 22. Three parameters estimation (,
and
): bootstrapping distribution for
. We used GLS and
runs.
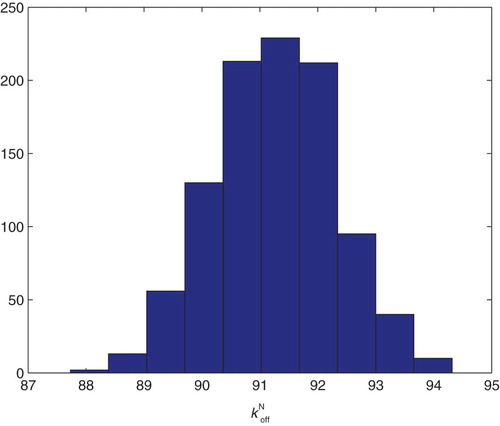
Table
Table
Table