Figures & data
Table 1. Ground data samples over Australia for the year 2014.
Figure 1. Methodology overview. Methodology workflow showing the integrated analysis of MODIS-NDVI time-series data using QSMTs and ACCA.
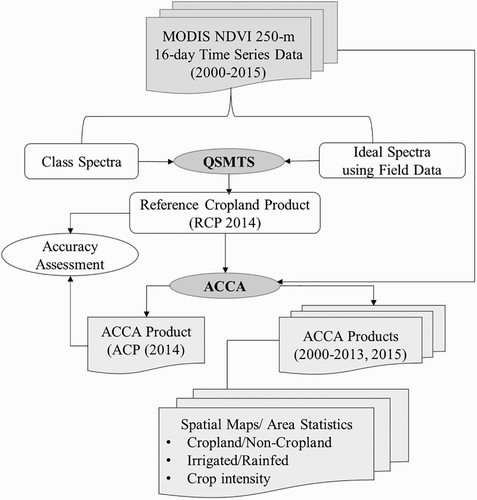
Figure 2. Three cropland masks of Australia and the development of ideal spectral signatures (ISSs) for each of these masks. Development of the three cropland masks is defined in Section 3.1. Using precise ground data and MODIS 250 m time-series data, unique and distinctly separable ISSs of the three masks were a total of 13 classes: (a) 4 for the rainfed mask, (b) 3 for the pasture mask, and (c) 6 for the irrigated-mask. The cropland fallow signature was common across. The total 13 classes can be combined to 6 unique classes by combining similar classes across masks.
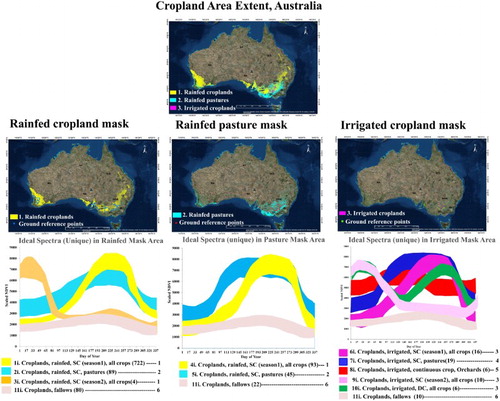
Figure 3. Ideal spectral library of crops. Illustration of few ground data samples collected throughout Australia and their t time-series MODIS 250 m NDVI ideal spectra profiles.
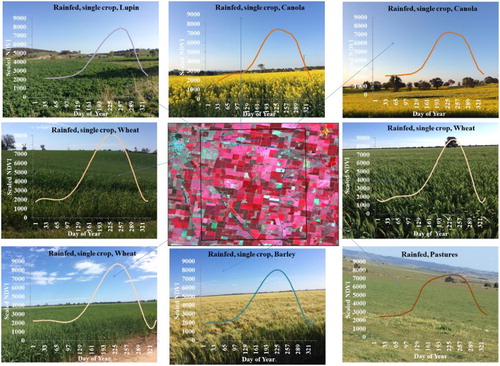
Figure 4. Illustration of SCS R2, a QSMT method, to group, match, assess, identify, and label classes. (a) Class spectra: group similar class spectra (e.g. class #’s 3,6,7, 9, … .,41, 44; total 27 similar classes from rainfed mask classification) obtained from ISOCLASS clustering classification, (b) ideal spectra: obtain an ideal spectra from the spectral library that closely matches with the grouped class spectra, (c) class spectra ((a)) matched with ideal spectra ((b)) using SCS R2 values (SCS R2 values varied between 0.83 and 0.994, with 26 of 27classes having SCS R-square values of 0.9 or greater), and (d) group of class spectra matched with ideal spectra: combine all similar class spectra into one group and match with ideal spectra which resulted in an SCS R2 value of 0.96 leading to labeling of the class spectral classes as similar name as ideal spectral label. So, the class name in this example is: croplands, rainfed, single crop (Season 1), all crops or mixed crops.
