Figures & data
Figure 1. Location of the study region and distribution of sampling points. (a) Location of Xiangfu District in Henan Province; (b) Location of leaf area index sampling points; (c) Leaf area index, Global Positioning System, and soil moisture was measured at each sampling site.
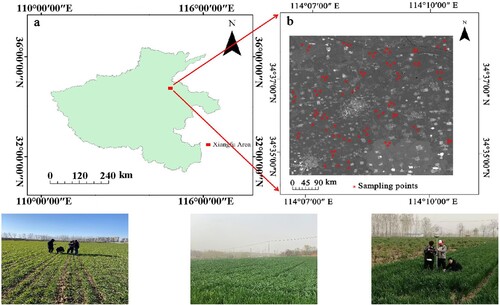
Table 1. Optical and microwave polarization decomposition vegetation index model.
Table 2. The range of all parameters in generating look up table
Table 3. Model accuracy index under different numbers of neurons in the hidden layer.
Table 4. Model accuracy index under different numbers of hidden layers.
Figure 3. The optical and microwave polarization decomposition vegetation index models. (a–e) are from the jointing, booting, heading, filling, and maturing stages, respectively.
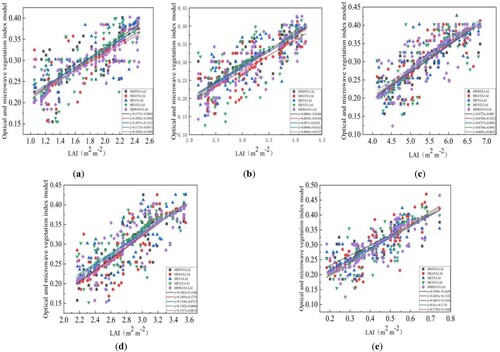
Table 5. Accuracy evaluation of the optical and microwave polarization decomposition vegetation index model.
Figure 4. Correlation analysis between the backscattering coefficient model and LAI. (a–e) are from the jointing, booting, heading, filling, and maturing stages, respectively.
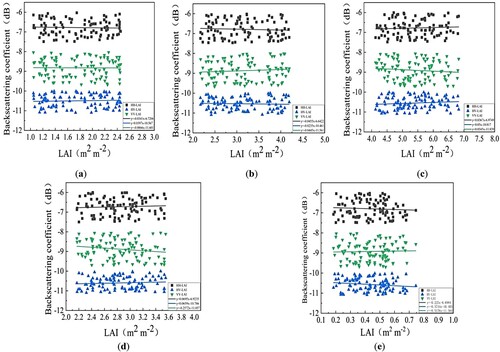
Table 6. Accuracy evaluation of the backscattering coefficient model.
Figure 5. Correlation analysis of MWCM and LAI. (a–e) are from the jointing, booting, heading, filling, and maturing stages, respectively.
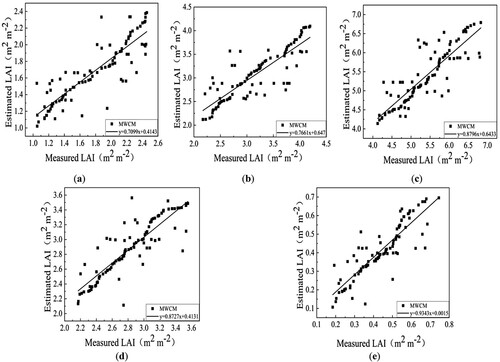
Table 7. Model parameters at HH, VV, and VH polarizations.
Table 8. MWCM accuracy evaluation.
Figure 6. Correlation analysis between BPNNM and GANNM models and LAI. (a–e) are from the jointing, booting, heading, filling, and maturing stages, respectively.
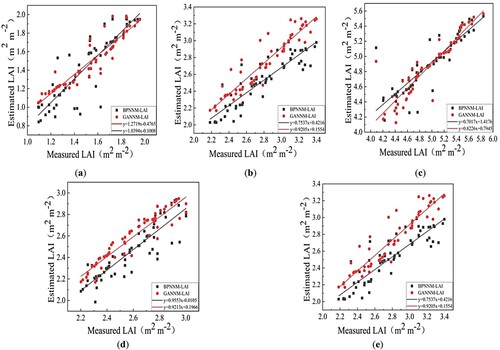
Table 9. Accuracy evaluation of the neural network model.
Figure 7. The GANNM model and LAI verification analysis. (a–e) are from the jointing, booting, heading, filling, and maturing stages, respectively.
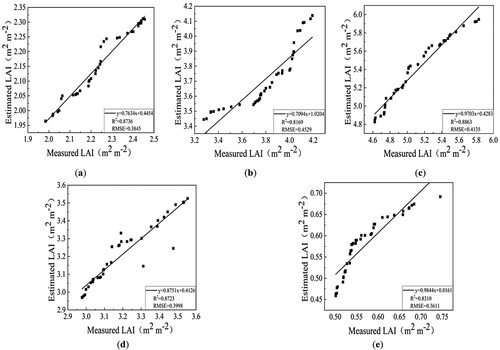
Data availability statement
The code used in this study are available by contacting the corresponding author.