Figures & data
Figure 1. Layout of four-neighbor combinations, with all yielding the same neighbor average. The spatial heteroskedasticity question is not resolved by the conventional Moran scatterplot.

Figure 2. The contiguity plot of the covariogram, variogram, and their close relationship: γ(h)=sill–cov(h). Anisotropic phenomena can also be modeled by using different distances for the major and minor range in a perpendicular layout. As expected, closer samples hold higher weights than distant ones.
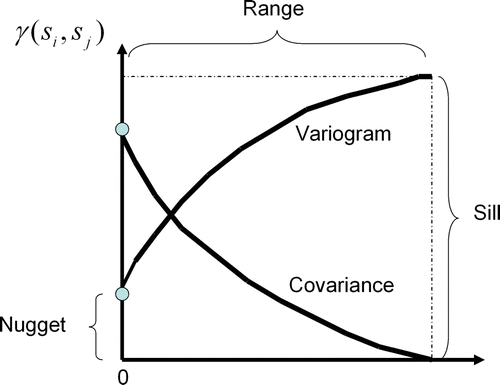
Figure 3. Overall picture of the Moran scatterplot showing the uncertainty regions, high–high, medium–medium, and low–low patterns, positive outliers (1), (3), (4), and (6), negative ones (5) and (2), and the Moran's I impact on the Moran scatterplot.
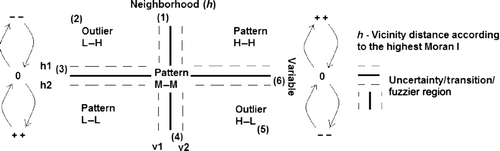
Figure 5. Theoretical four-sample layout for analysis of the weight impact on the Moran variance scatterplot.
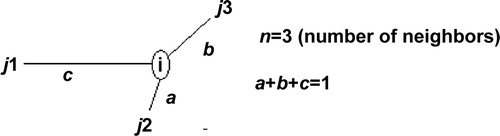
Table 1. The Moran variance scatterplot for the five theoretical situations of .
Figure 8. Contour map for the Nebraska soil organic matter dataset. Black triangles represent sampling points.
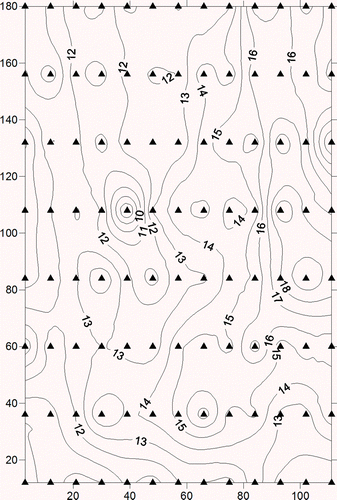
Figure 9. The Pb dataset mapping by the Moran location scatterplot. Filled squares represent location and type of spatial autocorrelation classification (depending on the corresponding quadrant) for each sample.
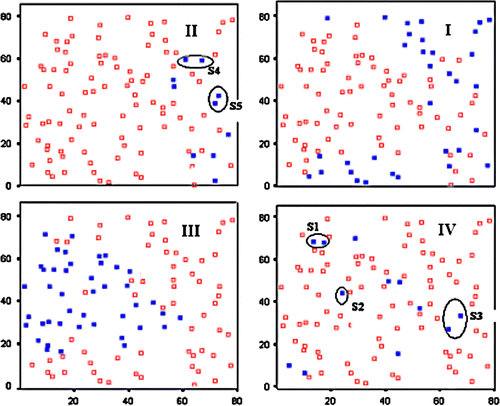
Figure 10. Effect of range misspecification in Moran's I calculation. Filled squares represent samples that lie within the uncertainty regions for the optimal range (I=+0.37, left) and a non-optimal range (I=+0.08, right).
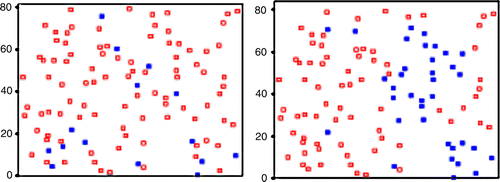
Figure 11. The Moran location scatterplot view of the four quadrants of the San Diego dataset (part II). There is a clear improvement in this spatial autocorrelation measure when compared with the traditional one (part I).
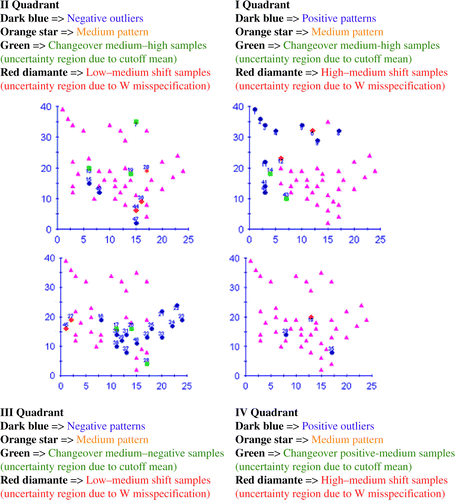