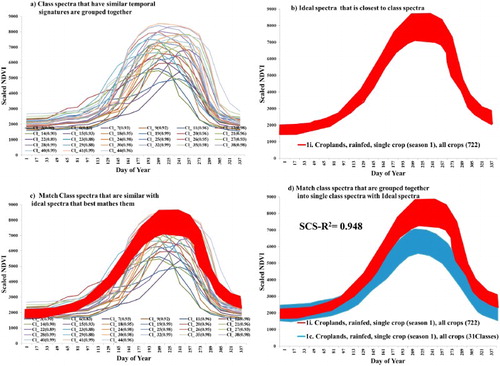
Table 2. SCS R2 values, a QSMT, matching class spectra with ideal spectra.
Figure 5. An automated cropland classification algorithm (ACCA) for Australia that can be used to compute six cropland classes as well as croplands versus non-croplands year-after-year automatically and accurately.
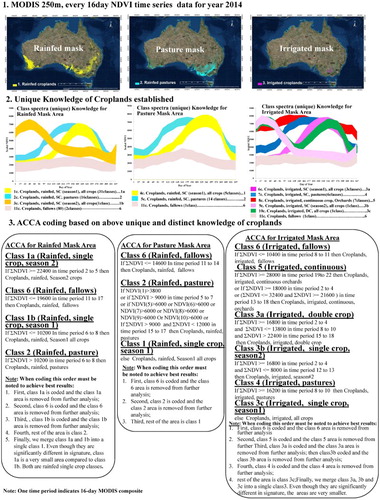
Figure 6. RCP of the year 2014 (RCP2014) for Australia at 250 m.
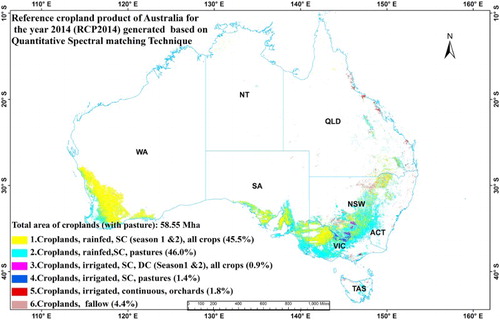
Table 3. Cropland areas of Australia for the year 2014 showing the net cropland areas (without considering cropland intensity) and annualized cropland areas (considering cropland intensity).
Table 4. Accuracy error matrix of the RCP of Australia for the year 2014 (RCP2014 on Y-axis) based on validation ground dataset #1 obtained during field visit (X-axis) also conducted during the year 2014.
Table 5. Accuracy Error matrix for the RCP for the year 2014 (RCP2014) conducted by external independent evaluation team based on validation dataset #2 obtained during field visit of year 2014.
Figure 7. ACCA algorithm derived cropland product for the year 2014 (ACP2014) for Australia at 250 m.
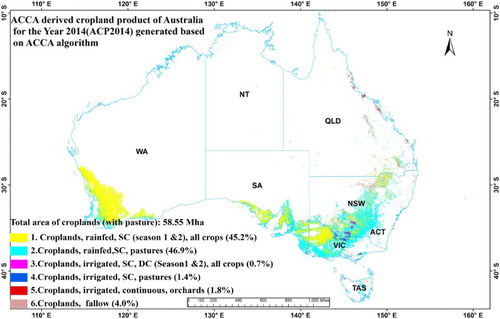
Table 6. Comparison of the statistics from RCP2014 with ACP2014.
Table 7. ACP2014 versus RCP2014 error/similarity matrix.
Table 8. ACP2014 versus GRIPC500 m (CitationSalmon et al. 2015) accuracy assessment.
Table 9. Comparison of Australia’s irrigated areas from different studies.
Figure 9. ACCA-derived cropland products for the past years (hind-cast) and future years (future-cast) for Australia. ACCA algorithm that was developed based on extensive ground data for the years 2014 was applied for the past years (2000–2013) and for a future year (2015). We have illustrated here ACCA-derived cropland products for 4 years: three past years (ACCA-derived cropland product for the year 2002 or ACP2002, ACP2004, and ACP2010) and for a future year (ACP2015). ACCA algorithm can be applied to develop similar products for the any future year.
