Figures & data
Table 1. The G-Pisarenko tomographic spectrum estimation method.
Table 2. Parameters of the P-band F-SAR airborne SAR system.
Table 3. Baseline information of the used datasets from the Lope region.
Figure 2. Comparison of the different methods for with different SNRs: (a) SNR = 20 dB; (b) SNR = 5 dB.
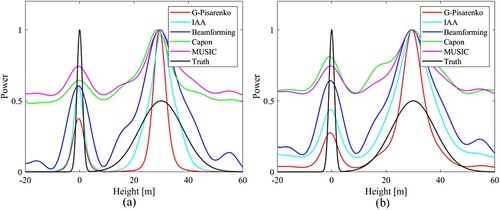
Figure 3. Comparison of the different methods for with different SNRs: (a) SNR = 20 dB; (b) SNR = 5 dB.
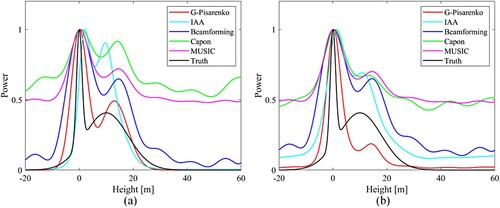
Figure 9. Tomograms of the HH (left column), HV (middle column), and VV (right column) channels on the selected range profile: (a), (f), and (k) Beamforming; (b), (g), and (l) Capon; (c), (h), and (m) MUSIC; (d), (i), and (n) IAA; (e), (j), and (o) G-Pisarenko. The black solid lines and white dotted lines denote the LiDAR DTM and CHM, respectively.
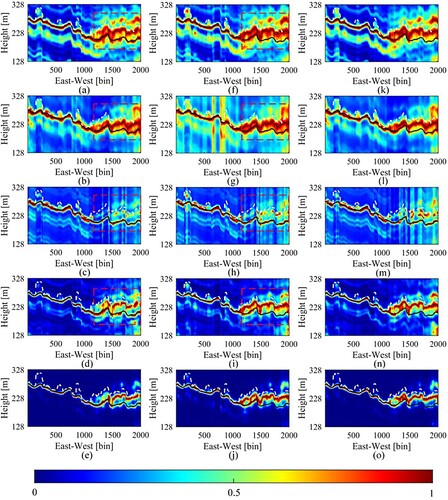
Figure 10. The estimated results obtained by the TomoSAR methods for the selected profile: (a) underlying topography, (b) forest canopy.
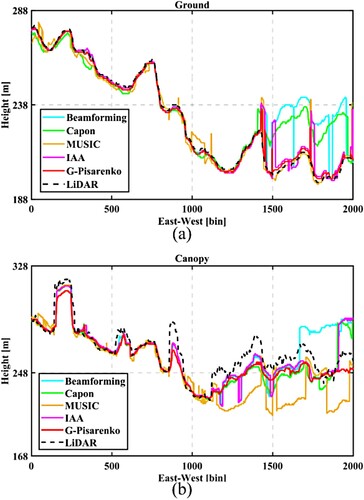
Table 4. Running times of the different methods for estimating the selected profile.
Figure 11. Estimated DTMs: (a) Beamforming, (b) Capon, (c) MUSIC, (d) IAA, (e) G-Pisarenko; referenced DTM: (f) LiDAR.
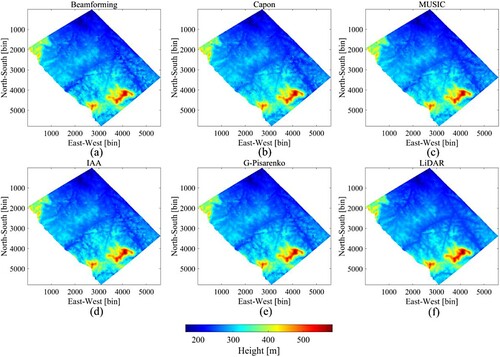
Figure 12. 2D joint distribution between the LiDAR DTM measurements and the estimations of the five methods for the selected range profile: (a) Beamforming, (b) Capon, (c) MUSIC, (d) IAA, (e) G-Pisarenko.
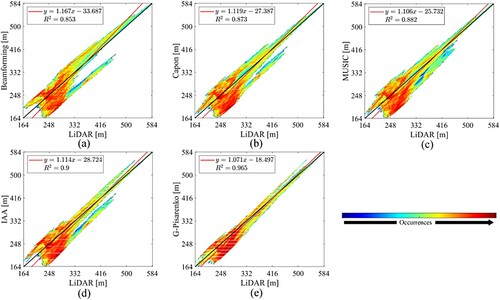
Table 5. The mean error and RMSE of the different methods for the underlying topography of the whole study area.
Figure 14. Estimated CHM: (a) Beamforming, (b) Capon, (c) MUSIC, (d) IAA, (e) G-Pisarenko; referenced CHM: (f) LiDAR.
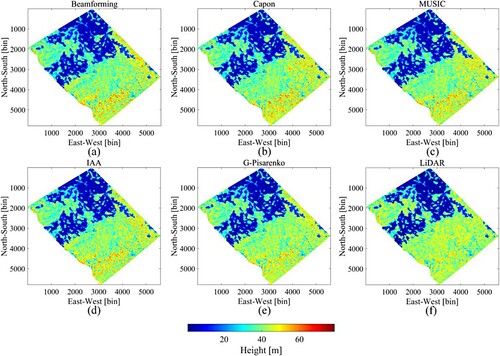
Table 6. The mean error and RMSE of the different methods for the forest heights of the whole study area.