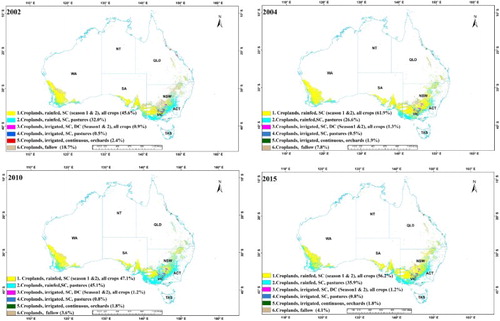
Figure 10. ACCA algorithm derived croplands (ACPs) for drought and non-drought years for Australia. Croplands versus cropland fallows computed using ACCA algorithm are shown for two: (a) worst drought years: 2002 and 2006 (ACP2000 and ACP2006), (b) normal years: 2003 and 2004 (ACP2003 and ACP2004), and (c) good years: 2000 and 2010 (ACP2000 and ACP2010).
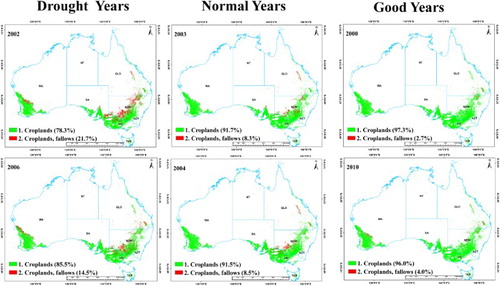
Figure 11. ACP2000 to ACP2015 derived cropland areas versus cropland fallow areas.
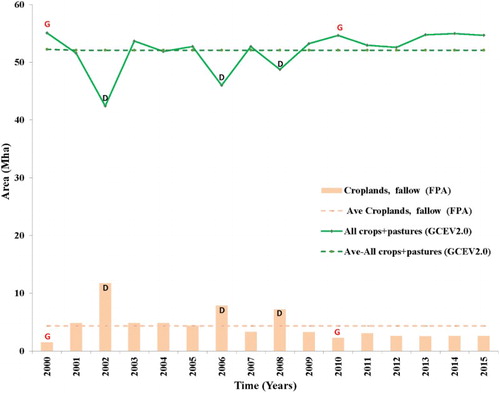
Figure 12. Cropland vigor during worst drought years versus the best above normal years. Mean MODIS 250 m NDVIs of Australia’s croplands during the: (a) three worst drought years of the century (2002, 2006, and 2008) versus (b) two very good years (2000 and 2010).
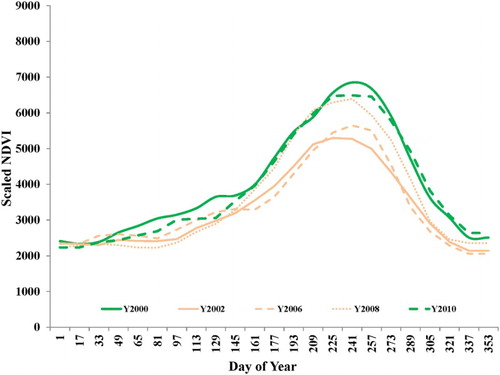
Figure 13. Cropland products of Australia. (a) Product 1: croplands versus non-croplands; (b) Product 2: irrigated versus rainfed; and (c) Product 3: cropping intensity: croplands with single, double, or triple cropping as well as cropland fallows.
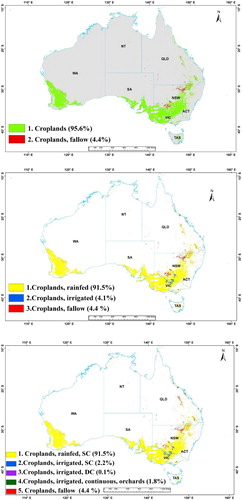